SUPERCHARGE YOUR SEO Strategy & VISIBILITY! CONTACT US AND LET’S ACHIEVE EXCELLENCE TOGETHER in 2025!
Thank you for joining us for the new wave of SEO with Hyper-Intelligence 2.0 by ThatWare. This next-generation approach is changing the paradigm of search optimization. Prepare well for 2025 with 150 groundbreaking strategies designed to promote your brand ahead of the competition. As strong believers in optimizing websites with AI, we are going beyond the current routine of achieving hyper-intelligent search solutions.
Envision a scenario where search engine optimization is reactive to the given sequence of rules and predictive—changing within seconds with unmatched precision to keep your campaigns ahead of time. ThatWare’s HYPER-INTELLIGENCE 2.0 gives you a glimpse of an SEO strategy that is light years ahead of the current market. In this ultimate guide, we aim to unveil our 150 advanced Hyper-Intelligence SEO techniques developed to boost your digital presence, outrun your competitors, and prepare your brand for success in the long run.
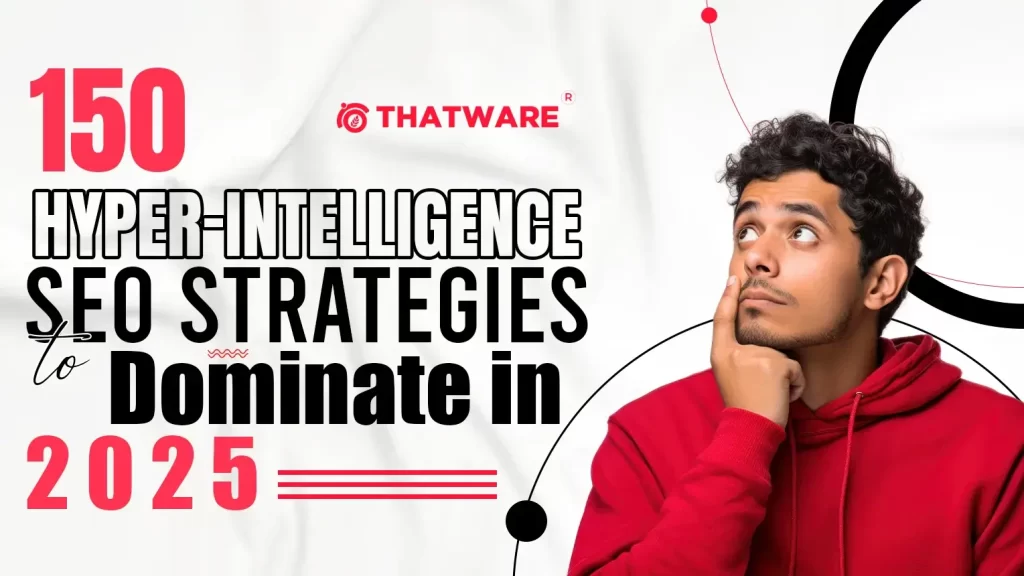
Here’s a sneak peek into what makes Hyper-Intelligence SEO truly transformative:
- A Game-Changer in Precision: It is time to stop relying on the traditional, all-inclusive SEO approach. By adopting Hyper-Intelligence 2.0 techniques, results can be highly segmented and targeted using deep learning algorithms and predictive analytics.
- Future-Proof Strategies: Instead of reacting to algorithm changes, we’re preparing you to stay a step ahead. Hyper-Intelligence 2.0 will position your brand with a dynamic, adaptable strategy, ensuring resilience against digital shifts.
- Beyond AI—Into Hyper-Intelligence: When typical AI SEO practices are still at the introductory level, we push the boundaries and make SEO much more cognitively effective and in harmony with one’s organizational objectives.
⭐️Exploring Hyper-Intelligence SEO: The Next Evolution in Digital Strategy
Today, the way businesses approach search engine optimisation (SEO) has transformed significantly. What began as a practice focused on keyword density and backlinks has now expanded to encompass content quality, user intent, and technical SEO. However, a new shift is emerging—one that promises to revolutionise SEO by integrating intelligent data processing, real-time analytics, and predictive insights. This innovation is known as “Hyper-Intelligence SEO,” an approach that leverages Hyper-Intelligence technology to deepen digital marketing strategies and create more dynamic, responsive optimisation methods.
Incorporating hyper-intelligence into SEO can unlock new levels of precision and effectiveness, driving impactful results and helping brands stay competitive in an increasingly digital-first world. But what exactly is hyper-intelligence, and how does it enhance SEO? In this article, we’ll explore what hyper-intelligence SEO entails, its role in digital marketing, the strategies it enables, and the advantages it offers to businesses.
⭐️What is Hyper-Intelligence?
Hyper-Intelligence is an advanced technological approach that combines Artificial Intelligence (AI), Machine Learning, and large-scale data processing to deliver highly refined insights in real-time. Unlike traditional intelligence systems, which may operate with limited data sources or require considerable time to analyze information, Hyper-Intelligence leverages cutting-edge algorithms and predictive analytics to analyze vast amounts of data almost instantaneously. It creates an interconnected web of insights that are highly adaptable, context-aware, and capable of making proactive decisions or recommendations.
The key concept behind Hyper-Intelligence is to go beyond simply collecting and analyzing data to provide actionable insights that can predict trends, identify patterns, and make strategic suggestions. For example, while traditional data intelligence might analyze past customer behavior to understand buying patterns, Hyper-Intelligence technology is capable of predicting future customer needs based on real-time data, emerging trends, and contextual understanding.
In a business context, Hyper-Intelligence can be applied across various functions, such as marketing, operations, customer service, and especially SEO. It aims to bring together fragmented data from multiple sources—social media activity, website engagement, customer feedback, and more—to create a unified, intelligent system that can interpret data in the context of overall business objectives.
⭐️How Hyper-Intelligence Differs from Traditional Intelligence
To understand what makes Hyper-Intelligence distinct, it’s helpful to compare it to traditional intelligence systems. Traditional business intelligence relies on historical data and operates largely on a reactive basis, analyzing past events to identify trends or opportunities. These systems are effective for reporting but lack the agility needed to make real-time decisions or adapt quickly to changes.
Hyper-Intelligence, on the other hand, incorporates real-time data analysis, predictive algorithms, and contextual insights. This allows it to function as a proactive tool rather than a reactive one. By constantly analyzing new data and recalibrating its insights, Hyper-Intelligence can make adjustments as market conditions or user behaviors shift. In digital marketing, for instance, this technology can detect shifts in consumer preferences and optimize campaigns within minutes, delivering highly relevant content or advertisements as user intent changes.
⭐️Components of Hyper-Intelligence Technology
Hyper-Intelligence is a convergence of several advanced technologies. Here are some core components:
- Artificial Intelligence (AI) and Machine Learning: At the heart of Hyper-Intelligence is AI, especially machine learning, which enables systems to “learn” from new data without needing reprogramming. Through machine learning, Hyper-Intelligence systems can recognize patterns, adapt to new data, and improve their accuracy over time.
- Natural Language Processing (NLP): NLP enables Hyper-Intelligence systems to understand and interpret human language, making them effective in analyzing text-based data, like customer reviews or social media comments. By understanding language context, NLP can derive sentiment, intent, and other qualitative insights, helping businesses understand the nuances of customer opinions and trends.
- Predictive Analytics: Predictive models play a crucial role in Hyper-Intelligence, allowing it to anticipate future trends, behaviors, or outcomes. By analyzing historical data alongside current information, predictive analytics can help businesses prepare for upcoming changes, whether they are shifts in customer behavior, emerging market trends, or potential operational disruptions.
- Big Data Processing: Hyper-Intelligence relies on big data technologies to process and analyze massive datasets in real-time. This enables organizations to draw from a wide range of data sources—social media feeds, IoT devices, web analytics, etc.—to create a holistic view of what’s happening and what is likely to happen.
- Real-Time Data Integration: Real-time data integration allows Hyper-Intelligence systems to capture and analyze data as it is generated. This component is vital for dynamic industries like SEO and digital marketing, where timely data can be the difference between capitalizing on a trend and missing it altogether.
⭐️The Purpose and Potential of Hyper-Intelligence
Hyper-Intelligence was developed to meet the growing demand for technology that can handle the vast, complex data of today’s digital landscape. Businesses need more than simple data insights—they need systems that can guide decisions, optimize strategies, and enhance efficiency. Hyper-Intelligence fills this gap by providing high-level insights that are timely, accurate, and actionable.
For example, in the context of SEO, Hyper-Intelligence can help brands understand not only what users are searching for but also why. By analyzing user behavior patterns, seasonal trends, and emerging topics, Hyper-Intelligence offers brands the ability to predict the types of content that will resonate with users before they even begin their searches. This proactive approach transforms SEO from a reactive practice into a forward-looking strategy, empowering businesses to stay ahead of their competition.
⭐️Understanding hyper-intelligence in the context of SEO
Hyper-intelligence is a term that describes advanced technology combining artificial intelligence (AI) with data processing capabilities far beyond conventional methods. By integrating data from various sources and interpreting complex user behaviours, yper-intelligence provides highly nuanced insights that help businesses make smarter, faster, and more proactive decisions.
Hyper-intelligence technology doesn’t simply analyse data; it synthesises information from diverse channels—such as social media, websites, and customer interactions—into a cohesive understanding of what drives user behavior. For SEO, this means going beyond optimising for search algorithms; it’s about crafting a digital experience that aligns seamlessly with user intent and behaviour in real-time.
This technology is not just theoretical. Major tech companies, including Google and Amazon, have leveraged forms of hyper-intelligence to refine their algorithms. Now, businesses of all sizes are beginning to harness this power to transform their SEO and marketing strategies.
⭐️The Role of Hyper-Intelligence in Digital Marketing
In the context of digital marketing, Hyper-Intelligence operates as a central hub that integrates insights from various touchpoints. Rather than relying on isolated data from individual channels, Hyper-Intelligence technology consolidates information across an entire digital ecosystem—everything from social media engagement to customer purchase histories—to form a complete picture of the audience.
Hyper-intelligence in digital marketing enables businesses to be far more agile, adjusting strategies on the fly based on data in real-time. Imagine a scenario where, during a product launch, Hyper-Intelligence can detect early feedback patterns and adjust messaging to enhance appeal or mitigate issues almost instantly. This kind of responsiveness is invaluable in today’s fast-paced market and allows brands to capture and retain audience attention more effectively.
For SEO, Hyper-Intelligence provides additional layers of insight by integrating data from organic search performance with other metrics, like user journey analysis and engagement rates. This holistic view allows marketers to tailor content not just to rank highly but to resonate deeply with users—improving both SEO results and overall customer satisfaction.
⭐️Hyper-Intelligence for SEO: Enhancing Strategies and Results
Hyper-Intelligence SEO leverages sophisticated algorithms that go beyond simple keyword matching. By analysing patterns in user search behaviour, content engagement, and click-through rates, it refines SEO strategies to align more closely with user intent. It can even help predict the types of content users might seek based on their past interactions, enabling brands to stay one step ahead in their content creation.
Hyper-Intelligence for SEO focuses on combining structured data, predictive analytics, and advanced natural language processing (NLP). These tools collectively allow brands to anticipate what users are likely to search for, the terms they’ll use, and the type of content they’ll engage with most. For example, by analysing NLP-driven insights, hyper-intelligence SEO can help identify emerging trends in user queries, allowing brands to create content that captures high traffic even before trends peak.
Additionally, by optimising on a predictive basis, Hyper-Intelligence SEO helps businesses maintain steady ranking positions rather than playing catch-up after trends emerge. This proactive approach gives brands an edge over competitors, especially in fast-evolving niches where timing is critical.
⭐️Hyper-intelligence strategies for boosting SEO performance
Integrating hyper-intelligence strategies into SEO involves more than just technological adjustments; it requires a strategic rethink of how SEO is approached. Below are some key hyper-intelligence strategies that enhance SEO performance:
- Audience Segmentation: Hyper-Intelligence enables highly detailed audience segmentation based on behaviour patterns and predictive analytics. Instead of broad categories, businesses can target specific user segments with precision, crafting content that speaks directly to their needs and preferences. This results in improved engagement and increased conversion rates.
- Predictive Analytics: Hyper-Intelligence uses predictive models to forecast user behaviours and preferences. This means SEO efforts can be directed towards content that is likely to gain traction based on anticipated trends rather than just current patterns. Predictive analytics allows brands to position themselves ahead of the competition by catering to future demands.
- Enhanced Personalisation: Tailoring content based on user-specific data, Hyper-Intelligence SEO enables a highly personalised experience for users. This includes personalised search results, dynamic content updates, and custom landing pages that adapt based on user interaction. The result is an experience that feels uniquely tailored to each visitor, driving both retention and loyalty.
These strategies create a powerful advantage by making SEO efforts more targeted, timely, and relevant. Rather than relying on historical data alone, hyper-intelligence allows brands to move with the market and make data-driven decisions in real time.
⭐️Benefits of Hyper-Intelligence in Business
The integration of hyper-intelligence SEO brings numerous benefits to businesses. Below are a few of the most impactful:
- Precision Targeting: By refining audience segmentation and predicting behaviour, businesses can achieve far more precise targeting. Hyper-inteligence in business means connecting with potential customers at the exact time they’re searching for relevant solutions.
- Increased Efficiency: Hyper-Intelligence marketing significantly improves the efficiency of campaigns. Instead of trial-and-error methods, Hyper-Intelligence uses data-driven insights to direct resources to the most promising strategies, maximising returns and minimising waste.
- Enhanced Decision-Making: With Hyper-Intelligence technology, businesses can make decisions faster and with greater confidence. Insights gained from integrated data can inform content creation, ad targeting, and product development, creating a cohesive approach that drives growth.
- Higher ROI: Hyper-Intelligence in digital marketing allows for a more effective allocation of resources, leading to higher returns on investment (ROI). By focusing on the most impactful strategies and aligning SEO with real-time insights, businesses can achieve measurable results faster.
- Improved Customer Satisfaction: With the ability to personalise and optimise user experiences, businesses can boost satisfaction levels. Hyper-intelligence technology enables a more responsive approach, addressing customer needs as they arise and fostering greater brand loyalty.
By embedding hyper-intelligence into their digital strategies, businesses not only improve their SEO but also achieve broader business goals more effectively.
⭐️Future Trends: The Evolution of Hyper-Intelligence SEO
Looking ahead, the future of hyper-intelligent SEO is poised to grow as new advancements emerge. Quantum computing, for instance, could exponentially increase processing speeds, allowing hyper-intelligent systems to analyse even larger datasets in real-time. This would make predictive SEO even more accurate and valuable.
Another area to watch is the integration of immersive technologies, such as augmented reality (AR) and virtual reality (VR), into hyper-intelligence strategies. As these technologies become more mainstream, they will likely reshape the digital experience, creating new SEO opportunities that cater to visually driven, interactive content.
Lastly, we can expect continued improvements in natural language processing (NLP), which will further refine Hyper-Intelligence’s ability to interpret complex queries and user intent. This means that search engines and websites alike will be better equipped to provide more accurate, personalized content to users, creating a better overall digital experience.
Learn how ThatWare’s Hyper-Intelligence SEO will change the digital marketing landscape in 2025 and beyond and give you an astounding edge in the clawing for the summit. We are not standing in motion with you but ahead of you.
⭐️RankBrain
RankBrain was the algorithm Google launched in 2015 that used machine learning to interpret the search data and the background of why people were looking rather than purely on actual word-based matches. It thus learned continuously and constantly from very large heaps of user data. It finds patterns between those collections and enables improving relevance for searching. Such relevance can now even arise in ambiguous and unknown types of queries. This advanced algorithm helps Google better interpret words and phrases in the context of understanding results that reflect user intent more closely than surface-keyword-based queries.
👉How ThatWare Enlists RankBrain for SEO Mastery
Application of RankBrain at ThatWare means digging deeper into user intent than keywords. Sophisticated AI-driven analysis lets ThatWare figure out what a user wants and why that user wants it. The intent-based keywords and semantic analysis that ThatWare develops result in content that can connect with a wider scope of user needs, resulting in better engagement and rankings. Unlike most of its competition, which is all shallow keyword optimization, ThatWare’s RankBrain strategies embrace several layers of user understanding, including mapping different types of user intent: informational, transactional, and navigational, and creating specific content pathways for each.
For instance, if a user searches for “best eco-friendly packaging for e-commerce,” ThatWare’s approach leverages RankBrain principles to produce content tailored to both informative aspects (explaining what eco-friendly packaging is) and transactional elements (providing product recommendations or links to eco-packaging solutions). This layered content improves rankings and ensures users find what they seek, making ThatWare’s SEO approach truly user-centric.
This subtle application of RankBrain ensures that ThatWare is ahead of the curve because its content is optimized for search engines and real user experiences. This edge keeps ThatWare ahead in the competitive SEO landscape.
⭐️BERT (Bidirectional Encoder Representations from Transformers)
BERT is a neural network-based NLP technique developed by Google in 2019 to enhance the accuracy of search results. Unlike typical models that linearly read text, BERT reads a search query from two directions, reading words about each other and their contexts in the sentence. This makes it possible to be sensitive to fine-grained language understanding, where a word may have different meanings based on the other words used with it. With this heightened contextual sense, BERT enables Google to deliver extremely relevant results, particularly in conversational and complex searches.
👉How ThatWare Uses BERT for SEO
ThatWare has recognized BERT’s revolution in search results, which have become more intuitive and user-centric. For SEO activities, ThatWare uses BERT to better understand user intent and develop more precise and contextually relevant content. In keyword research and content development, the team at ThatWare centers on placing keywords and developing content as closely as possible to the natural expression of needs in the search query. This approach captures pages well for complex, conversational queries where context is essential, which simple SEO may miss.
For example, if a real estate industry client wants to rank for “how to buy a house with no money down,” ThatWare’s content strategy goes far beyond keyword optimization. They write articles on financing the house purchase, providing in-depth, context-aware content directly addressing users’ needs. This approach fits well with BERT’s contextual understanding, and it’s much more likely that ThatWare’s clients will appear in top search results for queries requiring in-depth explanations.
⭐️Neural Matching
This is the breakthrough technology that Google uses to understand the intent of searching and recognize relationships between words and concepts within a search query. Google will, therefore, be able to identify synonyms and relevant contexts with neural matching, making the results very effective in responding to ambiguous or complex queries. For instance, if a person searches for “Why is my TV screen blurry?” neural matching would help Google know that the user is looking for troubleshooting advice on display quality, not screens or TV features.
ThatWare utilizes neural matching to enhance SEO techniques, making it structure content based on questions that users want. The approach does not just target keyword frequency; instead, it looks into the intent and context for each search term, allowing pages to hit the users’ concerns directly. That will make content much more findable even if the keywords differ, opening the scope up to more searches.
For example, based on the same dental patient that wants to rank on the query “tooth pain at night,” ThatWare would apply neural matching in structuring content for why patients will probably have a worse toothache at night, its probable cause, and some successful remedy. Now, given such intent-driven content structured this way, even as a user puts out different searches such as “teeth hurt when I sleep” or “night toothache,” the content will be aptly and possibly in better search results.
ThatWare stands out from the competition that uses keyword-stuffing methods, far from understanding search intent. ThatWare’s strategy maximizes content alignment with what users seek, enhancing engagement and driving higher-quality traffic, ultimately improving client conversion rates.
⭐️MUM (Multitask Unified Model)
MUM is one of the most advanced AI-driven frameworks that Google has built, and it can process very complicated search queries. Besides search models, this can process information in languages and modalities like text, images, and videos, making it very powerful for nuanced, multifaceted searches. For example, suppose a user is looking for hiking in another country. In that case, MUM can extract the most relevant insights from other language sources, guaranteeing that users receive much richer and more diverse results. Furthermore, MUM utilizes multimodal capabilities, which means it can interpret text and images; this function opens up to very personalized and contextual results depending on the various types of input.
ThatWare exploits MUM by designing its SEO practice to fit closely with this model’s capabilities. For instance, if the focus is on international markets, ThatWare has a multilingual SEO strategy; it creates content and optimizes websites for several languages. This way, its client’s content is always connected to a global audience. It also prefers diversification in the content strategy through images, video, and text so that search engines pick up on multiple cues and the content is better visible across different formats.
For instance, the eCommerce client may focus on outdoor gear. Thus, for a hiking gear campaign, ThatWare would develop visual content like infographics about trails or videos explaining equipment usage along with text-based guides. The method takes advantage of MUM’s multimodal processing capability, which allows Google to identify content irrespective of its form or language. This, therefore, fortifies the brand’s footprint in terms of SEO globally. Such alignment that differentiates MUM from the competition is a richer, more versatile approach to SEO that rings deep in a multifaceted digital landscape.
⭐️Passage Ranking
Passage Ranking is an innovation from Google that was published in 2020: the ranking of specific passages from a webpage rather than the page itself. This approach is very important for long-form content as the most critical insights may reside deep within the text, allowing Google to understand the language better. Passage Ranking lets Google separate answers or any other details from the page and then rank the passages by the query. For instance, in an extended blog on SEO, a section titled “the benefits of keyword research” could rank separately for users who type directly about this benefit. In doing so, Google aims to maximize relevance and user satisfaction with search results.
At ThatWare, Passage Ranking is one of the core strategies for providing results that meet the user’s intent. Our team carefully structures content so that Google’s algorithms can easily pick up the most relevant passages that will likely answer specific queries. ThatWare uses effective subheadings, concise paragraphs, and strategic keyword placement to enhance Google’s ability to pick out valuable content snippets. Our AI-based SEO tool can also analyze user search patterns to identify which passages may gain traction, optimizing content for Passage Ranking specifically.
For example, if a client website has a guide on “eCommerce SEO,” ThatWare structures sections to respond to possible micro-queries, such as “How to apply SEO to product pages” or “How to optimize meta tags for eCommerce.” Each piece is structured and optimized with keyword relevance, clarity, and contextual depth. ThatWare’s SEO practice, therefore, improves the visibility of a passage, making it information-rich, user-centric content. Their competitors will likely look past it, maximizing page authority and engagement.
⭐️Entity Understanding
Modern SEO uses entity understanding: It no longer only has keyword matching but can contextualize and recognize entities, people, places, events, or items within a search query; through these, search engines start interpreting and delivering results beyond superficial understanding, identifying connection patterns between concepts. It may seem quite simple, using a query like “Tesla achievements” to trigger everything more than just the written word “Tesla.” Instead, the algorithm interprets Tesla as an entity related to Elon Musk, electric vehicles, and sustainable energy innovations.
ThatWare stands out for its use of advanced entity recognition systems in its SEO strategy, which provides a deeper understanding of search queries and more targeted content. For example, if the site of a client is targeting “Artisanal Coffee Shops in Brooklyn,” the team at ThatWare will dig into understanding “Brooklyn” not only as a keyword but also as an entity associated with particular types of consumers, local culture, and trending coffee experiences. This semantic understanding allows them to create content and meta-data that reflect coffee and the culture surrounding Brooklyn’s artisanal cafes.
ThatWare implements entity understanding using an entity-based content framework that interlinks related entities across a client’s site. In other words, they encompass clusters around each single keyword instead of aiming to target a single keyword. For example, a personal injury attorney can use accident claims via the law firm, legal aid, and testimonials from clients to provide interlinking content. ThatWare applies NLP and machine learning algorithms to parse data and recognize such entities automatically; thus, their SEO efforts are both precise and scalable.
In essence, ThatWare’s approach to entity understanding puts SEO in line with the way people search today—dynamic, contextual, and competitive. By focusing on entities, they can optimize for both search engines and the intent behind human search queries.
⭐️Content Freshness Algorithm
The Content Freshness Algorithm is one of the important elements of modern SEO techniques. It favors the newest and most relevant content in search results, which means users get updated information while searching online. This algorithm is based on the dynamic nature of information on the web, where timely updates may greatly affect the authority and relevance of a website. Consequently, websites that update their information more frequently or post articles often are ranked higher during a search. This focus on freshness can be particularly beneficial in sectors that depend on news and current information, for example, news, tech, and health.
At ThatWare, content freshness is achieved by building a strong content calendar with frequent updates and new publications. The trends and user interests help the team know where the topics are regarding timely updates or new insights. For example, if the regulations governing digital marketing have a radical change, ThatWare would immediately produce and distribute a blog post explaining the change, the implications, and strategies to adapt. This proactive method not only delivers information and value to the audience but, through a search engine, also indicates that the website is an important and relevant source of information.
Second, ThatWare uses tools and analytics to refresh and revisit older posts. For instance, if a blog post on the best practices for SEO has started gaining traction but has been more than a year old, the team would update it with the most recent strategies, data, and case studies. Revisiting and rejuvenating existing content not only boosts the post’s visibility but enhances the entire website’s authority. ThatWare differentiates itself from competitors because its content will always remain up-to-date concerning the latest trends and updates, ensuring its clients obtain superior search engine visibility and engagement.
⭐️Synonym Matching
The synonym-matching form of search engine optimization enhances the site’s interpretation of user intent. Unlike most keyword matches, this technique identifies synonyms and replaces them to perfectly match the query searches. Today, users may pose their questions in a wide array of ways within this new search landscape. This is how the SEO practitioner’s content stays more relevant to a larger scope of searches and captures traffic they would otherwise not get at all.
👉ThatWare’s Implementation of Synonym Matching
Using more advanced NLP techniques in the SEO methods, ThatWare implements synonym matching. Given the algorithms that analyze and identify the context in words, ThatWare can automatically match synonymous terms with primary keywords. In the case where the user has entered “affordable housing,” ThatWare’s method would allow a website to also appear on related terms such as “budget-friendly homes” or “low-cost residences.”
This strategy maximizes search possibilities and improves the user’s experience in getting relevant results for their query. Synonym matching within a keyword strategy allows ThatWare to generate content that engages users based on different linguistics and increases its likelihood of ranking higher for diverse variations of search queries.
👉Differentiation from Competitors
ThatWare will differ with its ability to leverage the strength of the context and not keyword density. Most SEO companies are concerned with keyword optimization on certain terms; therefore, they must acquire variations, which leaves holes in organic traffic. For instance, while a competitor may be optimizing over the term “digital marketing,” ThatWare may optimize over synonyms of “online advertising,” “internet marketing,” and “web promotion” to become more exposed.
In addition, ThatWare continues to update its keyword strategy by adding new synonyms based on user behavior and trends in use. This implies that their SEO practice follows changing language, hence managing newly emerging search patterns even ahead of the competition. That’s how ThatWare shows more knowledge of language and a deeper understanding of context when turning SEO from a purely linear task into a real-time, user-centric strategy.
⭐️Knowledge Graphs
Knowledge graphs are powerful tools used in SEO to create a structured representation of interconnected information about entities such as people, places, and things. Knowledge graphs help search engines deliver more precise answers to user queries by organizing facts to highlight relationships and context. This shift towards providing direct answers rather than just a list of links enhances the user experience and aligns with the evolving landscape of search engine optimization.
ThatWare leverages knowledge graphs in its SEO practices to distinguish itself from competitors. By integrating structured data markup, they ensure that their content is understood by search engines, which increases the likelihood of appearing in rich snippets or knowledge panels. For instance, when a user searches for a specific brand or service, ThatWare’s implementation of knowledge graphs enables search engines to pull relevant information directly from their website, such as location, contact details, and service offerings.
An example of this can be seen with a local business like a dentist’s office. When optimized with a knowledge graph, a search for “dentist near me” might show a knowledge panel featuring the dental practice, complete with ratings, operating hours, and a brief description. ThatWare utilizes Schema.org markup to enhance its clients’ online presence, ensuring that essential information is easily accessible and visible in search results.
Additionally, ThatWare’s commitment to continuous updates and enhancements of its knowledge graph structure ensures that the information remains accurate and relevant. This proactive approach helps maintain high visibility in search results and fosters trust with potential customers seeking reliable and straightforward answers to their queries. Using knowledge graphs effectively, ThatWare demonstrates a sophisticated understanding of SEO that sets them apart in a competitive market.
⭐️Core Web Vitals
Core Web Vitals are specific factors that Google considers essential for delivering a high-quality user experience on the web. These metrics focus on three primary aspects of page performance: loading, interactivity, and visual stability. The three main components of Core Web Vitals are Largest Contentful Paint (LCP), First Input Delay (FID), and Cumulative Layout Shift (CLS). Each of these metrics plays a crucial role in how users perceive and interact with a webpage.
- Largest Contentful Paint (LCP) measures loading performance and reflects the time it takes for the largest visible content element (like an image or block of text) to load completely. An optimal LCP score is under 2.5 seconds. If a website takes longer to load, users will likely abandon it, leading to higher bounce rates.
- First Input Delay (FID) assesses interactivity and measures the time it takes for a page to respond to a user’s first interaction, such as clicking a button or a link. A good FID score is less than 100 milliseconds, ensuring users feel engaged and recognize their actions promptly.
- Cumulative Layout Shift (CLS) gauges visual stability by quantifying unexpected layout shifts that can occur as page loads. A CLS score of less than 0.1 indicates a stable layout, which helps prevent users from accidentally clicking on the wrong element due to shifting content.
At ThatWare, implementing Core Web Vitals is fundamental to their SEO strategy. They conduct thorough audits of client websites to identify areas needing improvement. For instance, they optimize image sizes and leverage lazy loading techniques to enhance LCP. To improve FID, they employ advanced JavaScript optimization strategies to reduce load times, ensuring that user interactions are swift and responsive. Additionally, ThatWare minimizes layout shifts by using proper size attributes for images and videos, thus achieving a favorable CLS score.
By prioritizing Core Web Vitals, ThatWare boosts its clients’ search engine rankings and enhances user satisfaction, setting them apart from competitors who may not fully grasp the significance of these metrics in SEO. This commitment to user experience has increased client engagement and conversion rates, demonstrating the effectiveness of integrating Core Web Vitals into their SEO practices.
⭐️Query Contextualization
Query contextualization refers to interpreting the context behind a search query based on a user’s previous searches and behavior. It enables search engines to deliver more relevant results tailored to the user’s intent rather than just matching keywords. By analyzing factors such as location, time, device used, and previous interactions, search engines can discern what a user is looking for, even if their search terms are vague or ambiguous. For example, if a user frequently searches for “best coffee shops” and then queries “near me,” the search engine can infer that the user is seeking nearby coffee shops based on their prior interest.
👉How ThatWare Implements Query Contextualization
At ThatWare, query contextualization is a cornerstone of their SEO strategy, setting them apart from competitors. They leverage advanced machine learning algorithms and user behavior analytics to fine-tune their understanding of user intent. By tracking how users interact with their websites and analyzing the patterns of their searches, ThatWare can create personalized experiences for visitors.
For instance, if a user consistently visits pages related to digital marketing strategies and later searches for “SEO tips,” ThatWare’s optimization strategies prioritize content that aligns with digital marketing, providing tailored advice that resonates with the user’s interests. This nuanced approach improves user satisfaction and enhances dwell time and engagement, which are critical factors for SEO ranking.
👉Example in Practice
A practical example of this implementation can be seen in a recent campaign for a local restaurant client. By analyzing previous search behaviors and seasonal trends, ThatWare optimized the restaurant’s website to highlight relevant menu items and promotions during specific times of the year, such as showcasing outdoor seating in spring. This level of contextual awareness boosted traffic and increased conversion rates, demonstrating the effectiveness of query contextualization in action. By focusing on the broader context of user queries, ThatWare ensures that its SEO practices drive traffic and foster meaningful user engagement and conversions.
⭐️Context-Aware Spelling Correction
Context-aware spelling correction is a sophisticated approach that goes beyond traditional spell checkers by considering the broader context of a query rather than merely correcting individual words. This method uses machine learning and natural language processing (NLP) algorithms to understand the intent behind a search, leading to more accurate corrections that align with user intent.
For instance, if a user types “best place to eat,” a traditional spell checker might correct “place” to “place” without understanding the context of the query. However, a context-aware system can analyze the surrounding words and correct them to “best place to eat,” ensuring the search engine delivers more relevant results. This nuanced understanding of language helps minimize user frustration and improve the overall search experience.
ThatWare implements context-aware spelling correction in its SEO practices by utilizing advanced algorithms that analyze user queries comprehensively. They focus on understanding the semantic meaning behind a search, allowing their content to be optimized not just for keyword accuracy but also for context relevance. This strategy particularly benefits long-tail keywords and phrases containing misspellings or informal language.
For example, if a user searches for “how to bake a cake,” ThatWare’s systems would recognize the intent behind the query and optimize their content for “how to bake a cake,” ensuring that their articles are not only accurate in spelling but also relevant to the user’s actual intent. This sets ThatWare apart from competitors who may rely solely on keyword matching without considering context, resulting in less effective SEO outcomes. By focusing on context-aware spelling correction, ThatWare enhances user engagement and satisfaction, ultimately driving more organic traffic to their client’s websites.
⭐️Sentiment Analysis in Search
Sentiment analysis is crucial in understanding the emotional tone behind search queries. This technique utilizes natural language processing (NLP) to gauge whether a query’s sentiment is positive, negative, or neutral. By interpreting these emotions, search engines can provide more tailored and relevant results that align with the user’s intent. For instance, a user searching for “best pizza near me” likely expresses a positive sentiment, while someone typing “worst pizza experiences” indicates a negative sentiment.
ThatWare effectively integrates sentiment analysis into its SEO practices by prioritizing content that resonates emotionally with target audiences. Leveraging sophisticated algorithms and machine learning models, ThatWare analyzes trending topics and keywords in their clients’ industries. This allows them to create content that addresses users’ informational needs and aligns with their emotional states. For example, suppose a trend shows a surge in negative sentiment around a specific product or service. In that case, ThatWare can swiftly adjust its content strategy to address those concerns directly, providing solutions or alternative recommendations.
Additionally, ThatWare employs sentiment analysis tools to monitor brand perception in real-time. By analyzing user reviews, social media mentions, and feedback, they can identify areas for improvement or capitalize on positive sentiment to enhance brand reputation. For instance, if positive sentiment is detected around a new feature of a software product, ThatWare might implement this feedback into promotional content or social media campaigns, emphasizing user satisfaction and trust.
Overall, the unique application of sentiment analysis in ThatWare’s SEO strategies distinguishes it from competitors and fosters a deeper connection between brands and their audiences, leading to improved engagement and conversion rates.
⭐️User Intent Classification
User intent classification is a crucial aspect of modern SEO strategies, as it involves understanding the underlying purpose behind a user’s search query. By categorizing these queries into three primary types—transactional, navigational, and informational—businesses can better tailor their content and optimization strategies to meet user needs.
- Transactional Intent: Queries with transactional intent indicate that users want to purchase or complete a specific action. For example, a user searching for “buy running shoes online” is ready to engage in a transaction. ThatWare leverages this insight by optimizing product pages with clear calls to action (CTAs) and relevant keywords. By ensuring that product descriptions are rich with details about features and benefits, they guide users toward conversion. An example of this could be an e-commerce site incorporating user reviews, ratings, and comparison features to enhance purchasing decisions.
- Navigational Intent: Users with navigational intent are searching for a specific website or page. For instance, someone typing “Facebook login” wants to access their account directly. ThatWare distinguishes itself by ensuring its clients have strong, well-structured internal linking systems that enhance user navigation. This can include creating a sitemap and optimizing the site’s navigation bar to allow easy access to important pages. For instance, a local restaurant might optimize its Google My Business listing to ensure users can find its menu and location quickly.
- Informational Intent: Queries with informational intent aim to find answers or acquire knowledge. For example, a user searching for “how to tie a tie” is looking for instructions rather than products. ThatWare addresses this by creating high-quality, informative blog posts that provide valuable insights. They utilize long-tail keywords that match users’ questions, ensuring the content is relevant and authoritative. For instance, a financial services website might produce articles on “how to save for retirement,” addressing common queries while establishing expertise in the field.
By classifying user intent accurately and optimizing content accordingly, ThatWare enhances the overall user experience, improves search rankings, and drives targeted traffic to clients’ websites. This strategic approach separates them from competitors and positions their clients for sustained online success.
⭐️Image Ranking Algorithms (e.g., Vision AI)
In the evolving landscape of search engine optimization (SEO), image ranking algorithms have emerged as a significant factor in enhancing the visibility of visual content. These algorithms, particularly those driven by technologies like Vision AI, utilize advanced image recognition techniques to interpret and analyze images more effectively. This capability allows search engines to deliver more relevant results for visual queries, transforming how users discover and interact with visual content online.
Vision AI analyzes various attributes of an image, including its content, colors, shapes, and even the context in which it appears. By leveraging machine learning, it can categorize images, identify objects, and even understand emotions conveyed through visuals. For instance, if a user searches for “blue ocean waves,” a search engine utilizing Vision AI will prioritize images that accurately depict this theme, enhancing the user experience through relevant visual representation.
ThatWare implements image ranking algorithms by optimizing images on their clients’ websites with strategic use of alt text, descriptive file names, and schema markup. This not only helps search engines understand the content of the images but also improves accessibility for users. By ensuring that images are relevant and optimized for search queries, ThatWare enhances the likelihood of appearing in image search results, distinguishing itself from competitors who may neglect the importance of visual content.
An example of this implementation can be seen in e-commerce websites that utilize rich images to showcase products. By optimizing these images with keywords related to the product and employing high-quality visuals, ThatWare helps clients rank better in image searches. This drives traffic and boosts conversion rates as users are more likely to engage with visually appealing and well-optimized images, setting ThatWare apart in a competitive SEO landscape.
By focusing on image ranking algorithms, ThatWare embraces the future of SEO and equips its clients with a competitive edge in attracting and retaining audience attention through superior visual content management.
⭐️Conversational Search Models
In short, conversational search models revolutionize how users interact with the search engines by allowing multiple-turn conversations within the results. As opposed to conventional searching methods that, in most cases, require one word to start a new search every time, conversational models allow for a much more natural and intuitive dialogue exchange. Advances in natural language processing help such models interpret contextual information as well as understand the intent of the user at the turn of the question. It will then be possible for users to provide their follow-up questions and therefore receive relevant responses to those of their questions. Besides this, the search activity will feel like a normal dialogue. Thus, by this, the chances to find the needed information go up without needing repetitive searching queries.
At ThatWare, we make our search models conversational by working robust NLP algorithms directly within our search engine optimization approaches. By analyzing user interactions, we gain a kind of insight into what makes certain query types successful. Hence, we optimize the contents of our clients’ sites to rank better in multi round interactions. We also take care about the rich snippet and the structured data. This also enhances the possibility of better visibility in conversational search. Our focus here is all about making it very seamless by guiding the users through all their questions. By focusing on user engagement and user satisfaction, we help businesses find a competitive edge in the fast-changing digital world.
⭐️Contextual Answer Retrieval
The process of using contextual answer retrieval is done such that even in instances of long forms and extensive information content, a search engine will be able to find an appropriate answer. In this manner, the usage of complicated algorithms that focus on relating contexts to given queries happens for the purposes of producing appropriate information based on extracted relationships between the words as well as how to use context-based language appropriately. This method cuts down on time spent seeking irrelevant information and raises a higher possibility of finding relevant answers sooner.
At ThatWare, we get contextual answer retrieval through robust content analysis and a range of optimization techniques. The entire team of professionals here conducts keyword research to determine the most common questions users usually ask. We make sure that relevant answers are easily available within the content we create. We also give importance to structuring information, using clear headings, bullet points, and short paragraphs to enhance readability. By optimizing the content for users and search engines, we improve the clients’ chances of attaining featured snippets and zero-position rankings significantly. It means that in addition to attracting more organic traffic, it also places the content at the top as the go-to source for a specific piece of information for users.
⭐️Hyper-Personalization Algorithms
Actually, hyper-personalization refers to taking personalization another step higher by tailoring search results based on their history of searches, what’s liked or disliked, as well as demographics. Actually, it understands that different people have different tastes or interests, and it desires to bring about a meaningful sense of a search experience from giving the kind of content and material of interest to various interested individuals. Data analytics coupled with machine learning allows search engines to curate results on users so that they receive relevant answers to their inquiry, and thus, increasing the chances of engagement through conversions.
At ThatWare, we leverage hyper-personalization algorithms by analyzing large amounts of user data to create detailed profiles that inform our content recommendations. We use these insights to tailor the content on websites, marketing messages, and even user experiences. Additionally, we continuously monitor how users interact with our content, refining and adapting our strategies in real time. This agile approach ensures that businesses stay in tune with changing user preferences, enhancing customer loyalty while fostering long-term growth. By enabling brands to connect with their audiences in the most hyper-personalized ways possible, we help build meaningful relationships that ultimately augment brand value.
⭐️Video Search and Ranking (e.g., AI for Video Context)
Search rankings can tap into the power of AI, effectively extracting, analyzing, and ranking content so it captures context together with sentiments. With every upswing of video consumption statistics, getting a video more seen, thus increasing consumer interaction and engagement, implies optimisation in search, specifically across the different web or other digital media portals by correlating metadata/visuol/audio details so they correspond to various possible searches. These capabilities enable search engines to differentiate between sentiments and context inside videos by providing users with more accurate and satisfactory outcomes.
ThatWare’s services in video search and ranking optimize the content for search. We make sure videos are followed by necessary metadata such as title descriptions, tags, and catchy thumbnails. With the best video content that reaches the right group of people, we strictly follow industry best practices starting from SEO to get videos ranked better. We also include transcripts in the video to make them more accessible, keeping in check search engine optimization. Captions take our video indexability further, thereby maximizing the reach of our video on the search engines.
Our all-rounded approach ensures video rankings are strong while leaving business open to the real possibility of tapping into full video marketing potential. This tactic activates consumer engagement and further drives traffic to our clients’ websites. Through best practice approaches in video search and ranking, we help capture the attention of brands as their digital landscapes become ever more visual and drive growth through increased visibility.
⭐️E-A-T (Expertise, Authoritativeness, Trustworthiness)
It represents Expertise, Authoritativeness, and Trustworthiness: the measure that search engines use when evaluating the quality of different contents. These days misinformation is rampant and, due to this factor, quality content should only be published for users and not fallacies. So E-A-T emphasizes the presence of premium content created by knowledgeable content authors, and established authors and sources at websites sites. This improves the search experience by providing content that meets the best expectations of users for truthfulness and reliability by focusing on E-A-T.
At ThatWare, we incorporate E-A-T (Expertise, Authoritativeness, Trustworthiness) into our SEO strategies because it emphasizes the quality of content and the credibility of authors. We create informationally rich content written by knowledgeable professionals who are experts in their respective fields. This not only enhances the quality of the information provided but also establishes authority in the topic at hand.
Furthermore, we ensure our clients maintain a strong online presence by acquiring high-quality backlinks from authentic sources, attracting users in a constructive manner. We also emphasize the importance of displaying authors’ credentials and organizational values, which play a significant role in increasing user trust. By focusing on E-A-T, we help businesses build a credible presence in the market, improve their rankings in search results, and foster greater trust among users. This comprehensive approach positions our clients for long-term success in an increasingly competitive digital landscape.
⭐️Voice Search Optimization
Voice search optimization is the most important technique in the new digital age, considering the fact that most people are using voice-activated gadgets to find information. This approach is based on the peculiarity of the spoken language and how it is understood in terms of tone, intonation, and phrases used in a conversation. Since voice searches are usually more natural and conversational, content needs to be created in a manner that directly answers the actual spoken questions. In embracing voice search optimization, companies not only optimize but rather expand and become more effective for the increased volume of users searching via voice.
At ThatWare, we understand the importance of voice search optimization and put into use cutting-edge methods to help businesses remain afloat in this dynamic game. Our team conducts broad keyword research; we recognize the most popular voice searches related to our clients’ industries. We then create high-quality, engaging content that answers the questions directly. We also apply structured data markup to help search engines better understand and index voice-friendly content. We focus on natural language processing and user intent to ensure that our clients’ websites are voice search optimized, thereby giving them a better chance of being found in voice search. This forward-thinking approach drives traffic and improves user experience, hence making our clients the authorities in their fields. By using ThatWare, businesses will successfully navigate the future of search to reach new audiences.
⭐️Quantum Computing Models for Search
The new quantum computing models are said to change the present information processing way of the search engine, making them faster with a more accurate result. The computers can process a much larger amount of calculation as compared to those using traditional computers that only employ the binary process. This potential technology would help solve those problems considered unachievable by modern computers, speeding up the data processing as well as producing a very accurate search result. As the search landscape keeps shifting, quantum power will reflect a lot of new changes in people’s access to information and communication with their peers.
We at ThatWare are pioneering this technological advancement to be well-equipped for the future of search with proactive moves for quantum computing. Our research and development team tracks new development within quantum technology and helps include it in our approach. We are embracing leading-edge methodologies to prepare businesses for leveraging the advantage of quantum computing when the quantum benefit finally gains popularity. Our goal is optimizing algorithms and enhancing data processing methods as related to quantum model capabilities. Strategic foresight benefits both the search performance of our clients currently but positions them for dominance in their fields of endeavor. Business enterprises can now break into the quantum ecosystem and stay at the forefront of this increasingly complex digital space through the partnership with ThatWare.
⭐️Knowledge-Based Trust Algorithm
Knowledge-Based Trust Algorithm is one of the fundamental constituents of modern search engines, which evaluate the trustworthiness of facts and data in the content. In the world where the pace of spreading misinformation through the internet has been growing so rapidly, the need for trusting information online has never been higher. This algorithm calculates the trustworthiness of content based on different factors such as the credibility of the source, reliability of the information, and context. Thus, search engines can enrich the online environment with more educated and generalized public opinion by prioritizing content which demonstrates expertise and reliability.
At ThatWare, we follow the Knowledge-Based Trust Algorithm by ensuring quality fact-based information that our clients consume. From time to time, every material published shall be acceptable in quality and credible. This will thus give the clients authority in consuming them. We also undertake strategic backlinking with good and reliable sources. This leads to an increase in both credibility of content and also its search ranking. Online reputation tracking for our clients enables us to maintain a credible and authoritative digital presence on the internet. On fact and data credibility grounds, we enable businesses to place a foundational basis of trust among their target audiences. That would not only increase engagement among users but position our clients as sources that people in their respective industries can be made to rely upon, making them more prominent and effective in search results.
⭐️Augmented Reality Search
Augmented reality search integrates real-world visual information and digital search results for the user to interactively use. This method is unique in how it will give the user a new and immersive way of dealing with search results by directly overlaying information onto their real-world surroundings. For instance, the user can point a device at a location, product, or object, which in turn will trigger related information or recommendations. The search in AR transforms the interaction of a user with information, which is richer and more engaging than text-based results.
At ThatWare, we work to introduce innovations within the SD that would include augmented reality search and, therefore, ensure that our clients are always on the curve. We collaborate with firms to produce AR content with the aim of maximizing engagement and interaction with the users. The usage of AR technology helps in designing appealing experiences for the clients to resonate with the target audience; whether this has to do with the interaction of the product display or location-based information, it enhances the user journey while strengthening meaningful interactions and optimizing AR content for search visibility. It would allow clients to stand out, offering distinct solutions and demanding the attention of the user, which would ultimately come to traffic. Through ThatWare, enterprises would be able to tap into the real capability of AR and change the way in which customers are connected and value is delivered.
⭐️Multi-modal Fusion Algorithms
Multi-modal fusion algorithms are a great advance in search technology, where it can process queries with text, images, and audio to deliver richer responses. It recognizes that people look for information in all sorts of formats, and the ability to integrate all these modalities creates a much more comprehensive search experience. Being sensitive to the interplay among text, visuals, and audio, multi-modal fusion algorithms can provide users with contextually relevant results for answering queries more effectively. This is a strategy which can enhance user satisfaction by offering an information view which is holistically more satisfactory to them and their preferences and learning styles.
ThatWare uses multi-modal fusion algorithms to optimize the contents in such a manner so that the targeted masses can be targeted via respective channels by us. We work on the high-quality content that has text, images, and audio involved, thus creating a richer user experience. Additionally, we use advanced analytics to understand user behavior across different modalities and adjust our strategy in real time. Adopting the multi-modal approach helps businesses enhance their visibility and engagement, ultimately driving higher conversion rates. This forward-thinking approach does improve search performance but positions our clients as innovators in their industries. Businesses will find through the fusion algorithms of ThatWare that users can be offered a much richer and engaging experience-one that puts businesses on the map as more successful players in the ever-changing digital landscape.
⭐️Search Query Trend Analysis
Search query trend analysis involves examining emerging trends to predict and prioritize new search behaviors. By leveraging data analytics, SEO professionals can identify shifts in user interests, preferences, and search patterns. This proactive strategy helps businesses stay ahead of the competition by creating timely and relevant content that resonates with their target audience. By understanding these trends, brands can adjust their marketing strategies and content creation efforts to align with user demands, ultimately driving more traffic and improving engagement.
At ThatWare, we place a strong emphasis on search query trend analysis to inform our SEO strategies. Our team employs advanced analytics tools and methodologies to track and analyze data from various sources, such as search engines, social media platforms, and industry reports. By identifying emerging trends and changes in search behaviors, we can provide our clients with valuable insights that shape their content strategies. For instance, if we notice a rising trend in a particular topic or keyword, we can quickly develop relevant content to capitalize on that interest.
Additionally, we prioritize the use of predictive analytics to forecast future search behaviors based on current trends. This allows us to anticipate shifts in user intent and optimize content accordingly. We also work closely with our clients to ensure their marketing campaigns align with identified trends, maximizing their visibility and impact. By focusing on trend-driven strategies, we help our clients position themselves as thought leaders in their respective industries. Our approach not only enhances organic traffic but also fosters deeper engagement with their audience, leading to higher conversion rates.
In a rapidly evolving digital landscape, understanding and adapting to search query trends is crucial for sustained success. At ThatWare, our commitment to thorough analysis and data-driven decision-making empowers our clients to navigate the complexities of SEO with confidence. By leveraging search query trend analysis, we enable businesses to remain relevant and impactful, ultimately driving growth and establishing long-term relationships with their customers.
⭐️Temporal Intent Detection
Temporal intent detection is a sophisticated strategy that identifies whether a search query relates to past, present, or future events. Understanding the temporal context of a query allows businesses to deliver more relevant and timely results, significantly enhancing user experience. For example, if a user searches for “2022 Olympics,” they are likely looking for historical information, while a search for “upcoming concerts” indicates a desire for future events. By accurately determining temporal intent, brands can ensure they provide users with the most relevant information that meets their specific needs.
At ThatWare, we leverage advanced natural language processing (NLP) algorithms to implement temporal intent detection effectively. Our team analyzes the language and structure of search queries to uncover clues about the user’s intent. For instance, we focus on identifying keywords and phrases that indicate a specific timeframe, allowing us to categorize content accordingly. This approach enables us to create targeted content that directly addresses the needs of users based on their temporal queries.
Furthermore, we utilize data analytics to monitor user interactions with our content, helping us refine our understanding of temporal intent over time. By continually assessing the effectiveness of our strategies, we can adapt our content to better align with user expectations. For example, if we notice an increase in searches related to “past events,” we can develop content that provides comprehensive overviews or summaries, enhancing the user experience.
Our commitment to temporal intent detection also extends to optimizing our clients’ content for search engines. By ensuring that content is relevant to the identified temporal intents, we improve search rankings and visibility. Ultimately, our approach allows our clients to engage with their audience more effectively, driving higher traffic and conversions. In a fast-paced digital world, understanding temporal intent is crucial for businesses to stay relevant and meet the evolving needs of their users.
⭐️Privacy-Driven Ranking Models
Privacy-driven ranking models are increasingly important in today’s digital landscape, where data privacy is under intense scrutiny. These models aim to reduce reliance on personal data for ranking while ensuring compliance with privacy norms and regulations. By prioritizing user privacy, businesses can foster trust and demonstrate their commitment to ethical data practices. This strategy not only enhances user confidence but also ensures that brands are aligned with evolving privacy regulations, making them more attractive to consumers who value their data security.
At ThatWare, we embrace privacy-driven ranking models by implementing strategies that minimize the use of sensitive personal information. Our SEO practices focus on optimizing content based on user intent and search behavior rather than relying on individual data points. We use anonymized data analytics to understand user preferences while upholding strict privacy standards. By prioritizing privacy in our strategies, we help our clients create a reputable online presence that resonates with consumers’ expectations.
In our approach, we also emphasize transparency and user control over personal data. By informing users about how their data is used and providing options for data management, we enhance trust and loyalty. We ensure that our clients’ content adheres to ethical standards and prioritizes user privacy, positioning them favorably in search rankings.
Moreover, we continuously monitor changes in privacy regulations and adapt our strategies accordingly. This proactive approach allows us to stay ahead of compliance requirements while still delivering relevant and valuable content to users. By focusing on privacy-driven ranking models, we empower our clients to navigate the complexities of data privacy while maintaining their competitive edge. Ultimately, our commitment to privacy not only protects users but also strengthens brands’ reputations in an increasingly privacy-conscious marketplace.
⭐️Ethical Filter Mechanisms
Ethical filter mechanisms play a crucial role in ensuring that search algorithms remain free from biases and align with ethical standards. In an age where misinformation and biases can significantly impact user experiences, implementing ethical filters is vital for promoting fairness and equity in search results. By prioritizing ethical considerations, businesses can enhance the credibility of their content and ensure that their search strategies do not perpetuate harmful stereotypes or misinformation.
At ThatWare, we are dedicated to incorporating ethical filter mechanisms into our SEO strategies. Our team conducts regular audits of our algorithms to identify and address potential biases. We believe that promoting diversity and inclusion is essential for providing equitable search results. By analyzing our content for representation and ensuring that various perspectives are included, we help our clients build trust and credibility with their audiences.
We also prioritize transparency in our practices, allowing users to understand how search results are generated. This commitment to transparency fosters trust and accountability, enhancing user confidence in our clients’ brands. Our ethical filter mechanisms extend beyond algorithm audits; we actively promote content that reflects diverse viewpoints and experiences, ensuring that our clients’ messages resonate with a broad audience.
Additionally, we keep abreast of developments in ethical standards and best practices in the industry. By staying informed, we can continually refine our strategies and ensure that our clients are aligned with evolving ethical norms. This proactive approach not only enhances user trust but also positions our clients as leaders in their respective industries.
In an increasingly interconnected digital world, prioritizing ethical considerations in search strategies is essential. At ThatWare, our commitment to ethical filter mechanisms enables businesses to navigate the complexities of search algorithms while promoting inclusivity and fairness. Ultimately, our focus on ethics strengthens our clients’ reputations and enhances user satisfaction.
⭐️Ambient Computing in Search
Ambient computing in search refers to the ability to predict users’ information needs by analyzing ambient signals such as location, time of day, and device usage. This strategy enables businesses to deliver contextually relevant information, enhancing user experience and engagement. By understanding users’ immediate contexts, brands can tailor their content and marketing efforts to meet users’ needs effectively.
At ThatWare, we harness the power of ambient computing by analyzing various user signals to gain insights into their preferences and behaviors. Our team utilizes data analytics and machine learning to interpret these signals, allowing us to optimize content for specific contexts. For example, by assessing user location data, we can deliver search results that are highly relevant to users in that area, enhancing their overall experience.
Moreover, we focus on creating personalized content that responds to the time of day or user activity. For instance, if a user searches for “lunch spots” during lunchtime, we ensure that our clients’ content appears prominently in search results, capturing users’ attention at the right moment. This context-driven approach not only increases visibility but also drives higher engagement and conversion rates.
Additionally, we implement strategies to leverage ambient signals in our marketing campaigns. By aligning our content with users’ real-time needs, we enable our clients to effectively capture attention and foster meaningful interactions with their audience. Our commitment to ambient computing allows us to provide our clients with a competitive edge in the digital landscape, ensuring they remain relevant and impactful.
Ultimately, by embracing ambient computing in our search strategies, we help businesses connect with their audiences in more meaningful ways. At ThatWare, we understand that delivering contextually relevant information is crucial for enhancing user satisfaction and driving growth. Our approach empowers brands to leverage ambient signals effectively, positioning them for success in a dynamic digital environment.
⭐️User Journey Pathing
User journey pathing is a strategic way of visualizing the sequence of user interactions with a brand, starting from initial awareness to conversion and beyond. We at ThatWare recognize that mapping these journeys will improve user experience and drive engagement. We start with a comprehensive data collection process that spans various touchpoints, such as website visits, social media interactions, and email communications.
We also use analytical tools to understand and gather insights from heat maps, click-tracking, and session recordings. That helps find points of friction where users get confused or abandon a form. For example, analytics might indicate a lot of abandoned shopping carts; then, we will go ahead and find the root causes, possibly bad navigation or just complex checkout processes.
Once we see these issues, we start collaborating with the design and content teams about making changes targeted at each of those problems. Sometimes, it means redesigning website navigation to make access easier; product descriptions might need to be clearer, and checkout might need to be simpler so people don’t have a hard time buying. We always do A/B testing to determine whether or not the improvements we are making will drive a better user experience for the user.
By continuously tracking user pathways and iterating through our strategies, we establish seamless interactions that lead to conversions and lasting brand loyalty. Ultimately, our concentration on user journey planning allows us to deliver personally relevant experiences for users in ways that bring engagement and revenue for clients.
⭐️Advanced User Feedback Loops
Organizations need advanced user feedback loops to improve their digital offerings constantly. For ThatWare, this means creating systems that collect feedback and act meaningfully on it. This starts with multiple feedback channels, including surveys, user testing sessions, and forms placed on websites and applications.
We don’t just collect data; we analyze it rigorously through tools such as sentiment analysis and natural language processing, so we know not just what people are saying but also how they feel about an experience. For instance, if feedback says that users are frustrated with a particular feature, we find out why they are getting frustrated with it. In this regard, we conduct follow-up interviews and focus groups to get additional insights.
After aggregating and processing the feedback, we act. We work with product development teams to fix areas that hurt or with customer support teams to build better resources based on what users are asking for. We also run iterative testing cycles to understand these changes’ impact, ensuring a continuous cycle of improvement.
Importantly, we also emphasize closing the loop with users. Letting them know how their input has driven changes promotes a sense of community and shared ownership among them. Such engagement boosts satisfaction and fosters continued participation in the feedback process. Ultimately, our commitment to advanced loops in user feedback gives us the leverage to create more effective and user-centered experiences, leading to loyalty and a powerful brand reputation.
⭐️Cross-Platform Behavior Analysis
Understanding cross-platform behavior is crucial in today’s digital landscape because people interact with brands in multiple mediums. ThatWare employs robust analytics to gain a comprehensive view of user interactions, enabling our clients to understand how their audiences behave across various platforms—websites, mobile apps, or social media channels.
Our approach begins with gathering comprehensive data from digital touchpoints, which enables us to analyze user journeys that can span multiple platforms. We use analytics tools that integrate data from different sources, enabling us to create detailed user profiles. Profiling helps us identify specific patterns, such as users starting their journey on mobile devices and later completing a purchase on a desktop.
Understanding these cross-platform behaviors will help us design more holistic marketing strategies. For example, if we note that mobile users are frequent viewers of video content, we can focus video marketing campaigns on the mobile platform. We also ensure that branding and messaging remain consistent across all channels, building brand identity and trust.
A regularly executed audit for the cross-platform strategies shows their effectiveness as well. Using an analysis of click-through and conversion rates, we calculate the places needing upgrading. It is this nature of being iterative, fine-tuning our approach further, and thus ensuring we help our clients meet even raise user expectations. By monitoring cross-platform behavior analysis in each business, we will reach out to engage audiences in a much higher way. This eventually yields increased conversions and contributes to the long-term generation of customers.
⭐️Page experience signals
Page experience is one metric thought to help understand which website will rank highly for organic searches, for organic users at least. An organization must ensure that it leverages and enhances the various web properties under optimization. Thus, ThatWare identifies page experience aspects, including loading time, speed, interaction, and visual stability, among several others, and starts with an exhaustive estimation.
We audit the client’s websites using advanced tools like Google PageSpeed Insights and Lighthouse. Such tools give insights into performance metrics so we know exactly where to look to optimize. For instance, if a webpage is taking too long to load, we first work on optimizing images, leverage browser caching, and then minimize JavaScript to improve the speed.
In addition, we believe in mobile-first design principles, ensuring the user experience is seamless across all devices. Because mobile devices are increasingly used for browsing, websites must be responsive and able to load quickly on smaller screens. We use best practices like responsive design, mobile-friendly navigation, and easy-to-click buttons to make the website usable even on mobile devices.
Besides technical optimization, we also care about creating interesting content to keep users on the page longer. That’s through compelling visuals, clear calls to action, and intuitive layouts that make users bounce less and spend more time on the page. Our commitment to optimizing page experience signals better search engine rankings, but it also increases user loyalty and satisfaction, which can be the best long-term business success.
⭐️SERP Intent Matching
SERP (Search Engine Results Page) intent matching is critical to an effective SEO strategy. At ThatWare, aligning content with user intent is essential for driving relevant traffic and improving search rankings. Our approach begins with comprehensive keyword research to uncover the various intents behind user searches—informational, transactional, or navigational.
We analyze search queries to identify patterns and categorize them based on intent. For instance, a query like “best-running shoes” indicates an informational intent, while “buy running shoes online” reflects transactional intent. By understanding these distinctions, we tailor our content strategy to meet the specific needs of users at each stage of their journey. This might involve creating informative blog posts for users seeking knowledge and optimizing product pages for those ready to purchase.
Additionally, we monitor SERP features such as featured snippets, local packs, and related questions to identify opportunities for visibility. By optimizing content to appear in these features, we enhance our clients’ chances of attracting clicks and engagement. Our team continuously assesses performance metrics to refine our approach, ensuring our strategies align with evolving user behaviors and search algorithms.
Ultimately, our focus on SERP intent matching allows us to create content that ranks well and resonates with users. By providing relevant and valuable information tailored to user intent, we drive organic traffic and increase conversion rates, helping our clients achieve their business objectives.
⭐️Real-Time Data Indexing
Real-time data indexing is vital for maintaining the relevance and accuracy of online content. At ThatWare, we prioritize implementing strategies that enable immediate website content updates, ensuring that search engines and users have access to the latest information. Our approach involves leveraging advanced technologies such as server-side rendering and content delivery networks (CDNs) to expedite indexing.
We continuously monitor our clients’ content for updates and changes, ensuring that search engines index any new information promptly. For example, if a client launches a new product or publishes an important announcement, our systems are designed to notify search engines to crawl the updated pages immediately. This agility helps businesses stay competitive, especially in industries where timely information is crucial.
Furthermore, we analyze data patterns and search behavior to anticipate changes in user interests. Understanding what topics are trending, we can proactively update existing content or create new resources that align with emerging interests. This boosts search visibility and positions our clients as thought leaders in their respective fields.
In addition to content updates, we employ regular audits to ensure that all indexed pages maintain high-quality standards. This involves checking for broken links, outdated information, and overall relevance. By focusing on real-time data indexing, we enhance our clients’ online presence, ensuring they remain competitive and can effectively engage their audiences in a fast-paced digital landscape.
⭐️Predictive Search Analytics
Predictive search analytics is a powerful tool that allows businesses to anticipate user behavior and preferences based on historical data. At ThatWare, we leverage machine learning algorithms and advanced analytics to analyze trends and patterns, enabling us to forecast future search behaviors. Our approach begins with collecting extensive data across multiple channels, including website interactions, social media engagement, and email responses.
We can identify potential shifts in user interest or emerging topics by applying predictive modeling techniques. For instance, if we notice a growing trend in searches related to sustainability, we can proactively develop relevant content that addresses this interest. This positions our clients as thought leaders and helps them capture traffic before competitors respond to the trend.
Additionally, we use predictive analytics to optimize marketing campaigns. By understanding which demographics will likely engage with specific content, we can tailor our outreach strategies to resonate with target audiences. This personalized approach enhances user engagement and increases conversion rates.
To ensure the effectiveness of our predictive strategies, we continuously monitor performance metrics and adjust our tactics based on real-time data. This iterative process allows us to refine our predictions and stay ahead of user trends. Ultimately, our focus on predictive search analytics empowers businesses to make informed decisions, align their content strategies with user expectations, and drive meaningful engagement in an ever-evolving digital landscape.
⭐️Topic Modeling Algorithms
Topic modeling algorithms are essential for extracting meaningful insights from vast text data. At ThatWare, we utilize these algorithms to analyze content and identify key themes that resonate with target audiences. Our approach begins with employing advanced natural language processing (NLP) techniques to process large datasets, such as blog posts, articles, and user-generated content.
Algorithms like Latent Dirichlet Allocation (LDA) help us categorize content into distinct topics, helping us understand which themes are most relevant to users. This analysis informs our content strategy, enabling us to effectively create targeted resources that address user interests. For example, if our analysis reveals a growing interest in mental health topics, we can prioritize creating informative articles, videos, or podcasts.
Moreover, we continuously refine our topic modeling process to adapt to evolving user preferences. This involves regular updates to our datasets and algorithms, ensuring our insights remain relevant and actionable. We also analyze engagement metrics to gauge the effectiveness of our content strategies, allowing us to iterate and improve continually.
By focusing on topic modeling algorithms, we empower our clients to create content that resonates with their audiences, drives engagement, and enhances their online presence. This data-driven approach improves user satisfaction and positions brands as authorities in their respective niches, ultimately contributing to long-term business success.
⭐️Emotional State Detection in Queries
Emotional state detection in queries is a groundbreaking approach that enhances user experience by understanding the emotional context behind search requests. At ThatWare, we recognize that user intent is often intertwined with their emotional state, influencing how they interact with content. Our strategy employs advanced natural language processing (NLP) and machine learning algorithms to analyze user queries for emotional cues.
We begin by collecting a diverse dataset of search queries and user interactions, which we analyze to identify patterns associated with specific emotional states—such as frustration, curiosity, or happiness. For instance, queries that include “struggling with” or “need help” often indicate frustration or confusion. By categorizing these emotional states, we can tailor content to meet users’ needs more effectively.
Once we have identified the emotional context, we optimize content to resonate with users emotionally. This might involve creating empathetic messaging for distressed users or providing uplifting and positive content for those seeking inspiration. Addressing the emotional state reflected in queries enhances user engagement and satisfaction. additionally, we continuously monitor the effectiveness of our emotional state detection strategies through user feedback and performance metrics. This iterative approach allows us to refine our algorithms and content offerings, ensuring they remain relevant and impactful.
Ultimately, our focus on emotional state detection in queries enables ThatWare to deliver highly personalized user experiences. By aligning content with users’ emotional needs, we drive higher engagement, improve retention rates, and foster deeper connections between brands and their audiences, ultimately leading to increased conversions and brand loyalty.
⭐️Behavioral Analytics in Ranking
Behavioral analytics is crucial in understanding user interactions and preferences, significantly impacting search engine rankings. At ThatWare, we leverage behavioral data to optimize our clients’ content strategies, ensuring they resonate with target audiences while aligning with search engine algorithms. Our approach begins with comprehensive data collection from various sources, including website analytics, user sessions, and interaction logs.
We analyze user behavior patterns to gain insights into how visitors engage with content—what captures their attention, leads to conversion, and causes drop-offs. For instance, if users frequently abandon a page after a specific section, we investigate that content to identify potential issues, such as unclear messaging or insufficient information.
By understanding these behavioral signals, we can optimize content to enhance user engagement and satisfaction. This may involve restructuring web pages, improving calls-to-action clarity, or creating more compelling headlines. Furthermore, we employ A/B testing to evaluate the impact of these changes, allowing us to refine our strategies based on real-time data.
Additionally, we integrate behavioral analytics into our SEO efforts, ensuring that content is relevant to users and favored by search engine algorithms. By aligning user behavior insights with SEO best practices, we enhance our clients’ chances of achieving higher rankings and increased visibility.
Ultimately, our focus on behavioral analytics in ranking empowers ThatWare to create user-centric content strategies that drive engagement, improve search rankings, and contribute to overall business success. We help our clients maintain a competitive edge in the digital landscape by continually monitoring and adapting to user behavior.
⭐️Query Clarification Models
Query clarification models are essential for improving search engine performance by ensuring users find the most relevant information for their needs. At ThatWare, we implement sophisticated algorithms that analyze user queries to identify ambiguities and suggest clarifications that enhance search accuracy. Our approach begins with understanding the nuances of user intent and the potential for misinterpretation.
We collect and analyze large datasets of search queries to identify common patterns of ambiguity. For instance, a query like “jaguar” could refer to the animal or the car brand. By leveraging contextual information and historical data, we can develop models that disambiguate such queries and provide users with the most relevant results.
When users encounter ambiguous search results, our query clarification model prompts them with options or follow-up questions that help narrow down their intent. This enhances the user experience and increases the likelihood of conversions, as users receive results that more accurately match their needs.
Moreover, we continually refine our query clarification models based on user feedback and search behavior. By analyzing the effectiveness of our clarifications—such as click-through rates and user engagement metrics—we can iterate on our approach to ensure optimal performance.
Ultimately, our commitment to query clarification models allows ThatWare to deliver precise and relevant search results. By minimizing ambiguity and aligning content with user intent, we enhance the overall search experience, increasing user satisfaction and loyalty.
⭐️Language Translation and Localization Algorithms
Language translation and localization algorithms are vital for businesses seeking to reach global audiences effectively. At ThatWare, we understand that merely translating content is insufficient; it must also resonate culturally and contextually with target audiences. Our approach incorporates advanced machine translation and natural language processing techniques to ensure content is accurately translated and appropriately localized.
We begin by analyzing the source content to identify idiomatic expressions, cultural references, and nuances that may not translate directly. Our linguistic experts collaborate with algorithm developers to create a comprehensive localization strategy that addresses these challenges, ensuring that translated content is accurate and culturally relevant.
Additionally, we implement localization tools that adapt content to meet regional preferences and norms. This includes adjusting date formats, currency, and measurements and modifying visuals and graphics to align with local customs. We enhance user engagement and satisfaction by creating a tailored experience for users in different markets.
Furthermore, we continually evaluate the effectiveness of our translation and localization efforts through user feedback and performance metrics. This iterative process allows us to refine our algorithms and strategies, ensuring they remain effective as language and cultural trends evolve.
Ultimately, our focus on language translation and localization algorithms empowers ThatWare to help clients expand their reach and connect with diverse audiences. By delivering culturally relevant content, we enhance brand loyalty and drive conversions, positioning our clients for success in the global marketplace.
⭐️Multi-Intent Query Parsing
Multi-intent query parsing is essential in today’s complex search landscape, where users often have multiple intentions behind their queries. At ThatWare, we leverage advanced natural language processing (NLP) techniques to analyze and decode these complex queries, ensuring that users receive relevant results that cater to their diverse needs. Our approach begins with extensive research into user behavior and query patterns to identify common instances of multi-intent searches.
We employ machine learning algorithms to dissect queries, allowing us to recognize and prioritize different intents within a single search. For example, a query like “best running shoes for marathons and casual wear” contains multiple intents: one for performance running shoes and another for casual footwear. We can create targeted content that addresses each query aspect by accurately parsing these intents.
To enhance user experience, we develop user-friendly interfaces that present search results in a way that acknowledges and fulfills multiple intents. This might involve displaying separate sections for different categories or providing filters that allow users to refine their search based on specific criteria.
Additionally, we continuously analyze user interactions with multi-intent queries to gauge the effectiveness of our parsing strategies. By monitoring engagement metrics and user feedback, we can refine our algorithms and improve the accuracy of intent recognition.
Our commitment to multi-intent query parsing ultimately empowers ThatWare to deliver more nuanced and relevant search results. By aligning content with users’ diverse needs, we enhance engagement, drive conversions, and foster long-term brand loyalty.
⭐️Zero Search Result Optimization
Zero search result optimization addresses instances when users conduct searches but find no relevant results. This scenario can lead to user frustration and increased bounce rates, negatively impacting brand perception. At ThatWare, we implement strategies to minimize zero search results and enhance user satisfaction. Our approach begins witha thorough analysis of search queries that lead to zero results, allowing us to identify common themes or gaps in content.
Once we understand the nature of these queries, we work to create or optimize content that aligns with user intent. This might involve developing new resources, such as blog posts or FAQs, that address specific topics users search for but can’t find. Additionally, we optimize existing content by incorporating relevant keywords and phrases that align with user queries.
We also focus on enhancing the user experience when zero search results occur. This could involve displaying related topics or suggesting alternative queries that provide valuable information. Providing users with options keeps them engaged and reduces the likelihood of abandoning the site.
Furthermore, we continually monitor search analytics and user feedback to refine our strategies. By assessing the impact of our optimizations on user behavior, we can adjust our approach to ensure ongoing effectiveness.
Ultimately, our commitment to zero search result optimization enables ThatWare to enhance user satisfaction and drive engagement. By addressing gaps in content and improving the overall search experience, we help our clients foster positive interactions and build long-term brand loyalty.
⭐️Anomaly Detection for Search Quality
Anomaly detection for search quality is critical for maintaining optimal user experience in digital environments. At ThatWare, we implement advanced machine learning algorithms to identify and address anomalies that may indicate potential issues in search performance. Our approach begins with establishing baseline metrics for search quality, including relevance, speed, and user engagement.
We collect extensive data on search queries and user interactions, allowing us to monitor performance trends over time. When deviations from the established norms occur—such as sudden drops in click-through rates or increased bounce rates—our anomaly detection systems flag these irregularities for further investigation.
Once an anomaly is detected, we conduct a thorough analysis to determine its root cause. This might involve examining changes in search algorithms, content updates, or shifts in user behavior. For instance, if we observe a spike in zero search results for specific queries, we investigate whether new content needs to be created or optimized to meet user intent.
We can proactively address anomalies and maintain high search quality and user satisfaction. We continually refine our detection algorithms based on historical data and feedback, ensuring they adapt to evolving trends and behaviors.
Ultimately, our focus on anomaly detection for search quality empowers ThatWare to deliver exceptional user experiences. By quickly identifying and addressing issues, we help our clients maintain their competitive edge and foster long-term relationships with their audiences.
Personal Data Minimization Models
Personal Data Minimization Models are about gathering only a minimal amount of information as is necessary for the users without excessive reliance upon their info. This, therefore, allows the service provider to provide appropriate content that is user-specific but not to the extent that it may break various privacy regulations and win further user trust. A way in which this will keep offering engaging experiences with fewer invasions of the consumer’s rights is through furthering the concept of collection minimization.
At ThatWare, we implement such models by focusing on user intent rather than collecting masses of personal data. We recognize genuine user needs through patterns in search and user interaction. For example, if a user searches for “best eco-friendly packaging,” we’re focused on the user’s underlying motivations: information seeking or product recommendation, which means the content we’re producing is relevant and of value.
We also leverage first-party data gath user interactions on our website, like feedback forms and newsletter sign-ups, to tailor content to individual preferences without invasive tracking. Dynamic content personalization allows us to highlight related articles or products based on user behavior, creating engaging experiences while safeguarding privacy. A/B testing further refines our strategies, gathering meaningful insights without excessive data collection. For example, testing different versions of landing pages can determine which elements best resonate with our eco-conscious audience. Personal Data Minimization Models mean ThatWare is balancing a commitment to a personalized user experience with an equally strong commitment to privacy. We are differentiating our approach in the competitive space, focusing on understanding user intent, maximizing first-party data usage, and optimizing through testing while ensuring users obtain relevant content based on needs and that their privacy will be respected.
⭐️Semantic Proximity Detection
Semantic Proximity Detection in modern SEO identifies the closeness of words’ meanings within content and improves their relevance in the search engine results. This method is more advanced than keyword matches since it provides context for words and the relationships within them, which helps search engines better understand what a given query means. Using this semantic proximity helps businesses put out content that fits their intent better, thus generating more engagements and higher ranks.
At ThatWare, we apply Semantic Proximity Detection to ensure our content is rich in relevant keywords and captures the wider context of user queries. This is achieved through related terms and phrases commonly used in c the target keywords, thus giving our content-richantic meaning. For example, while preparing content for “organic skincare,” we are not limited to using only the term organic skincare but also employ other terms like natural ingredients, sustainable practices, and eco-friendly packaging, which has added volume to our content and made it more relevant to the actual search queries of users.
ThatWare further scans competitors’ content and understands the lacuna in semantic coverage by using very advanced AI tools. Understanding the semantic landscape surrounding a specific topic will help us create unique content relevant to user needs more thoroughly than those of competitors. For instance, suppose our competitors rank for “benefits of organic skincare.” We can expand our focus to cover “impact on skin health,” “environmental benefits,” and “user testimonials.” This way, not only will the content be more relevant, but we will also stand out as a thought leader in the niche.
What will make ThatWare take the leading stride above its competitors will be semantic proximity detection—the tion of complex yet rich in semantics context-driven contents matching the intent of the user. This would enable semantic relation appreciation toward perfecting our semantic play while ensuring improved chances at creating meaningful engagement.
⭐️Self-Learning Algorithms
The most innovative approach in SEO is self-learning algorithms, which learn to improve their performance autonomously by analyzing new data and usage patterns. Self-learning algorithms do not work on static rules like traditional algorithms but adapt to real-time information, making them maximally effective in giving relevant search results. This is essential in the fast-paced digital world because user behaviors and preferences constantly change. ThatWare, we continually use self-learning algorithms to optimize our SEO strategies. We can identify trends and shifts in user behavior by integrating learning models that analyze user interactions with our content. For instance, if we find out that people spend more time reading or are more engaged with some types of blog posts than others, we can update our content strategy to give more emphasis to those topics. This adaptive approach ensures we keep up with the market trends and makes our content relevant and engaging.
ThatWare also refines keyword targeting over time because self-learning algorithms allow it to. Our algorithm can determine what words to focus on based on performance metrics by analyzing our users’ varied keyword and phrase responses. If a keyword that never previously performed well suddenly became popular, our adaptive learning system would recognize a behavior change and learn to optimize content around said keyword to capitalize on any new opportunities. Fluidity in SEO practice such as this sets us apart from the competition, which cannot adapt at such lightning speeds.
Gamification of User Engagement
Gamification is the process that allows game-like elements to be integrated into non-gaming contexts so that the environment is made more engaging and motivating. For SEO, gamification can be the most viable option to heighten users’ engagement with content. This technique makes users enjoy the process and increases their chances of retaining themselves on the platform for a long time, while also promoting loyalty.
Gamification makes the application engaging for users in that there are interactive quizzes and polls concerning issues with our blogs. We would call them upon to try their understanding or state an opinion regarding some blog-related issues. We make this user spend much more time on our website, so if that time elapses, inform search engines that we are adding quality. Brand loyalty significantly improves, making their learning experience enjoyable and drawing the users back.
In addition, we have reward systems wherein we give points or badges for using our content. Ways to use content may include completing quizzes, sharing articles, or commenting on comments in the article. Such recognition creates a feeling of achievement and belonging among users, increasing engagement with the content. For example, those who have gained points may receive service discounts or access to certain exclusive content, further pushing people to participate.
Last but not least, gamification helps validate the content. When users engage with our quizzes or comment on an article, they are helping to contribute to the overall quality and relevance of our content. ThatWare’s social validation through user input will then increase the credibility of information, thus increasing organic traffic. This givemakestWare a different gamification in SEO, making user engagement a fun, rewarding experience. By including game elements that help validate the content, I do not get higher engagements and keep my authentic content, which brings long-term growth and loyalty.
⭐️Edge AI Processing for Search
Edge AI processing executes AI algorithms close to the source of data, whether mobile devices or some other form of edge hardware, instead of relying strictly on centralized cloud servers. This significantly cuts research results’ latency, making more responsive user experiences. It is possible that more edge AI improves user privacy since less personal information crosses the internet, creating a safer browsing environment.
We’re using edge AI processing to optimize SEO strategies for mobile users at ThatWare. This involves using driven algorithms that run directly on devices to analyze real-time user behavior and preferences. For instance, when a user searches for “best hiking trails,” our edge AI processes this request in real-time, returning relevant, localized content without delay from server-based processing. This rapid response fulfills the users’ needs for more satisfaction and enhances the possibility of conversion; they engage more when the content is available quickly and relevant to their search needs.
In addition, ge AI enables us to process users’ privacy when delivering customized experiences. By minimizing data transmission, we can assure our clients that their personal information is being held in safe custody. For example, our algorithms may operate locally on the user’s device instead of sending sensitive location data to a central server. This preserves privacy yet returns highly relevant search results. Furthermore, analyzing user interactions on-device enables dynamic content recommendations so that the most pertinent information reaches users. Overall, ThatWare’s advantage of AI processing keeps us miles ahead of the competition by deploying fast, privacy-sensitive searches with personalized user experiences, which all create loyalty and increase growth.
👉Advanced Topics in Information Retrieval, LLMs, and Page Relevance
Advanced topics in information retrieval optimize how the contents are analyzed and returned for specific user queries through a particular search engine. Massive growth in the application in this field has occurred since the integration of the LLM, which enhances understanding and processing of the natural language. Capabilities of the search engine can appreciate nuances of user intent to make the search much more accurate. Concepts that include semantic search, awareness of context, and personalization are also becoming increasingly indispensable. These developments ensure that users are not given relevant pages and content suited to their preferences and needs. Some of the important points in this area include the following, which have been developed to enhance the relevance of pages and improve user interaction.
⭐️Hierarchical Clustering of Search Results
Hierarchical clustering is a method applied in information retrieval to group search results into a structured format. This allows users to access complex queries in an easy-to-use format. It would lead to an improved user experience and more efficient grouping of similar characteristics or shared themes. For instance, if a user types in something like “best smartphone,” results can be separated into sub-groups that feature “budget smartphones,” “high-end smartphones,” “gaming smartphones,” or so forth. That facilitates quick information retrieval instead of wading through useless answers.
ThatWare uses hierarchical clustering to fine-tune the search experience for clients. ThatWare creates sharply defined clusters of content cut across different aspects of the query based on queries and client search behavior. Advanced data analytics techniques are involved in recognizing key topics and keywords associated with the content to organize their search results effectively. For example, if a visitor searches on “travel tips,” ThatWare can show “budget travel,” “luxury travel,” and “family travel tips” groups so users can navigate to areas of interest easily without additional click-throughs or website browsing.
This has helped not only with user navigation ease but also in SEO, as when a search engine deems that the site is using hierarchical clustering, it likely pushes sites up for better rankings. Unlike competitors that would linearly post results, ThatWare’s approach is structured, making users find what they need within the shortest time possible and thus lowering conversion rates with increased time on site. That makes it stand out among the rest in the competitive landscape, positioning it as the leader in the delivery of user-centric search experiences.
⭐️Contextual Synonym Embedding
Contextual synonym embedding is a complex NLP technique that enhances the understanding of synonyms in a given context. Unlike earlier methods, where synonyms are always treated interchangeably regardless of context, this technique understands that the meaning of a word changes significantly depending on the context in which the word appears. For example, “bank” refers to a financial institution or the side of a river. The better contextual word embedding, the better quarter query interpretations will be and the more relevant the results.
Using contextual synonym embedding improves relevance and search engine ranking. A firm uses deep models for machine learning. They considered large amounts of datasets to identify the actual meaning of the synonyms.
The same enables ThatWare to make better contentful productions of the intent that searchers want. For example, when searching for “automobile,” the system should understand that related terms such as “car,” “vehicle,” or “motor vehicle” may have a different importance ranking depending on the text context.
This approach makes ThatWare unique compared to competitors, who may be based only on keyword matching. For example, if a client sells cars and the content on their website uses “autos” and “vehicles,” ThatWare ensures that the content is contextual to the potential searches, such as “best family cars” or “affordable sedans.” This subtle understanding improves user engagement since visitors find more relevant information faster. In addition, search engines prefer sites that use contextual synonym embedding, thus ranking better. This strategic use of NLP only adds to the users’ quality of experience while forcing ThatWare’s presence as a competitive SEO.
⭐️Multi-hop Reasoning
Multi-hop reasoning is an advanced information retrieval technique that uses tactical flows of steps that are erected over pages or sources. This approach mirrors human reasoning because it requires one to generate information from several sources rather than relying on one source. For example, if a user asks, “What are the health benefits of green tea, and how does it compare to black tea?” a multi-hop reasoning system would collect information from multiple articles and studies to deliver a coherent answer, both on the benefits of green tea and its comparison with black tea.
ThatWare uses multi-hop reasoning in its SEO work to make the content more relevant and deep. ThatWare uses complex algorithms that analyze and aggregate information from multiple trusted sources to ensure that the content produced addresses the user’s query comprehensively. ThatWare produces content that answers multiple sides of a question, thus making its clients stand out in search results.
For example, if the user seeks “the benefits of meditation and how to start,” ThatWare would compile all information on psychological benefits, improvement in physical health, and practical ways to begin meditation from authoritative articles. This way, the information is much more comprehensive, answering the question while keeping the user occupied and spending more time on the site.
This technique differentiates ThatWare from competitors that may only provide surface-level answers. By providing in-depth, multi-faceted responses, Ware enhances user satisfaction and the possibility of higher rankings in search engines. Ultimately, multi-hop reasoning enriches the user experience, making content more informative and valuable, forcing ThatWare’s position as a leader in the SEO landscape.
⭐️Vector-Based Search Retrieval
Vector-based search retrieval is an innovative approach that utilizes mathematical representations, or vectors, of words and phrases to improve search accuracy beyond traditional keyword matching. This method allows search engines to understand the semantic meaning of queries and the relationships between words, enabling them to deliver more relevant search results. By representing text as vectors in a high-dimensional space, the system can determine the proximity of different phrases. This allows the system to capture context and nuance that simple keyword searches often miss.
ThatWare harnesses vector-based search retrieval in its SEO practices to provide clients with content that resonates more effectively with users. By eUsinganced Natural Language Processing (NLP) techniques, ThatWare transforms queries and web pages into vector representations, facilitating a deeper understanding of user intent. This means that when a user searches for terms like “best plant-based protein sources,” the system can identify related concepts such as “vegan protein,” “nutritional benefits,” and “plant nutrition,” even if those terms aren’t explicitly stated in the search.
For instance, if a user searches for “how to boost productivity,” traditional keyword matching might only return articles with that exact phrase. In contrast, ThatWare’s vector-based approach would consider synonyms and related terms, providing results that include articles on “time management,” “effective habits,” and “work-life balance.” This comprehensive response not only enhances user satisfaction but also increases the likelihood of clicks and engagement.
This strategy sets ThatWare apart from competitors who primarily rely on keyword-based methods. By focusing on the underlying meanings and relationships of terms, ThatWare can create more relevant content that is better aligned with what users genuinely seek. This commitment to understanding semantic context ultimately leads to improved rankings and visibility in search engine results, solidifying ThatWare’s reputation as a leader in innovative SEO solutions.
⭐️Transformer-Based Summarization
Transformer-based summarization is an advanced technique that leverages transformer neural networks to condense extensive content into concise summaries without losing critical information. This method is especially beneficial in the era of information overload, where users often seek quick insights rather than detailed documents. By understanding the context and relationships within the text, transformer models generate summaries that encapsulate the essence of the original content, making it easier for readers to grasp the main ideas quickly.
At ThatWare, transformer-based summarization is seamlessly integrated into the SEO strategy to enhance user experience and engagement. The company utilizes state-of-the-art transformer models like BERT or GPT to analyze web content and extract key points laid prominently on client websites. This approach not only aids users in digesting information quickly but also helps improve the website’s overall SEO performance by keeping users engaged and reducing bounce rates.
For instance, if a user searches for “benefits of remote work,” the content of that client’s site might include a detailed article. However, ThatWare employs transformer-based summarization to create an engaging summary highlighting the key advantages such as flexibility, increased productivity, and work-life balance. This summary could be positioned at the top of the article or even used as meta descriptions, ensuring that potential visitors immediately see the most relevant information. Rights focus on effective summarization, differentiating ThatWare from competitors who might present lengthy articles without highlighting essential insights. By providing succinct and informative content, ThatWare does not meet user expectations and search engine algorithms that favor concise, relevant information. This innovative approach enhances user satisfaction, encourages deeper site exploration, and boosts search rankings, making ThatWare a standout in the competitive SEO landscape.
⭐️Cross-Lingual Information Retrieval (CLIR)
CrosCross-lingual information retrieval (CLIR) is an advanced search technique that enables users to retrieve information in multiple languages. This approach is particularly useful in our increasingly globalized world, where users may search for information using queries in one language but want results in another. CLIR utilizes sophisticated algorithms to translate and interpret complex queries, ensuring that relevant content is accessible regardless of the language in which it was originally written. By bridging language barriers, CLIR enhances the user experience and broadens the scope of available information.
At ThatWare, CLIR is integrated into its SEO practices to expand client reach and visibility in diverse markets. Implementing state-of-the-art language processing and machine translation technologies, ThatWare can translate user queries into multiple languages, providing more comprehensive search results. For example, if a user searches for “best travel destinations” in English, ThatWare’s CLIR capabilities can retrieve relevant articles, blogs, or reviews written in French, Spanish, or other languages, ensuring that users receive the most pertinent information avahis multilingual approach not only helps ThatWare’s clients attract a more diverse audience but also sets them apart from competitors who may only focus on English-language content. By offering relevant content across various languages, ThatWare enhances user engagement and satisfaction, leading to higher conversion rates. Moreover, the company’s ability to rank for multilingual queries provides clients a significant competitive advantage, driving organic traffic from international markets. This focus on Cross-Lingual Information Retrieval demonstrates ThatWare’s commitment to innovation and user-centric solutions, ensuring it can connect with a broader audience while meeting their specific information needs.
⭐️Domain-Aware Ranking Models
Domain-aware ranking Models are specialized algorithms designed to customize search rankings based on specific domains or industries, such as medical, legal, or financial sectors. Unlike traditional ranking systems that apply a one-size-fits-all approach, domain-aware models consider the characteristics and requirements of different fields, ensuring that the most relevant and authoritative content is prioritized. This targeted approach enhances the user experience by delivering more pertinent results, improving engagement and satisfaction.
At ThatWare, domain-aware ranking models are an integral part of their SEO strategy, allowing them to optimize content effectively for various industries. Utilizing advanced data analytics and machine learning, ThatWare tailors its ranking algorithms to reflect different domains’ nuances and specific needs. For instance, when working with clients in the medical field, ThatWare prioritizes authoritative sources and peer-reviewed studies to ensure that users receive credible information about health-related queries. In contrast, for legal topics, the focus shifts to case law, legal precedents, and documents from reputable law firms.
This specialized approach not only enhances the quality of search results but also differentiates ThatWare from competitors who may lack this level of granularity in their SEO practices. Delivering highly relevant and domain-specific content, ThatWare helps clients rank better in their respective industries. For example, a healthcare client leveraging ThatWare’s domain-aware models could see significant improvements in organic traffic and conversions due to higher rankings for critical keywords in medical search queries. This commitment to domain specificity underscores ThatWare’s dedication to providing tailored solutions that align with clients’ unique needs, ultimately fostering greater trust and authority within their respective markets.
⭐️Dense Passage Retrieval (DPR)
Dense Passage Retrieval (DPR) is an advanced information retrieval technique that utilizes dense embeddings to locate and rank highly relevant text passages within large datasets. Unlike traditional keyword-based search methods, which rely on exact matches, DPR leverages machine learning models to understand the semantic meaning of queries and documents. By representing both queries and passages as high-dimensional vectors, DPR can effectively identify and rank content that closely aligns with user intent, even if the wording differs from the search terms.
ThatWare effectively implements the Dense Passage Retrieval (DPR) SEO strategy by creating content rich in semantic relevance. This approach allows them to capture a wider array of user queries, ensuring their clients’ websites rank for diverse related terms. For example, when optimizing a client’s site about sustainable living, ThatWare uses DPR to analyze user queries about eco-friendly practices, thus surfacing relevant passages that may not explicitly mention the searched keywords but are still contextually related. This enhances the likelihood of engaging users who seek comprehensive information on sustainability topics.
By utilizing Dense Passage Retrieval, ThatWare sets itself apart from competitors who may rely solely on traditional search optimization techniques. The ability to rank nuanced, semantically related passages allows clients to reach broader audiences and improve overall content visibility. A practical example of this can be seen in the travel industry: a client specializing in eco-tourism might benefit from DPR by appearing in search results for queries about green travel tips, even if those specific phrases aren’t peer-reviewed articles. This strategic advantage increases organic traffic and enhances user satisfaction, solidifying ThatWare’s position as a leader in innovative SEO practices.
⭐️Multi-document Summarization
Multi-document summarizations are the most powerful summarization techniques that summarize information from many sources. Areese provides a condensed answer to very wide-ranging questions, but they are correct. It actus users very much while searching for information on general topics spread over several articles or documents. This means that multi-document summarizations improve efficiency but also richen the user experience relative to answers that are not only understandable but also lucid. This is one of the techniques that assist in properly making decisions regarding complex queries that need an all-rounded view.
It also sets ThatWare apart from the others as it uses multi-document summarization in its SEO practices to make the content more relevant and engaging for users. Instead of merely using single-source content, ThatWare analyzes and summarizes data from several documents to answer user queries better. For instance, if ThatWare is creating content for a healthcare client, it can retrieve information on the same medical condition from thousands of research papers, articles, and case studies and present a synthesized summary of symptoms, treatments, recent studies, and much more to the user, acting as a one-stop shop.
By using multi-document summarization, ThatWare has the unique ability that its competition may not necessarily have a focus on separate articles only. This enhances the content quality while creating clients with authoritative status over their given fields, as clients will benefit from being provided a multi-document summary that assembles necessary information about how el Inc., including instructions and local intelligence from diverse sources. This rich content allows for better search visibility and builds trust in the content. The more probable end-user engagement is followed, and appropriate conversion results can be anticipated. So, in these aspects, ThatWare, with its use of multi-document summarization, innovates and stands ahead of competitors in the world of SEO.
⭐️Conversational Context Memory
It refers to one of the sophisticated and higher-order features for retaining all interactions shattered from users previously to qualify the responses made and which would come after users’ questions. This power of recalling prior interactions would be especially important in SEO because it ensures seamless and personalized experiences and provides content that can cater to the user’s best interest, thereby improving their engagement and satisfaction levels. It gives the capability of knowing more about what people want and how they respond to certain stimuli to make the conversation even more effective.
ThatWare applies conversational context memory in its SEO strategy by monitoring user-to-user intetouchpoint interactions. That way, ThatWare can track user queries, preferences, and engagement patterns to ensure a more precise response to subsequent queries. This information can help Ware find relevant data when a user asks for recommendations based on his previous interests after enquiring about a specific product on an e-commerce website. Thus, it ensures consistency and relevance of suggestions sent to the users, increasing conversion chances. While the competition may be so focused on the end that they forget the value of user interaction history, ThatWare’s approach boosts the SEO efforts of its clients by making the experience for the user more personal. For example, a travel industry client will greatly benefit from the conversational context memory if he or she can provide specific travel recommendations based on previous inquiries about destinations or activities. By making the experience more engaging and personalized, ThatWare delights a user and contributes more traffic and better conversions to drive its clients toward the forefront of their particular market. ThatWare makes itself known as a groundbreaking player in the SEO space and improves user interaction via context memory.
⭐️Contextualized Entity Linking
Contextualized entity linking is an advanced method that ensures all search queries are related to the right entities, producing more accurate results and consequently improving a user’s experience with any results. These methods distinguish entities with meanings like “Apple,” which might be a company dealing in technology or, of course, the fruit itself, by assessing the context behind each query.
This is a reality for ThatWare through its utilization of semantic search techniques with natural language processing and machine learning algorithms, which interpret intent within actual search queries. The algorithm has the relationship mapping word phrases and then links based on the more significant view. We at ThatWare continue refining our approach by utilizing data points like user searches, content trends, and entity recognition to ensure that there is an accurate match for whatever users intend to look for.
Being one of their SEO strategies in embedding the same, our experts at ThatWare also provide our client with a good competitive edge at the given time because keyword and correct entity alignment give your content all the chances for reaching an appropriate audience with a decent level of users’ interest. Such usability results increase engagement, leading to appropriate search rankings supporting the target clients.
⭐️Sentence-BERT for Similarity Matching
The use of Sentence-BERT will boost the precision of their SEO strategy’s content relevance. With the help of Sentence-BERT, the company can now analyze and compare the semantic similarity between sentences to ensure that the search results are well aligned with the users’ intent.
Using this advanced model, We at ThatWare can take sentences and convert them into dense vector representations to determine contextual similarity across large datasets. This approach is specifically helpful in refining search results since ThatWare can rank content based not only on keyword matches but also on the subtleties of meaning and relationships in the text.
In a way, this process trains the Sentence-BERT on highly diversified text data, whereby the model understands the various nuances of the context along with the varying expressions of language. Using this technology, our team at ThatWare ensures that clients’ content reaches relevance that connects with the user’s query. Thus, it results in higher accuracy searches, improved click-throughs, and more user involvement. The bottom line finally enables ThatWare to build personalized content solutions that result in higher views and increased organic traffic.
⭐️Intent-Aware Query Expansion
The approach that an intent-aware query expansion considers is examining underlying intention while determining the correct terms to seek when ambiguity refers to a question. Even though queries are expanded to be filled with related synonyms or topics to search for, that improvement in service to the search experience is centered upon capturing several meanings within the application of one query. This will enable businesses to be more visible on the Web, reach a greater audience, and engage with content that resonates with users.
We at ThatWare exploit advanced NLP algorithms that analyze user behavior and query patterns in intent-aware query expansion. It studies historical search data and user interactions to establish constant synonyms and relevant topics aligned with a user’s intent. By creating a solid database of terms and phrases, we can expand ambiguous queries intelligently. This ensures that clients’ content is optimized for several relevant search terms, enhances search engine visibility, and drives qualified traffic to clients’ websites, further increasing user engagement and conversion rates.
⭐️Cross-Attention Mechanisms for Document Relevance
The cross-attention mechanisms enhance document relevance by allowing the processing of multiple documents simultaneously to identify interconnections and how they are related to a user’s query. It helps gain a deeper understanding of the relationship and hierarchy between content and, therefore, improves the contextual relevance of search results. Focusing on the interaction and information sharing of different documents can help identify the most relevant content for fulfilling user intent. This implies ranking the documents so that users eventually get answers of more contextual value to their searches, and user satisfaction and engagements are even higher.
At ThatWare, cross-attention is implemented by complex machine learning algorithms that search multiple documents and web pages. It would allow ThatWare to recognize the key themes and relevance scores for each document with models that assess relationships between different sources of information. This analysis helps optimize client content to match user queries and search engine algorithms. Using these findings, we at ThatWare provide better SEO services in terms of the content brought to the clients that would be ranked better, increase the chances of gaining exposure, drive traffic, and enhance overall engagement on the clients’ websites.
⭐️Dual Encoder Models for Search
The dual encoder models are revolutionary as they encode the query and document independently; it’s fast and scalable for retrieval. This approach improves the search process by enabling the query and the document to be represented simultaneously in a shared vector space. Since both elements have been converted to fixed-size embeddings, the dual encoders can quickly compute similarities, and it becomes easy to retrieve the relevant documents based on user queries. This model would be efficient with large amounts of data, ensuring a prompt and accurate search outcome while optimizing the search experience by minimizing latency and improving retrieval performance.
At ThatWare, our team implements dual encoder models that use state-of-the-art machine learning frameworks to create efficient embeddings for client queries and their respective content. By encoding these in isolation, we quickly find the most contextually aligned documents for a particular user query in real time. Beyond saving time on retrieval, accuracy is further improved by retrieving results contextual to the original intent of the user query. Integration of dual encoder models within their SEO packages enhances client content visibility, increases user satisfaction, and drives higher engagement through efficient delivery of search results.
⭐️Causal Reasoning in Search
In search, causal reasoning seeks causes in information rather than mere correlation. The analysis of causal relationships is better to assess the relevance of content to any query from a user. This will uncover deeper insights into how factors influence search results into more meaningful and contextually accurate outcomes. By focusing on causation, the approach improves the user experience by providing information not only matched by keywords but also related to the causes that motivated users to start searching in the first place.
Our team at ThatWare overlays the clients’ content with causal reasoning and advanced analytical frameworks to analyze everything, every data point, and their relationship. How certain topics interact forms a better picture of relevance for the user queries, thereby requiring deeper analysis and observation of user behavior in interaction with content. It thus identifies the underlying drivers of user engagement. By incorporating causal reasoning in our SEO strategies, we improve relevance and accuracy in response to the needs and intent of user traffic and better engagement for the clients.
⭐️Self-Supervised Language Model Pre-training
Self-supervised pre-training of a language model refers to training large language models on enormous datasets without requiring labeled data. Thus, it helps better capture the patterns in the language, context, and semantics in which it is expressed. Therefore, the model will likely give a more apt and coherent answer. They also acquire fine meanings and relationships from the enormous textual information they own. This means that the pre-trained LLM will better understand the user query and add more contextually relevant content, thereby greatly enhancing users’ search experience with rapid and efficient findings.
At ThatWare, we leverage self-supervised language model pre-training to help make our SEO services stronger for businesses. We train the LLMs on many different datasets so that they build an understanding of the subtlety of language and the context involved. Thus, we are in a position to enable our models to give more relevant content and gain a deeper understanding of the queries being asked by the users. A deep learning approach will enable the generation of optimized content that supports our user intent and drives traffic to our client sites. Our ability to learn according to continually changing landscapes within search algorithms allows for highly effective and responsive SEO, as we possess pre-trained LLMs.
⭐️Reinforcement Learning from Human Feedback (RLHF)
The idea of reinforcement learning from human feedback is that the approach will train the models using human feedback to improve relevance and page ranking through reinforcement of learning on what the end-user prefers through interaction in the interface. This then enhances the search engine’s ability to surface more pertinent content. This approach is intended to make search results much more accurate and relevant and ensures that the information provided to a user is precisely what that user needs. It makes the search experience much more fulfilling.
At ThatWare, we put into practice the use of RLHF to make the best SEO services for business. We collect user’s feedback regarding their interaction with our content and understand how they are inclined towards its usage, i.e., levels of preference and engagement. Real-time feedback allows us to change our strategies and maximize the content’s relevance. We always ensure that, based on user responses, our client’s websites are ranked highly in search results and best meet user needs. Hence, we keep a strong commitment to RLHF. We can bring exceptional value to our clients as we always improve their SEO tactics and ensure more traffic and engagement.
⭐️Inverse Document Frequency Weighting
This is called Inverse Document Frequency weighting, by which content can be ranked based on how rarely a term is used on the web. It helps in finding valuable keywords with less frequently used terms with greater emphasis on the terms, which would attract targeted traffic. Unique and niche keywords help in making search engines treat them as knowledge, unlike others. It makes the ranking since such a strategy creates more relevant content to search for particular queries than popular words.
On ThatWare, we adopt Inverse Document Frequency weighing to advance SEO strategies toward businesses. Using keyword frequency analysis across different platforms to determine rare yet invaluable terms that give a big difference in terms of the uniqueness of content brought out from our clients would include actual keyword research, the search for unique phrases one will like to bring out a message to his or her intended audience. This ensures good optimization of those keywords in all their content activities and optimization, after which more chances of increasing the rank in search results have been realized. Our methods enable more targeted traffic to result in high engagement conversion for clients’ websites by simply giving the user exactly what they came looking for.
⭐️Meta-Embedding Aggregation
Meta-embedding aggregation increases the search retrieval quality and relevance of multiple models for different embedding models. Integrating diverse models can make good use of other strengths. In this regard, full content representation would be attained for having a semantic understanding of the sense of queries or relevance concerning the documents involved. It captures more fine-grained meanings and relations within the data, hence ensuring that the information aggregated will be more relevant to users’ needs, which further leads to a more meaningful search and increased user satisfaction.
At ThatWare, we use meta-embedding aggregation to enhance our SEO services. Here, we aggregate the output of different embedding models into a unified representation to capture various semantic relationships from the content. This requires choosing the best-performing embedding models according to the client’s needs and industry trends. Such embeddings will allow the capacity of mapping to further improve between the query of relevance from the user to the content of the interest. The above strategy isn’t only used for a retrieval quality improvement in website terms but also assists targeted traffic, whereby the information source boosts the engagement and overall conversion rate at large.
⭐️Entity-Driven Relevance Scoring
This method scores content according to the relevance of identified entities and ensures that the content aligns better with specific topics of interest. This approach focuses on entities, which include people, places, and organizations. This approach enhances the search experience because the information found is contextually relevant and directly related to the query. This scoring mechanism will enable search engines to know the importance of entities in content, increasing the relevance of the matched intent and visibility of topics that matter most to users.
At ThatWare, we use entity-driven relevance scoring to ensure the best possible SEO strategy for businesses. We leverage advanced natural language processing techniques to identify the key entities in our client’s content and the industry. We can rank content better on topic-based relevance by analyzing these entities. This helps target optimization efforts to meet the user’s interest and search trends. Our clients get higher rankings, increased visibility, more targeted site traffic, and higher engagement with the content.
⭐️Temporal Information Embeddings
The time-based information embeddings incorporate temporal data to consider recency and history in a user’s query. The truth is that relevance changes with time for some pieces of information, so there ought to be an incorporation of time-related factors into the retrieval of content. By applying these embeddings, which include the trend in the current and history patterns, the search model will return the contextual correct answer at the right time. This approach will give users the data according to their direct needs and thus enrich the searching process, which is relevant content reflecting time dynamics.
We at ThatWare apply time information embeddings to strengthen SEO services for our client companies. We analyze the pattern by which users search over time, integrating time-based data into its content strategies to address current trends and historical relevance. This will periodically update our clients’ content regarding new events and use interactions with the previous ones to be relevant in those areas. By the temporal embeddings, we would draw closer to the ideal content for our clients through their user queries and enhancing the search rankings and the level of engagement. This helps the business and connects it with a better need for its audience.
⭐️Causal Inference in Information Retrieval
Causal inference in information retrieval pertains to how cause and effect relationships might be uncovered within data, thereby strengthening contextual relevance. Causal inference is much more advanced than correlation studies because, through this research, we discover why certain factors produce certain outcomes in search and user behavior. Thus, we could create more intuitive search and content strategies that address a user’s specific needs and motivations. Causal inference allows the algorithms to provide much more relevant information, all based on the causes that were detected behind the queries being made by the users so that it can find accurately and satisfactorily exactly what the user was searching for.
We make use of causal inference to improve our business SEO at ThatWare. It undergoes deep analyses of users’ data to discover cause-and-effect relationships behind the content in our strategy. By understanding what affects user behavior, we can create content that serves our users’ needs. Advanced analytics track the user’s behavior and alter our SEO strategies accordingly. Bringing causality to our process amplifies the relevance of our client’s content, thereby providing better search rankings and more engaging experiences for their audiences.
⭐️Discourse Analysis for Long-form Content
Discourse analysis for long texts is about interpreting complex discourse structures to improve content relevance in voluminous documents. It is the strategy of understanding how the individual sections of a document contribute to one another that will guarantee the coherence and intelligibility of a document, which improves relevance in long-tail content. The meaning and significance of the parts and components within long-length content enhance its relevance and utility for answering users’ inquiries. It improves search engine functionality to recognize the flow of information and its relevant hierarchy, thereby improving discovery and interaction. Finally, discourse analysis ensures that users derive both useful and coherent data. In essence, reading worthwhile text is meaningful.
At ThatWare, we help long-form content for clients via discourse analysis. Through us, the structure of large texts is analyzed to check and enhance every section within an individual document to coincide with a user’s desire in general while improving coherence in overall sections. Through themes and relationships, the creation of content relevant to the search engines but, above all, interesting for the reader is possible. With logical and intuitive rules regarding information organization, organizing longer forms of content is a breeze for the end users, thus giving higher-ranking searches and an improved user experience. With such a system, businesses can ultimately interact better with their target group.
⭐️Domain Adaptive Pre-Training for LLMs
One of the promising techniques to enhance the accuracy of information retrieval is the adaptation of large pre-trained language models via pre-training to specific domains such as health or finance. This technique adapts the pre-trained models to the domains under consideration and exposes them to domain-specific terminology, context, and nuances. Fine-tuning such LLMs for a specific domain would significantly improve the generation capabilities of such systems, thus increasing the relevance and accuracy of content generated according to the user’s query. This results in more effective engagement and satisfaction as users get more relevant information directly answering their needs in a particular context.
In ThatWare, we implement domain-adaptive pre-training for LLMs to improve our SEO services and suit different industries. Fine-tuning our models on industry-specific datasets allows the content we will produce to better resonate with the specific language and requirements of the industry. We tend to adapt and make decisions on the current circumstances through discussions with the proper subject matter experts who gather information that seems relevant to that context and share it. This makes our final product so precise and has perfect search engine placement. People are aptly engaged, thereby taking the business on a correct pedestal, improving the overall drive for target audiences, improving conversion opportunities, and handling industry niche issues.
⭐️Graph Neural Networks in Document Clustering
A particular kind of neural network called Graph Neural Networks (GNNs) was created to operate with graph data structures. GNNs use the connections and links between documents (as nodes) to improve the clustering process in document clustering.
👉 What are Graph Neural Networks?
- Graph Representation: Documents can be shown as nodes in a graph, with edges denoting connections between them (e.g., similarity based on co-occurrence of phrases, citations, or content).
- Node Features: Each document’s content can be represented by its related features, such as TF-IDF vectors or embeddings from pre-trained models like BERT.
👉 Why Use GNNs for Document Clustering?
- Identifying Relationships: Conventional clustering techniques like k-means frequently overlook the data’s related structure. GNNs can produce more relevant clusters by efficiently capturing document similarities and dependencies.
- Managing Irregular Structures: Documents usually don’t have a consistent structure; they can have intricate interactions. Because GNNs can handle such erratic data forms, they are a good fit for document grouping jobs.
- Better Feature Representation: By combining data from nearby nodes, GNNs can improve document representations and produce richer embeddings, leading to better clustering results.
At ThatWare, we use Graph Neural Networks (GNNs) to improve our information retrieval techniques and transform document clustering. Our group builds and examines the interconnections between documents using sophisticated graph-based methods, utilizing cutting-edge GNN architectures to provide insightful embeddings that reflect the complex relationships present in the data. We can find groups of related content with remarkably high accuracy by concentrating on the structural information in document relationships. For instance, we may swiftly arrange and classify data when we identify a collection of documents with similar themes or subjects, giving our clients the best insights and suggestions that suit their unique requirements. By ensuring consumers are shown the most pertinent information, this strategy improves content discoverability and increases engagement, reaffirming ThatWare’s dedication to providing innovative solutions in the digital space.
⭐️Open-Domain Question Answering (ODQA)
The goal of the artificial intelligence field known as “Open-Domain Question Answering” (ODQA) is to create systems that can respond to inquiries on almost any subject by utilizing a sizable amount of unstructured data, usually without regard to domain boundaries. Using extensive sources like Wikipedia, sizable document corpora, or the open web, ODQA seeks to answer queries on a wide range of subjects, unlike traditional question-answering systems, which are typically limited to a particular field of knowledge.
Retrieval and answer extraction are typically the two main steps in an ODQA system. A retrieval module first finds pertinent documents or sections from a sizable database (typically using search or dense retrieval processes) in response to a user’s query. The best accurate response is then found and displayed by an answer extraction module that examines the extracted sections.
Deep learning methods, including transformer-based language models like BERT, RoBERTa, and GPT, are used in recent ODQA developments. These models have allowed systems to attain remarkable accuracy through their comprehension of intricate linguistic patterns and contextual subtleties in data. To increase accuracy and better handle knowledge-intensive issues, graph-based methods and hybrid models combine structured databases with unstructured text.
ThatWare uses modern artificial intelligence (AI) technologies to enable robust Open-Domain Question Answering (ODQA) solutions. Our method builds systems that can respond to various queries on a broad range of subjects by fusing sophisticated retrieval processes with cutting-edge NLP models. Large language models and deep learning algorithms enable our ODQA solutions to provide precise, contextually appropriate responses from enormous unstructured text databases, such as knowledge repositories and public datasets. Businesses may obtain quick, insightful answers to complicated questions using ThatWare’s ODQA solutions, which improve decision-making and facilitate customer engagement by providing prompt, accurate information.
⭐️Saliency Mapping in Page Relevance
The technique known as saliency mapping in page relevance is used to draw attention to or “map” the most important elements of a webpage that help make it relevant for particular queries. Saliency mapping is useful in digital marketing, web development, and SEO because it makes it easier to determine which webpage components—text blocks, images, headings, and links—are most likely to affect the page’s ranking or relevance for specific keywords.
Saliency mapping often applies attention algorithms that allocate “saliency scores” to various page areas by analyzing its structure and content using machine learning and deep learning models. These scores indicate the relative significance of each component in establishing page relevance. In contrast to ancillary elements like sidebars or footers, which may obtain lower saliency scores, the heading, and main content regions may receive high saliency ratings if they contain terms closely related to a search query.
With this method, content producers and website developers can efficiently optimize their sites by concentrating on the components that most affect search engine results. Furthermore, by aligning page content with user expectations and behaviors, saliency mapping can be combined with heatmaps and eye-tracking research to enhance search relevancy and user experience.
At ThatWare, we improve page relevance for search engines and human intent by utilizing sophisticated saliency mapping algorithms. Our method ensures that high-relevance keywords and user-focused content are positioned strategically by identifying the most impactful features on a page, from core text blocks to subtle layout details. We give each section a saliency score by fusing deep learning models with attention mechanisms. This helps clients understand which parts of their pages have the greatest potential for ranking. By using this technique, we can improve layouts and rank content components according to user expectations and SEO algorithms, producing search engine-optimized sites that successfully connect with audiences.
⭐️Knowledge-Augmented LLMs
Knowledge-Augmented Large Language Models (LLMs) are sophisticated language models that incorporate information from real-time sources, databases, and knowledge graphs into their responses. Knowledge-augmented LLMs can obtain and update their responses based on up-to-date, domain-specific information, unlike regular LLMs, which only employ the data used during their initial training phase. Even when dealing with subjects not addressed in their training data or that call for extremely specialized expertise, these models can offer more accurate, current, and contextually relevant responses because of this integration.
These models function in practice by combining structured and unstructured knowledge sources with their natural language processing skills. To answer a challenging question or to confirm the information in their answers, individuals might, for instance, extract data from a knowledge network. Applications like healthcare, law, and scientific research, where accuracy and precision are essential, benefit greatly from this architecture. Knowledge-augmented LLMs are at the vanguard of improving AI’s adaptability to changes in real-world data, which will greatly increase its usefulness across various industries.
ThatWare specializes in using cutting-edge AI methods, such as Knowledge-Augmented Large Language Models (LLMs), to give our clients more accurate and contextually aware solutions. Our language models become more accurate and relevant by incorporating external knowledge sources, like real-time data feeds and domain-specific databases. With this strategy, we can provide state-of-the-art apps that support highly specialized industries where accuracy is crucial, such as the healthcare, financial, and legal sectors. At ThatWare, we’re dedicated to pushing the limits of artificial intelligence to develop models that are smart and adaptable to changing data environments and particular business requirements.
⭐️Sparse Attention Mechanisms
Attention mechanisms are a potent idea in neural networks, particularly in computer vision and natural language processing (NLP). Instead of giving each input data component the same weight, they enable models to concentrate on the most pertinent portions, like particular words in a sentence or areas of a picture. This is especially helpful for jobs like language translation or object recognition in photos when some components are more crucial than others.
Attention aids the model in focusing on particular words in an NLP context for tasks like translation, summarization, and sentiment analysis or for predicting the next word in a sentence. Attention methods, for instance, enable the model to concentrate on the English words most pertinent to the French term generated while translating a sentence from English to French.
The most widely used kind, known as “self-attention,” enables a sentence or other chain of words to relate to each other. The Transformer architecture, which has transformed the discipline by enabling highly parallelized and effective training, is built on this foundation. To record relationships between words independent of their position in the phrase, self-attention calculates a set of “attention weights” that determine the influence each word in a sentence has on others.
At ThatWare, we use cutting-edge technologies like attention mechanisms to improve our machine-learning models, especially in data analysis and natural language processing. Our team focuses on creating state-of-the-art solutions that maximize performance in activities like sentiment analysis, language translation, and content creation by utilizing attention-based architectures like Transformers. We guarantee that our models can dynamically focus on the most pertinent data by integrating attention mechanisms, which produces more accurate forecasts and insights.
We are constantly investigating and incorporating the most recent developments in artificial intelligence because of our dedication to innovation. ThatWare uses the power of attention processes to build intelligent systems that comprehend the context and adjust to changing user needs while focusing on providing outstanding value to our clients. With this strategy, we can offer customized solutions that enhance user experiences, optimize processes, and produce significant outcomes for various sectors. ThatWare is committed to pushing the limits of artificial intelligence and machine learning, improving consumer experiences, and increasing operational efficiencies.
⭐️Latent Dirichlet Allocation (LDA) for Topic Modeling
A popular generative probabilistic model for topic modeling in text data is Latent Dirichlet Allocation (LDA). It works especially well for revealing latent topic patterns in huge document collections. According to the basic tenet of LDA, each document is made up of various themes, and each topic is defined by a distribution across words.
👉LDA applications include:
- Text Classification: LDA can improve classification problems by giving algorithms more features by detecting document topics.
- Information Retrieval: LDA can enhance search engines by assisting them in comprehending the context of questions and documents.
- Recommender systems are useful for suggesting information based on user behavior-derived subjects of interest.
- Social Media Analysis: LDA can instantly discover recurring themes and attitudes by examining posts and comments.
👉Advantages of LDA Use:
- Unsupervised Learning: LDA is appropriate for exploratory data analysis since it doesn’t require labeled data.
- Interpretability: LDA frequently produces comprehensible and interpretable subjects, offering insights into the data’s underlying themes.
- Scalability: LDA is appropriate for big data applications since it can manage massive datasets.
Latent Dirichlet Allocation (LDA) is one of the cutting-edge machine learning approaches that ThatWare uses to improve the caliber and applicability of our content strategies. Our team of professionals is skilled in using LDA for topic modeling, which helps us find hidden themes and patterns in big datasets. Using this creative method, we may find popular subjects that appeal to viewers and create material that suits their interests and requirements. We know how important it is to stay ahead in the quickly changing digital market, and our dedication to using state-of-the-art technology guarantees that our clients get insights that can be put to use. Our proficiency in LDA and other machine learning models enables companies to produce powerful content that increases engagement and cultivates brand loyalty. At ThatWare, we’re not only interested in data but also in turning that data into solutions that will improve performance and visibility. Our team is available to help you at every stage, whether you’re trying to improve your content strategy or find new ways to engage your audience.
⭐️Knowledge Distillation for Model Efficiency
A model compression method called Knowledge Distillation seeks to increase machine learning models’ effectiveness without compromising functionality. The idea was presented to solve the problem of implementing big, intricate models—often called “teacher” models—that demand a lot of processing power. Knowledge distillation aims to transfer the knowledge acquired via the teacher model to a more compact and effective “student” model. Since these soft labels reflect the probabilities of the several classes the teacher model predicted, they provide more information than standard hard labels. These soft labels are then used to train the student model, which enables it to pick up on the teacher’s taught underlying patterns and decision boundaries.
👉Among the benefits of knowledge distillation are:
- Decreased Model Size: Due to its substantial size reduction, the student model is simpler to implement on gadgets with constrained processing power, including smartphones and edge devices.
- Faster Inference: Smaller models typically offer faster inference times for real-time applications.
- Maintained Accuracy: Although the student model is smaller, it still captures the necessary knowledge at performance levels that are on par with the instructor model.
- Improved Generalisation: Knowledge distillation can result in improved generalization as the student model learns from the teacher’s soft outputs, which contain more detailed information about the relationships in the classroom.
At ThatWare, we use cutting-edge artificial intelligence and machine learning techniques to provide innovative solutions that are customised to meet our clients’ demands. Our proficiency in several areas, such as knowledge distillation and model efficiency, enables us to develop optimized models that are incredibly effective and resource-efficient.
We know companies have difficulties implementing and managing AI technologies. For this reason, our team concentrates on cutting-edge strategies like knowledge distillation to enable our clients to take advantage of complex models without worrying about exorbitant computational expenses. Our simplified, effective AI solutions enable companies to reach their full potential using the fewest resources possible. Our dedication to remaining on the cutting edge of technology guarantees that our clients take advantage of the newest AI trends and techniques. ThatWare is here to help you at every stage, turning your idea into a reality with unmatched skill and commitment, whether you want to enhance your current models or investigate novel AI applications.
⭐️Page-Interaction Modeling
Page-interaction modeling analyzes how users interact with web pages in web analytics and user experience (UX). Organizations may learn more about user behavior, preferences, and pain areas by examining user interactions, including clicks, scrolls, and time spent on a page. This modeling eventually raises engagement and conversion rates by optimizing user paths, increasing content placement, and improving website design.
👉Important Elements of Modeling Page Interactions:
- Information Gathering: Data on user interactions is first gathered using heatmaps, click tracking, and session recordings. This data records numerous indicators, such as click-through rates, scroll depth, and navigation pathways.
- User Segmentation: User demographics or behavior patterns frequently divide interactions. This segmentation makes targeted enhancements possible and provides a deeper understanding of how various groups engage with the information.
- Behavioral Analysis: Businesses can examine trends in user interactions by utilizing statistical and machine learning methods. This study can determine which page parts are most interesting and which may be frustrating.
- Predictive Modelling: Using past data, sophisticated page-interaction modeling can forecast future user behavior. It can predict, for example, how page modifications may impact conversion rates or user engagement.
- A/B Testing: Organisations can test various designs or content layouts to determine which works best by using insights from page-interaction models to guide their A/B testing methods.
- Continuous Improvement: Page-interaction modeling necessitates constant study and iteration; it is not a one-time endeavor. Maintaining web page optimization for optimal user experience can be achieved by routinely updating models based on fresh user data.
Our specialty at ThatWare uses artificial intelligence and advanced analytics to improve user engagement and optimize digital experiences. Our method of modeling page interactions blends state-of-the-art technology with in-depth knowledge of user behavior, empowering companies to make informed decisions that improve their online visibility. To learn how users explore websites, where they click, and how long they stay engaged, we employ advanced methods to gather and analyze user interaction data. We assist our clients in improving their website designs and content strategies to better suit the tastes and requirements of their users by spotting trends in user behavior.
Furthermore, ThatWare’s knowledge goes beyond analysis. We work directly with our clients to implement customized solutions that improve user experiences, boost conversions, and propel overall company expansion. We constantly update our tactics based on the most recent data because we are dedicated to continuous improvement, which helps our clients stay ahead in the rapidly changing digital landscape.
ThatWare is your reliable companion in navigating the intricacies of digital user experience, whether your goal is to improve user engagement on your website or acquire a deeper understanding of user interactions.
⭐️Cohesion-Based Text Segmentation
Cohesion-Based Text Segmentation is a natural language processing (NLP) technique that separates a text into parts or coherent segments according to its cohesive features. This approach aims to find significant textual components, such as paragraphs or subjects, that enhance the overall comprehension of the subject.
👉Advantages
- Better Readability: By arranging material logically, cohesion-based segmentation makes publications easier to read.
- Contextual Understanding: This approach guarantees that segments maintain context by emphasizing coherent parts, facilitating users’ comprehension of the key concepts.
- Enhanced NLP activities: This segmentation technique can enhance the performance of several NLP activities, including text categorization, sentiment analysis, and summarization, by offering more lucid input.
To improve our natural language processing solutions, ThatWare uses advanced methods like Cohesion-Based Text Segmentation. Our team’s expertise in text analysis and segmentation into meaningful and cohesive pieces greatly enhances the caliber of our information retrieval and content analysis systems. We guarantee that our segmentation procedures preserve the context and meaning by concentrating on the coherent components of texts, producing outputs that are easier to understand and comprehend.
We use cutting-edge algorithms and machine learning techniques to maximize text segmentation and give our clients accurate insights and analytics. This method improves sentiment analysis and document summarising while increasing our NLP applications’ general efficacy. At ThatWare, we’re dedicated to providing our clients with cutting-edge solutions that leverage language’s power so they can explore and use their data more efficiently.
⭐️Semantic Drift Detection
The practice of detecting shifts in the context or meaning of data over time within a dataset or a particular domain is known as semantic drift detection. Semantic drift detection is a process where terms and concepts are understood differently when language and usage change. Numerous applications may be greatly impacted by this, particularly those that depend on information retrieval, machine learning, and natural language processing (NLP).
👉Key Aspects of Semantic Drift Detection:
- Dynamic Environments: The context in which data is generated might vary quickly in many real-world applications. For example, user preferences, industry jargon, and social media trends all change over time, requiring an adaptable approach to data analysis.
- Problems: Differentiating between real drift and noise in data, detecting it quickly, and ensuring systems adjust without losing previously learned information are some problems that semantic drift detection must overcome.
- Importance: Organisations may preserve the precision and applicability of their data-driven applications by successfully identifying and adjusting to semantic drift, which will ultimately improve user experience and decision-making procedures.
ThatWare is aware of how important Semantic Drift Detection is to preserving the precision and applicability of data-driven applications. Our group uses cutting-edge algorithms and natural language processing methods to track and detect changes in context and meaning across time. Our analytics framework’s integration of Semantic Drift Detection guarantees that our clients’ systems smoothly adjust to changing trends and terminology.
We employ advanced techniques like word embeddings and topic modeling to capture changes in data semantics. Thanks to this proactive approach, we can offer practical insights that guide content initiatives and improve user engagement. We assist our clients in staying ahead of the curve in their respective businesses by providing information regarding semantic shifts. We continuously improve our procedures to effectively and precisely detect semantic drift since we are dedicated to utilizing state-of-the-art technology. With ThatWare, you can be sure that your data analysis will continue to be significant and relevant, enabling your company to improve user experiences and make wise decisions in a constantly shifting environment.
⭐️Hybrid Recommender Systems in Search
Hybrid recommender systems use multiple recommendation approaches to increase the precision and pertinence of search results. These systems use the advantages of multiple strategies, including knowledge-based techniques, content-based filtering, and collaborative filtering, to offer users tailored suggestions based on their interests and actions.
👉Benefits of Hybrid Recommender Systems
- Enhanced Accuracy: By combining several approaches, hybrid systems can lessen each one’s drawbacks, producing more accurate recommendations.
- Personalization: These systems can increase user pleasure by customizing suggestions based on a thorough knowledge of user preferences.
- Diverse Recommendations: By presenting a greater range of recommendations, hybrid systems can avoid the “filter bubble” effect, which occurs when users are only exposed to a limited number of items.
At ThatWare, we deploy cutting-edge hybrid recommender systems to improve customer satisfaction and engagement across various platforms. To provide our clients with tailored and pertinent recommendations, we specialize in integrating collaborative filtering, content-based filtering, and knowledge-based techniques. We create customized solutions that enhance search results and offer various options to suit personal preferences by examining user behavior and preferences. Our team uses state-of-the-art algorithms and data analytics methodologies to guarantee that customers receive correct recommendations, eventually leading to improved conversion rates and client loyalty.
Our systems are made to adjust to users’ current needs and preferences since we recognize how crucial context is to providing useful recommendations. ThatWare is dedicated to improving the user experience through cutting-edge hybrid recommender systems that distinguish our clients in a crowded digital market, whether for e-commerce, streaming services, or content platforms.
⭐️Attention-Based Context Fusion
Attention-based context fusion is a method that improves the integration of contextual information from several sources or modalities. It is mostly utilized in computer vision and natural language processing (NLP). This approach uses attention processes, which are intended to increase models’ focus on pertinent elements while reducing the influence of unimportant ones.
👉Applications:
- Natural Language Processing: Attention-based context fusion aids models in comprehending the relationship between words and their context-based meanings in tasks such as machine translation, text summarization, and question answering.
- Multimodal Learning: This method can successfully combine textual and visual information to produce a cohesive comprehension of the subject in situations where both text and visuals are present (such as visual question responses).
- Speech Recognition: Eliminating background noise and concentrating on pertinent acoustic information can also improve speech recognition systems.
At ThatWare, we leverage cutting-edge technology, such as Attention-Based Context Fusion, to propel creative solutions that improve our clients’ digital experiences. Our team of professionals knows how important contextual information is for improving model performance, especially in domains like multimodal learning and natural language processing. By integrating attention processes, we optimize our models’ emphasis on pertinent data, guaranteeing that the most important traits are emphasized for increased efficiency and accuracy.
Whether improving search algorithms, creating strong recommendation systems, or refining content strategies, we are committed to offering customised solutions that satisfy the particular requirements of every customer. Our dedication to utilising advanced methods allows us to produce exceptional insights and results, enabling companies to prosper in a digital environment that is becoming increasingly competitive. By working with ThatWare, you’re not just selecting a service; you’re collaborating with a progressive group that places a high value on creativity and quality in each project.
⭐️Compositional Generalisation in Queries
The ability of a model or system to comprehend and interpret large questions created by combining simpler components or elements is known as a compositional generalization in inquiries. This idea is especially crucial in machine learning and natural language processing (NLP), where the objective is to allow models to generalize beyond the individual samples they have encountered during training.
👉Applications:
Compositional generalization is essential in many applications, such as chatbots, search engines, and voice assistants, where users commonly create new inquiries by mixing pre-existing terms. Improving this skill results in more accurate responses and better user experiences.
Our goal at ThatWare is to improve search intelligence using cutting-edge machine learning and natural language processing techniques. Our group concentrates on cutting-edge techniques that let computers attain compositional generalization, which enables a more thorough comprehension of user inquiries. We enable our clients to create solutions that can easily understand and react to complicated inquiries by utilizing advanced technologies, enhancing user engagement and experience. To guarantee that models can correctly interpret a broad range of queries, including original or unique ones, we stress the significance of training models on various datasets.
⭐️Zero-Shot and Few-Shot Learning in IR
Advanced machine learning paradigms called Zero-Shot and Few-Shot Learning in Information Retrieval (IR) tackle the difficulties of training models with little labeled data, which is frequently the case in IR problems.
👉Zero-Shot Learning
The capacity of a model to predict tasks for which it has not seen any labeled examples during training is known as zero-shot learning (ZSL). This implies that a system can efficiently retrieve pertinent documents for novel queries in the context of information retrieval without needing particular training samples for such queries. Usually, ZSL uses domain information contained in embeddings or knowledge transfer from related tasks. For example, a model that comprehends the connections between various concepts and categories can extrapolate this knowledge to previously undiscovered categories, enabling it to retrieve documents associated with such categories.
👉Few-Shot Learning
A comparable method called few-shot learning (FSL) focuses on teaching models to generalize from a relatively limited set of labeled examples. This can be particularly helpful in IR situations where gathering labeled data is costly or time-consuming. Based on a few samples, models can be taught to swiftly adjust to new queries or information requests using few-shot learning. Techniques like meta-learning, in which models learn to learn and adapt based on prior experiences, are frequently used to achieve this.
At ThatWare, we improve information retrieval systems by utilizing state-of-the-art machine learning approaches like zero-shot and few-shot learning. Our group focuses on creating cutting-edge solutions using little labeled data to get relevant search results. We guarantee that our systems can generalize efficiently by utilizing sophisticated algorithms and models, even in situations with few training examples. ThatWare is dedicated to providing game-changing solutions that satisfy the ever-changing demands of the modern digital environment.
⭐️Text-to-Text Transfer Transformer (T5)
Google Research created the robust and adaptable Text-to-Text Transfer Transformer (T5) model, which can handle a variety of natural language processing (NLP) tasks by transforming them into a text-to-text format. This implies that the model’s input and output are handled as text strings independent of the particular job.
👉Applications of T5:
- Machine translation is the process of translating text between languages.
- Writing succinct summaries of lengthy materials is known as text summarization.
- Answering questions: Producing responses from given text.
- Text classification is the process of grouping texts into pre-established groups.
At ThatWare, we use cutting-edge natural language processing models, such as the Text-to-Text Transfer Transformer (T5), to provide innovative AI solutions customized to our clients’ various demands. Our team of professionals focuses on using cutting-edge technologies to improve consumer interactions, streamline corporate processes, and provide creative results. We know that clear communication and data interpretation are essential in the current digital environment. We ensure our clients can smoothly incorporate AI into their operations by converting complex data into meaningful insights using models such as T5.
⭐️Attention Flow in Document Ranking
Attention flow in document ranking plays a crucial role in evaluating the relevance of various passages within a document. By tracking attention across sentences, systems can effectively identify the most pertinent information that addresses a user’s query. The process begins with receiving a query from a user’s client system and determining initial memory slots related to the query. This is followed by accessing a user-specific memory graph, which consists of nodes (representing episodic memories) and edges that illustrate relationships between these nodes.
In tThismework, ma employs machine-learning models to select candidate nodes based on the initial memory slots from the memory graph. The selected nodes are then used to generate a coherent response delivered to the user’s client system. This method ensures the response is irrelevant and textualized based on the user’s history and preferences.
👉How ThatWare Implements It into Their Hyper-Intelligence SEO Model
ThatWare integrates attention flow mechanisms into its HypeHyper-Intelligent strategies by leveraging user memory graphs to enhance content relevance and ranking. They employ advanced algorithms to analyze attention patterns across various content segments, ensuring that the most relevant passages are highlighted in search results. This targeted approach allows ThatWare to deliver tailored responses that effectively meet user queries; additionally, optimizing their memory graph models, ThatWare can improve the contextual understanding of content. This enables them to create SEO strategies that do not attract traffic and maintain user engagement through highly relevant and personalized search experiences. The result is a sophisticated SEO framework that maximizes visibility and enhances the overall user experience.
⭐️Interpretability in Ranking Models
Interpretability in ranking models is essential for clarifying the factors contributing to a page’s ranking. This aspect enhances user trust and improves usability by allowing users to understand why certain results are prioritized over others. Recent advancements in this area include the integration of global and local low-rank matrix approximations. This method leverages both broad patterns and localized preferences to enhance recommendation accuracy.
In particular, the global-local low-rank matrix approximation method combines a pre-trained global model with a localized approach, which helps unify hidden representations of users and items. However, challenges persist, including the need to adaptively determine the number of sub-matrices for capturing local information and to set variables ranked on user interactions. Fixed ranks can lead to incomplete representations and reduced predictive accuracy.
Moreover, the evidence suggesting non-random patterns in missing data has challenged the assumption that missing scores in collaborative filtering are random recognition. This indicates that a more nuanced approach to interpreting data can ignore ranking models by providing accurate and context-aware predictions.
👉How ThatWare Implements It
ThatWare leverages interpretability in its Hyper-Intelligent SEO strategies by employing advanced matrix approximation techniques to enhance its ranking models. They refine their algorithms by analyzing both user behavior and specific local interactions to offer clearer insights into ranking decisions. This ensures that clients receive explanations for rankings that are not understandable and actionable. By integrating user-specific and content-related data, ThatWare improves the relevance of its SEO solutions, leading to more effective content strategies and improved user engagement.
⭐️Polarity and Sentiment Embedding
Polarity and sentiment embedding techniques are crucial for enhancing the context and relevance of retrieved documents in information retrieval systems. These methods focus on analyzing the emotional tone of text data to improve the understanding of public sentiment, especially in long-form news articles. The analysis process typically involves several systematic steps.
Initially, text data is collected and cleaned to remove any noise, such as special characters. This cleaned dataset is then segmented into a training set that facilitates effective machine-learning training and evaluation. A deep learning network is constructed based on a representation model, utilizing pre-trained parameters to enhance the model’s performance. Following this, a specialized long text emotion polarity analysis network model is developed to assess the sentiment embedded in the text.
Training the model involves modifying the structure of the sample data and employing hierarchical sampling along with K-fold cross-validation. This ensures that the proportion of samples in each fold remains consistent with the original dataset, promoting robust model validation. The results from each fold are stored, with the highest-scoring model selected for the validation set. The test set is evaluated biennially by integrating the K-fold models and averaging the probabilities to produce the model’s sentiment predictions.
👉How ThatWare Implements It
ThatWare integrates polarity and sentiment embedding within its Hyper-Intelligent SEO strategies by utilizing advanced deep-learning techniques to analyze the sentiment of content. These methodologies refine document retrieval processes, ensuring that the most contextually relevant and sentimentally aligned content is provided. The approach enhances user engagement and satisfaction, as the content matches user queries and resonates emotionally. Through continuous sentiment analysis, ThatWare enables businesses to better understand audience sentiment, which informs content strategies and drives SEO performance.
⭐️Context-Aware Sentence Ranking
Context-aware sentence ranking is an advanced approach that enhances the relevance of sentences based on their surrounding context within long-form content. This methodology is particularly beneficial in managing contextual mobile searches, where the user’s environment and behavior significantly influence the search results.
A system designed for this purpose interprets user inputs by considering various types of “vitality information.” This may include the user’s current location, time of day, relevant calendar events, past behaviors, weather conditions, and even data from social networks. By integrating this contextual information, the system can tailor search results to provide more relevant and meaningful content that resonates with the user’s immediate circumstances.
For instance, when a mobile user initiates a search, the system assesses the contextual cues derived from the vitality information and formulates a context-based search request. This results in a more precise response that aligns with the user’s needs and preferences at that moment. Additionally, the system allows users to comment on the search results, fostering interaction and enriching the search experience by creating a sense of community among users.
👉How ThatWare Implements It
ThatWare incorporates context-aware sentence ranking in its Hyper-Intelligent SEO strategies by leveraging real-time user data to optimize content delivery. By analyzing various contextual signals, they ensure that the content shown to users is not only relevant but also timely and location-specific. This enhances user engagement, as visitors receive personalized content that meets their immediate needs and preferences.
Moreover, capturing user interactions, such as comments, allows ThatWare to refine its content strategies further. This continuous feedback loop helps improve search algorithms, ensuring they consistently receive the most pertinent and contextually relevant information. Through these practices, ThatWare positions itself to deliver enhanced user experiences, ultimately driving better engagement and conversion rates for businesses leveraging their SEO solutions.
⭐️Dynamic Search Intent Analysis
Dynamic search intent analysis is a sophisticated method that enhances user experience by identifying and adapting to evolving intents during user sessions. This technology recognizes that users’ needs and motivations change throughout their interactions, requiring a flexible approach to content delivery and relevance.
The core functionality of this system involves categorizing information based on user input and behavior. As users engage with the content, their thoughts and ideas are rapidly incorporated into a dynamic map, effectively aligning search goals with current user intents. This map serves various purposes, such as prior art searching, competitive analysis, and innovation gap identification. It transforms how users access and interact with information by creating a structured environment that promotes exploration and creativity.
By utilizing techniques such as fuzzy clustering and hierarchical self-organizing maps, dynamic search intent analysis ensures that information retrieval is not efficient and comprehensive. This allows for deeper insights into user needs, which traditional systems often fail to provide due to rigid categorization structures. The dynamic nature of this approach enables users to manage their search and categorization processes proactively, fostering innovation and collaboration.
How ThatWare Implements It
ThatWare integrates dynamic search intent analysis into its Hyper-Intelligence SEO strategies by continuously monitoring and adapting to user behavior throughout their sessions. This technology enables ThatWare to refine page relevance dynamically, ensuring that the content presented to users aligns closely with their evolving needs.
By employing this approach, ThatWare enhances the effectiveness of its SEO solutions, allowing businesses to respond to user intent in real time. The system captures data on user interactions, analyzes intent changes, and updates content accordingly. This improves user engagement and drives better conversion rates as users find the information they seek more efficiently.
Furthermore, by using advanced categorization techniques, ThatWare enhances navigability within large data sets, enabling users to explore sub-categories and related content seamlessly. This capability promotes a more interactive experience, ultimately leading to higher user satisfaction and loyalty. Through the strategic application of dynamic search intent analysis, ThatWare leads in providing intelligent and responsive SEO solutions.
⭐️Complex Query Decomposition
Complex query decomposition is a vital method in modern information retrieval systems that improves the processing and relevance ranking of intricate user queries. The approach involves breaking down a complex query into simpler, manageable parts, allowing for a more efficient search process. This technique particularly benefits systems that handle continuous data streams rather than traditional finite datasets.
In conventional database systems, queries are executed on static data using languages like SQL. Such queries produce finite results, limiting their effectiveness for applications that rely on continuous data or event streams. These applications, such as sensor data analysis and financial monitoring, generate data in real time, requiring a different querying mechanism that accommodates long-running queries over unbounded datasets.
To enhance performance, complex queries can be segmented into two primary functions: aggregate functions and global functions. Aggregate functions can be executed in parallel across multiple distributed nodes, improving scalability and efficiency. Once the aggregate results are computed, the global function consolidates these results, yielding a comprehensive output. This modular approach not only streamlines processing but also adapts to the dynamic nature of modern data sources.
👉How ThatWare Implements It
ThatWare leverages complex query decomposition within its Hyper-Intelligence SEO strategies to refine its data retrieval capabilities. By breaking down user queries, ThatWare can better understand search intent and context, leading to more relevant results. The ability to process continuous data allows for real-time analysis of user behavior and search trends, enhancing the overall effectiveness of SEO efforts. This method enables ThatWare to stay ahead of evolving search patterns and deliver optimized content that aligns with user expectations.
⭐️Self-Training for Information Retrieval
Self-training for information retrieval is a sophisticated approach that enables search models to evolve and enhance their performance over time based on user interactions and query results. This method employs deep neural networks (DNNs), including architectures such as retrieval-based, generative, and transformer models like BERT and GPT. The self-training process involves logically grouping or clustering training data to provide relevant context, which improves the model’s accuracy. For example, data can be organized by technology field, industry specialization, or types of writing genres.
The training begins by gathering user customization information, allowing the DNN to generate context-sensitive text suggestions. By determining which group or cluster best matches the user’s preferences, the model can be biased toward generating more accurate and relevant responses. This customization capability is not only applicable to text generation but also extends to chatbots and SEO optimization.
oThetem utilizes a large vocabulary and processes a corpus of documents to optimize long-text generation. By selecting a subset of documents related to specific queries, the system can generate text that aligns closely with user expectations. A histogram of tokens is used to normalize and merge probabilities, increasing the likelihood that the generated text reflects desired characteristics. Techniques such as pre-tokenization and custom encoding ensure that the model can efficiently process and generate large datasets.
👉How ThatWare Implements It
ThatWare integrates self-training for information retrieval into its Hyper-Intelligence SEO strategies to refine search and content generation processes. Using DNNs, ThatWare adapts its models to learn from user interactions and continuously improve search accuracy. The ability to customize responses based on user behavior enhances the relevance of generated content, ultimately driving better engagement and conversion rates. This self-training approach ensures ThatWare remains responsive to evolving user needs, allowing for a more dynamic and effective SEO strategy.
⭐️Time-Series Analysis for Search Trends
Time-series analysis for search trends is a critical technique to detect and prioritize emerging topics in the digital landscape. With the exponential growth of social media, which boasts over 483 million users as of 2009 and continues to expand at an annual rate of more than 47%, analyzing consumer sentiment on these platforms has become increasingly important. This analysis provides valuable insights into consumer opinions, brand perceptions, and market trends. By quantifying social media feedback, businesses can make informed decisions regarding advertising strategies, marketing campaigns, customer service improvements, brand management, product development, and investor relations.
The traditional methods of gathering and analyzing consumer feedback from social media can be cumbersome and resource-intensive, often requiring significant human intervention. While online content analytics tools can facilitate this process, they still fall short of providing real-time insights due to the manual effort involved in data collection and analysis. Time-series techniques can streamline this process by automatically analyzing patterns over time, thus enabling businesses to identify trending topics and shifts in consumer sentiment more efficiently.
Furthermore, integrating spatial information enhances the analytical capabilities of time-series analysis, allowing organizations to map consumer sentiment geographically. This functionality enables targeted advertising based on location and user behavior, thereby improving the effectiveness of marketing efforts.
👉How ThatWare Implements It
ThatWare leverages time-series analysis to refine its Hyper-Intelligence SEO strategies by continuously monitoring search trends and consumer sentiment across social media platforms. Using automated analytical tools, ThatWare can quickly identify emerging topics relevant to its clients, enabling timely content creation and optimization. The insights gained through time-series analysis allow ThatWare to adjust its strategies proactively, ensuring that its clients’ content aligns with current consumer interests and behaviors. This dynamic approach does not enhance brand visibility and improves engagement and conversion rates through targeted marketing efforts.
⭐️Dynamic Memory Networks for Query Relevance
Dynamic Memory Networks (DMNs) utilize memory mechanisms to enhance the relevance of queries across multiple sessions. This approach is particularly beneficial in search systems where maintaining contextual awareness is crucial for improving user experience and search accuracy. In a typical implementation, a visited website search system consists of a mini web server installed on a user’s local computer. The cois server receives search commands from a web browser following the HTTP protocol and generates relevant search results based on the user’s prior interactions with websites.
When a user performs a search, the system utilizes stored memory to reference previous queries and user behavior, which helps tailor search results to their specific context. This ensures that users receive the most relevant information based on their historical data, as the searcher component systematically searches for websites the user has visited previously, correlating the current query with that stored context. The web document generator then compiles the search results into a format suitable for display in the web browser, providing a seamless experience.
The ability to recall context from past sessions enhances the overall efficiency of the search process and helps users find information more quickly and accurately.
👉How ThatWare Implements It
ThatWare incorporates Dynamic Memory Networks into its Hyper-Intelligent SEO strategies to optimize search relevance and user engagement. By applying memory mechanisms, ThatWare ensures that systems can recall previous queries and user interactions, allowing for a more personalized and relevant search experience. This capability enables ThatWare to analyze patterns in user behavior and adjust content strategies accordingly.
As a result, clients benefit from improved content delivery that aligns with users’ needs and preferences over time. The application of DMNs enhances search relevance and helps ThatWare identify trends and optimize content for better visibility across search engines, ultimately leading to higher engagement rates and improved conversion outcomes.
⭐️Sparse Embedding Representations
Sparse embedding representations focus on essential features within data, streamlining model size while enhancing the relevance of outcomes. This method employs content modeling techniques to improve content production and editing processes. The automated system is designed to render content effectively by generating a content model for specific portions as they are being created.
As the content is processed, the system assesses the relevancy of the content model against a target content-derived model. The target model is established through several key steps: first, it identifies relevant portions of target content where a specific concept is articulated. Second, it generates associated target content models from each of these portions. Finally, the system conducts vector operations on these models to formulate a comprehensive target content model. This entire process heavily relies on AI-driven content analysis to ensure accuracy and depth in the generated models.
Once the relevancy score is established through this comparison, it can be utilized to inform content production recommendations. This approach increases the complexity and size of the models used. It prioritizes the most pertinent features, thereby improving the efficiency of content generation and ensuring that the final output aligns closely with user expectations and needs.
👉How ThatWare Implements It
ThatWare integrates sparse embedding representations into its Hyper-Intelligence SEO strategies to optimize content relevance and production efficiency. By utilizing AI-driven content modeling, ThatWare can quickly analyze existing content against target models, allowing for the generation of tailored content recommendations based on relevancy scores. This process enables identifying essential features that resonate with target audiences, ensuring that the content produced is concise and highly relevant.
Moreover, by focusing on the most critical aspects of content, ThatWare reduces model complexity, leading to improved processing speeds and lower resource consumption. This strategic implementation supports more effective SEO practices, helping clients achieve better visibility and engagement in search results while efficiently managing content workflows.
👉Advanced Topics in Ranking Algorithms, Information Retrieval, and Semantic Engineering
Advanced topics in ranking algorithms, information retrieval, and semantic engineering play a crucial role in enhancing the effectiveness and efficiency of content discovery. These areas focus on refining how information is indexed, retrieved, and presented to users, sequencing their search experiences and interactions with content.
As search engines become more sophisticated, understanding the intricacies of these advanced topics becomes essential for businesses seeking to improve their online visibility. Innovations in ranking algorithms enable search engines to better assess the relevance and quality of content, while advancements in information retrieval techniques streamline the process of finding pertinent information quickly. Meanwhile, semantic engineering allows for the interpretation of user intent, facilitating a more intuitive connection between search queries and content.
At the forefront of these advancements is ThatWare, which leverages its Hyper-Intelligence SEO strategies to implement cutting-edge ranking and information retrieval techniques. By integrating semantic understanding and advanced algorithms, ThatWare enhances the SEO performance of its clients, driving better traffic and engagement through intelligent content strategies. This comprehensive approach not only improves rankings but also improves the overall user experience.
⭐️Bidirectional Attention Mechanisms
Bidirectional attention mechanisms are pivotal in understanding user intent. They analyze the context of queries in both forward and backward directions. This approach enhances the accuracy of recommendation systems by effectively capturing the nuances of user and product features. The method involves several steps that streamline the process of generating relevant recommendations.
Initially, user and commodity features are extracted from training samples. These features are then transformed into embedded vectors serving as numerical representations. Both user and commodity-embedded vectors are input into an attention mechanism model for training. Through this model, the weight of each feature is learned via an attention network, which performs a weighted summation of all embedded vectors. This results in creating a user characterization vector and a commodity characterization vector.
To determine the alignment between user preferences and product offerings, the inner product of the user and commodity characterization vectors are calculated. This value indicates the degree of matching between the user’s purchasing intentions and the products. A cross-entropy loss function is established to minimize discrepancies in this matching degree, leading to the convergence of the attention mechanism model. Once trained, this model can process new samples to generate recall results based on predetermined matching intervals.
👉Implementation at ThatWare
ThatWare integrates bidirectional attention mechanisms into its Hyper-Intelligence SEO strategies to enhance the relevance of content recommendations. By applying this technology, ThatWare can analyze user queries more comprehensively, ensuring that content aligns closely with user intent. This approach improves user engagement and increases the effectiveness of SEO efforts by delivering highly relevant content based on the immediate context and historical interactions. As a result, clients benefit from optimized visibility and a stronger connection with their target audience.
⭐️Document-Level BERT Encoding
Document-level BERT encoding is an advanced method that leverages BERT (Bidirectional Encoder Representations from Transformers) to create a comprehensive understanding of content relevance across entire documents. This technique focuses on generating word embeddings in the document while accounting for their positions within the text. The process involves several key steps that aim to improve the representation of visual words and their relationships in the context of the entire document.
Initially, the words in a document are encoded to produce individual word embeddings. This includes identifying the relative positions of word pairs to generate position embeddings. Each word is transformed into a representation vector, combining pre-trained word embedding and associated position embeddings. The model utilizes contextualized embeddings, such as GloVe, and contextualized embeddings from BERT. When a word is divided into multiple sub-words or word pieces, the model averages the hidden vectors corresponding to those pieces to form a unified word embedding.
For position embeddings, the vectors are calculated based on the relative distances between the words and specific trigger and argument words identified in the document. This relationship is crucial as the model relies on these position embeddings to accurately represent each word’s contextual importance; the position embedding table is initialized randomly and refined during the training process, enhancing the model’s ability to understand the significance of word placement within the text.
👉Implementation at ThatWare
ThatWare employs document-level BERT encoding as a fundamental component of its Hyper-Intelligent SEO strategies. Applying this method, ThatWare enhances its ability to analyze content comprehensively, ensuring that search engines can evaluate the relevance of entire documents rather than just isolated keywords. This approach allows for a more nuanced understanding of user queries and content alignment, ultimately improving search visibility and engagement. Using BERT embeddings enables ThatWare to deliver highly relevant content tailored to user intent, positioning clients favorably in competitive online environments.
⭐️Poly-Encoder Networks
Poly-encoder networks represent an advanced architecture of residence information retrieval, particularly in scenarios with diverse query variations. This approach employs multiple embeddings to capture global contextual features, allowing for a more nuanced understanding of candidate responses. The architecture utilizes a hyperparameter 𝑛 to define the number of representations, enabling the model to effectively and to each candidate’s response; unlike single global vector representations, known as “encoders,” poly-encoders facilitate enhanced performance while remaining computationally efficient. They avoid the cross-encoderplexity of the input and output, which are simply concatenated. Instead, a Sequence-to-Sequence (Seq2Seq) Transformer architecture is employed to generate responses, leveraging pre-trained data with techniques such as Byte-Level BPE (Byte Pair Encoding) tokenization, as implemented in HuggingFace’s Tokenizers.
To prevent the generation of repetitive or generic responses, the poly-encoder first utilizes a retrieval model to draft a response based on the dialogue history. This draft is then integrated into the input sequence for the generator, separated by a distinct token. The generator can either produce a response based on this modified input or retrieve information from an extensive knowledge base. This retrieval process involves a TF-IDF-based inverted index lookup to identify potential knowledge candidates, which are then ranked using a Transformer retriever model. A classifier is also trained to determine when retrieval is necessary, distinguishing contexts that require additional knowledge from those that do not.
The domamodel’s domain-specific training allows it to grasp industry-specific terminology and jargon, ensuring relevant and precise responses without resorting to simplistic language or repetitive phrases.
👉Implementation at ThatWare
ThatWare integrates poly-encoder networks into its Hyper-Intelligence SEO strategies to enhance information retrieval and user engagement. Using this architecture, ThatWare ensures that its SEO solutions can effectively process varied user queries and generate contextually appropriate responses. Combining a retrieval model and a generator allows ThatWare to provide highly relevant content, tailoring responses based on user intent and contextual information. This capability enhances the effectiveness of ThatWare’s SEO strategies, positioning clients favorably in competitive search environments.
⭐️Contextualized Query Re-ranking
Contextualized query re-ranking enhances the relevance of search engine results by analyzing user intent, personalized search history, and session context. Keywords and phrases serve as the primary means users interact with search engines, influencing website traffic. EffeEffectively selecting keywords is crucial for website promoters aiming to optimize search engine visibility.
Techniques such as human evaluation of website content and order popularity tools are commonly employed to identify relevant keywords. These tools track how frequently specific keywords are searched, guiding promoters in selecting terms that generate significant search queries. Once a set of keywords is established, promoters may bid to achieve higher positions in search results, employing a pay-per-click model where bids determine the prominence of their listings.
Traditional clustering algorithms often group similar objects while assuming homogeneity among data types. However, this approach can lead to inefficiencies when applied to heterogeneous data, as relationships among different object types may remain underexplored. The high dimensionality of data generated from such clustering poses challenges, as it can render the data sparse and difficult to analyze effectively.
👉How ThatWare Implements It
At ThatWare, contextualized query re-ranking is integrated into their Hyper-Intelligence SEO strategies using data analytics and machine learning. The company employs sophisticated algorithms to analyze user behavior and intent, allowing them to rank keywords dynamically based on real-time search trends. By leveraging these insights, ThatWare can optimize content and improve website rankings effectively, ensuring that its clients’ online presence is maximized in a competitive digital landscape. This method enhances the accuracy of keyword selection and improves the overall efficiency of search engine optimization efforts.
⭐️Long-Document Transformers (e.g., Longformer)
Long-document transformers like su like former are designed to manage long-form content through sparse attention mechanisms. This capability makes them particularly suitable for content-heavy applications, including processing large datasets like patents. In general, a public patent dataset was utilized, whereas, in a typical workflow, patents were normalized to ensure equivalent total counts across various technology centers. This normalization involved random downsampling to align the least-represented tech center with others.
Data preparation involved tokenizing patents using the RoBERTa tokenizer and segmenting the data into labeled sequences, accommodating maximum input sizes for both RoBERTa and Longformer. However, Longformer was ultimately not employed due to GPU memory limitations and insufficient classification accuracy compared to RoBERTa.
Training procedures were optimized by leveraging parallel GPU training, utilizing mixed-precision (fp16) and gradient accumulation to achieve an effective batch size of 128. The training process was terminated once the evaluation F1 score ensured that the most accurate model was selected for predicting art unit assignments. Additionally, the SmoothGrad algorithm provided token-wise impact attribution for predictions, highlighting influential tokens in the final output.
👉Implementation in SEO Strategies at ThatWare
At ThatWare, the principles of long-document transformers are integrated into Hyper-Intelligence SEO strategies to enhance content analysis and optimization. Using these models, ThatWare can efficiently analyze extensive datasets, ensuring that key insights are derived from long-form content. This capability improves keyword targeting and content structuring, leading to better ranking performance in search engine results. The use of transformer models facilitates precise content categorization and enhances the overall effectiveness of SEO campaigns, aligning closely with ThatWare’s commitment to data-driven strategies.
⭐️Latent Semantic Analysis (LSA) for Relevance
Latent Semantic Analysis (LSA) is a technique in the area of natural language processing that allows one to extract more of the hidden meanings or the underlying relationships that can be found in words. It deals with the issue of analyzing the context under which the words are used while revealing patterns and connections of things that do not occur otherwise. The method improves semantic relevance matching, identifying synonyms, associated terms, and contextually similar phrases that can strongly enhance the content relevance for searching.
Therefore, in the world of SEO, LSA plays an important role in making sure that businesses have better content available for search engines to rank those businesses. Marketers can create more resounding content with algorithms as it also reflects user intent based on understanding language, which leads to improved engagement metrics and higher rankings of targeted keywords because it will resonate more with what a person is searching for.
At ThatWare, LSA is used in the SEO strategy multi-dimensionally. For example, we conduct in-depth keyword research where they identify the primary keywords alongside related terms and phrases the potential customers would search for. This allows them to create a more comprehensive keyword strategy that captures different aspects of user intent.
Then, we use LSA to measure the existing content so as to fill the gaps with more semantic relevance. So, we include relevant terms and synonyms in the content, which in turn deepens the content and gives it more relevance for better ranking in SERPs. Our team further utilize LSA for the optimization of on-page elements such as meta tags, headings, and alt text in a manner that those on-page elements are in agreement with the semantic themes of the content.
In real life, LSA can be observed in the tourism sector. If the word “beach vacations” is the targeted key phrase, a website offering travel can find “coastal getaways, tropical retreats,” or “oceanfront resorts” as the results of the LSA used. Such related terms will probably be used in content-including blog posts and services descriptions the site’s audience increases, increasing one’s possibilities of ranking to various searches that people execute on “beach vacation.”. This also increases relevance but gives depth and richness to the user experience, which in turn raises traffic and conversions.
⭐️Attention-Based Sentence Compression
It compresses the information into the crux of a sentence because this technique focuses on the most semantically dense words of a sentence. This helps retain key phrases and concepts that carry the message forward while omitting the least critical words for the prime message. In SEO terms, this improves content clarity and relevance, which search engines and readers find appealing as well.
ThatWare uses attention-based sentence compression in SEO practices. We optimize the content on the web, which makes it meaningful to the readers while keeping the information both engaging and relevant to them. We prioritize semantically rich words in order to come up with impactful and concise sentences, which boosts keyword relevance and improves rankings on search engines. Such an approach is very handy for meta descriptions, headings, and content aimed at quickly grasping user intent.
For example, consider the sentence: “The quick brown fox jumps over the lazy dog.” With an attention-based compression of the sentence, the outcome could be “quick fox jumps lazy dog,” in which the most important words were focused. The compressed sentence still delivered the main idea but in fewer words; therefore, it is much easier for search engines to identify keywords.
In practice, in optimizing a blog post for a client, We can look at long paragraphs and pick out the phrases that best resonate with their audience. With attention-based compression, the content becomes easy to read, improving user engagement and lowering bounce rates. This helps keep the readers interested and is well-aligned with search algorithms, which reward short, relevant content.
⭐️Knowledge Graph Embeddings for Entity Linking
We at ThatWare employ knowledge graph power in enhancing search algorithm entity linking. A representation of the entities involved in the interaction and those connecting them takes place by being mapped onto a higher dimensional vector space, with rankability and linkability emerging naturally as it allows to assessment of contextual relevance and semantics behind the given entities to identify the relevance between search objects. This enables improving results accuracy with better experiences.
We combine knowledge graph embeddings with high-performance algorithms for the advanced application of structured data found within knowledge graphs to make informed decisions for clients about search engine optimization. Our algorithm identifies entities critical to businesses and clients’ including products, services, and target audiences- embedding these in a way that algorithms will know their rank according to their context of relevance in queries in the search engines.
Our process involves the following steps:
- Data Extraction: We extract data from relevant knowledge graphs, such as Google Knowledge Graph, and other industry-specific databases.
- Embedding Creation: We convert this data into embeddings, capturing the relationships and attributes of each entity.
- Entity Linking: By analyzing user queries, our algorithms link these embeddings to provide accurate and contextually relevant search results.
A very actual example of such an implementation is demonstrated by the fact that ThatWare can aid a local restaurant in upgrading its online presence. It can be achieved with Knowledge Graph Embeddings; so, when some customer looks up relevant terms, like “Italian food near me” or “best pizza in [City],”, we can ensure that the restaurant our client owns will come first in the list of search results. Thus, the algorithm can sense a semantic relation between the restaurant, the type of food offered there, and the location, thereby linking the restaurant entity to the user’s search intent effectively. It results in improved visibility, increased click-through rates, and, ultimately, foot traffic into the restaurant.
⭐️Sentence Transformers for Similarity Matching
Sentence transformers are very strong tools in the domain of NLP as they map the sentences to high-dimensional embeddings, where the similarities between two different sentences can be seen through these embeddings. They, unlike word embeddings which put words into vector space, give out embeddings of whole sentences. This approach manages to capture much subtler meanings as it captures contextual and relation-based relations inside the words of the sentence.
ThatWare makes its content relevant to users through the application of sentence transformers, thereby enhancing the SEO techniques. When using optimisation techniques for web content, the material used needs to contain the relevant keywords, as well as fulfilling the intent behind them. Sentence transformers help in analyzing semantic similarity between user search queries and already existing content. We can use this embedding to search for the gaps in content or where existing content may potentially be more aligned with the intent of users.
For example, if the user searches with the keyword “best practices for content marketing,” ThatWare can use sentence transformers to compare this search query to the existing content on the client’s site. Where the embeddings provide a very low similarity score, that would mean that the contents might not appropriately address what the user needs. From this, we create targeted, quality content aligning with users’ searches, thereby bringing about enhanced ranking and presence of the site in results.
⭐️Cross-Lingual Embeddings
Cross-lingual embeddings describe the representation of words or phrases from different languages in a shared vector space, which enables more effective multilingual understanding and retrieval. The embedding of multilingual data into a unified space improves cross-language retrieval tasks by enabling more accurate results. The method relies on the semantic relationships between words across languages, which can better match queries and documents that are not in the same language but convey the same meaning.
Cross-lingual embeddings can significantly improve the discoverability of content across multiple languages if applied in SEO. For example, ThatWare is a leading provider of innovative SEO strategies that use cross-lingual embeddings to optimize content. We therefore ensure the websites of clients can rank correctly in various regions by providing multilingual keyword research coupled with semantically aligned content for different languages.
For example, in the scenario where a business sells its products in an English-speaking country, then Spanish. We intervene in the sense of using cross-lingual embeddings to seek keywords that exist within the same language to determine which of the English keywords exist or have corresponding meaning within the Spanish language, then used while embedding the former into one vector space in the sense of creating content appealing to the language speakers while maintaining the meaning for the searches made.
One real example of cross-lingual embeddings in real life can be seen on Google Search. If an individual performs a search query in Spanish for a particular product but the website containing the desired information is only available in English, cross-lingual embeddings could be applied by Google algorithms to get the appropriate relevant English pages that satisfy the intent behind the search in Spanish. This would lead to greater reach for the business, and user experience will also improve due to relevant results no matter the language used.
By using cross-lingual embeddings, we enhance SEO performance and drive global engagement for our clients.
⭐️Predictive Intent Recognition
Predictive intent recognition is an analysis of current and historical user query behaviour to predict what a user will search for or how a user will engage with content in the future. It applies data analytics and machine learning algorithms to look for patterns and trends within user behaviour. Once understand the intent behind a user’s query, businesses are better equipped to shape content and marketing strategies that would serve user needs, increasing engagement and conversion rates.
ThatWare uses predictive intent recognition in its SEO tactics. Advanced analytics tools and algorithms are used to assess the search patterns of users. From data obtained from sources such as search engines, social media, and website analytics, we can determine trends in user behaviour. We can, for instance, find the most frequently searched terms, common questions, and preferred content. Such knowledge will enable us to optimize the existing content and create new content that will align with the anticipated interests and needs of the users.
E-commerce sites, such as Amazon, provide one of the good examples of recognition in the real world through predictive intent. Here, the user’s purchase history, browsing behaviour, and reviews of the product enable Amazon to suggest what products may be of interest to its users. The user experience will be enhanced, as well as the sales volume. For instance, a customer who purchases outdoor equipment on average frequently will trigger the prediction by Amazon on hiking equipment; thus, such items are recommended. It will lead to enhanced conversion rates and customer satisfaction.
A very important SEO tool, predictive intent recognition enables companies such as ThatWare to foresee what users are looking for and to deliver content targeted at these needs, thereby improving online visibility and engagement.
⭐️Content Generation Detection Algorithms
Content generation detection algorithms are made to check whether the content is original, AI-generated, or a copy. It analyses sentence formation, complexity, vocabulary, and semantic patterns to mark the content as AI-generated or plagiarized. Search engines like Google are capable of detecting the source of the content. This is what leads to unique, quality material, which boosts the user experience and their confidence in it. For SEO, these are very important algorithms because they have a direct impact on rank. Original content ranks while AI-generated or copied gets penalized, which subsequently lowers the visibility of such a site.
ThatWare applies content detection with the use of algorithms to keep things authentic and ranking as unique and high-quality materials. Through AI and Machine Learning, we develop algorithms to analyze content before publishing. These algorithms tag text that looks like often-spotted AI-generation patterns or even matches existing content on the web, meaning that what goes out is highly original and relevant to the context. Another thing we do is implement LSI (Latent Semantic Indexing) and NLP (Natural Language Processing) to create keyword-enriched content that naturally reads but is also good enough for search engines.
In addition to detection, we have continuous monitoring as part of its approach. Scanning a site’s content regularly ensures any unintentional duplication or AI-generated fragments are detected and improved to preserve the authenticity of the content and reputation of the site.
For a recent e-commerce campaign, ThatWare found duplicate product descriptions that were dragging rankings down. We identified the duplicate parts within their contents and made their team rewrite those descriptions in an authentic voice to bring out unique selling points. Within two months, organic traffic increased by 30 per cent with higher ranks on the results page and this was due to freshness that was brought into the content through proactive detection and optimization by ThatWare.
⭐️Hierarchical Embedding Models
Very complex, advanced method of embedding text. Processes and analyses data across multiple levels, the granularity ranging from paragraph up to sentence to word level, whereas the traditional embedding either works on words or even sentences in isolation, though HEMs combine layer upon layer of context emanating from different parts of the document for a granularity of language. This multi-layered approach captures the complex interplay of words, sentences, and paragraphs, making it more helpful for information retrieval applications, such as sentiment analysis and a variety of NLP tasks. In the field of SEO, the precision of information retrieval in the analysis of long-form content with variable themes increases because of hierarchical embeddings.
In this respect, ThatWare applies Hierarchical Embedding Models to its SEO strategies such that each piece of content is thoroughly optimized for subtle search queries. In this way, we can break down how a particular sentence or even word may affect the general theme of an article or webpage by embedding it at multiple levels. This way, the tool will highlight and take advantage of significant keywords that match user intent, all while relevant information is being provided coherently and contextually. This is how we enhance a site’s position in the search engine rankings, attracting more query types and producing content that directly responds to users’ needs. For instance, consider an article about “sustainable fashion” and optimize it with SEO keywords.
This helps us realize the interdependency of terms like “eco-friendly materials” at the word level, “affordable green options” at the sentence level, and “the long-term impact of sustainable practices on the environment” at the paragraph level through the usage of HEMs. Such subtle structure allows search engines to recognize the article as a high relevance for users with various interests in sustainable fashion-its affordability, its ecological impacts, and so This helps improve its ranking over different search queries. Implementation of hierarchical embedding in our SEO strategies will therefore ensure that the content ranks higher but also communicates more effectively with the reader.
⭐️Semantic Memory Networks
Semantic Memory Networks are frameworks that enable systems to retain and utilize relevant semantic information across multiple queries. This approach refines responses by identifying patterns and context from past interactions, allowing future answers to be more accurate and contextually aligned. Unlike traditional keyword-based methods, semantic memory focuses on understanding the deeper relationships between concepts, ensuring that the response aligns with user intent. In SEO, Semantic Memory Networks help refine content, keyword targeting, and user engagement strategies based on past interactions, leading to more precise and meaningful search results over time.
ThatWare, a leader in AI-driven SEO solutions, uses Semantic Memory Networks to enhance its strategies and create adaptive content that aligns with evolving user needs. By implementing machine learning algorithms and Natural Language Processing (NLP), our systems can “remember” previous interactions and refine future recommendations. This is especially useful for complex, multi-step customer journeys where user intent may vary across interactions. With Semantic Memory Networks, our SEO strategies dynamically adapt to user behaviour, refining keyword relevance and delivering content that closely matches user queries and preferences. This strategic advantage allows for continuously optimized search experiences, meeting Google’s latest SEO criteria for relevance and user satisfaction.
For example, consider a user interested in purchasing a home gym setup. Our Semantic Memory Network tracks and retains data on the user’s previous interactions, such as “best home gym equipment,” “home workout routines,” and “budget home gym ideas.” Each query provides valuable semantic information, and our system can refine its responses to suggest relevant products, compare equipment, or offer content on affordable setups. By retaining context from prior searches, our system improves the personalization and relevance of search results, effectively guiding the user closer to a decision.
This approach not only enhances user satisfaction but also strengthens ThatWare’s client results by maintaining relevancy and building content that evolves with user intent.
⭐️Positional Encoding in Ranking
Positional encoding is a search engine optimization technique. The point is that a search algorithm will take into consideration text snippets’ positions in any given document or webpage to judge its pertinence. In Natural Language Processing, it highlights the sequence of words because the search engines want to understand the word’s relative value. Applied to SEO, positional encoding helps the search engine prioritize text in crucial positions, such as headings, introductions, or meta descriptions. In this way, search engines can rank pages more effectively according to user intent and provide more accurate and meaningful results.
ThatWare is an innovative SEO service provider that uses positional encoding in its SEO strategies to enhance content relevancy and ranking. We use keyword placement in headlines, subheadlines, and within the page content’s first 100 words. Our team also utilize structured internal linking and anchor text in strategic places throughout to indicate the importance of given sections while being both user-friendly and search engine-friendly. We apply the usage of highly advanced AI and machine learning technologies to check on the page layout, to try to guess where best a given set of keywords or phrases may fit within a given page layout in a particular version, according to a certain set of search algorithms. Through the mapping of relevance between content and a particular location, we assists its clients in improving the ranking for their competitive keywords.
A direct practical use case for positional encoding in SEO can be applied to an e-commerce site. Suppose ThatWare is also optimizing a product page for the clothing retailer targeting the keyword “men’s leather jacket.” Placing this keyword in the page’s title, the product description introduction, and a subheading under “Features,” makes sure that the search engine detects it as highly relevant. They also position similar keywords like “genuine leather” and “winter collection” strategically in such primary positions. This way of keyword placement makes the pages more visible for related searches, thereby helping retailers rank better and reach the right audience.
⭐️Entity Disambiguation in Search Queries
Entity disambiguation with search queries answers the ambiguity of a search term that may refer to several meanings, people, or locations. Understanding the background, the search engine tries to return results based on the intent of the users. For example, for the search term “apple,” the search engine has a choice of deciding whether you are referring to the fruit or the company of Apple technology. Even more disambiguation help might come from the context of the query, previous searches, or even a specific location, thereby making results relevant to what the user might be expecting.
The process of entity disambiguation in ThatWare SEO techniques uses advanced semantic engineering. The structured data and schema on websites implemented by us allow search engines to know the details of the entity, such as the brand, location, or industry information. This helps to associate queries more precisely with the intended meaning of each search term. Besides, we use AI and machine learning methods like clustering and co-occurrence analysis to identify patterns and relationships between entities. Such a strategy helps in recognizing entities in search results more effectively, which is quite helpful for clients in competitive or ambiguous fields.
An example of the use of ThatWare’s entity disambiguation in the real world is legal services search. When a client specializes in “civil law,” we optimize content so that search engines understand the specific legal service focus, differentiating it from other topics, such as civil engineering. Through semantic engineering that clarifies the entity of “civil law,” ThatWare improves visibility for users who specifically seek legal support in civil law, thus helping the client rank more effectively in relevant search results.
It helps increase precision within results delivered by search engines, reduces ambiguity, and consequently increases user engagement through the delivery of contextually accurate content to users.
⭐️Contextualized Language Representations
Contextualized language representations are a sophisticated method of word embedding within the context they are used in, thus enabling algorithms to understand and process subtle meanings that depend on context. Unlike traditional word embeddings, which treat words as static entities with one meaning, contextualized language models, such as BERT and GPT, change word meanings dynamically according to the structure of the sentence and the words surrounding them. This allows for deep language understanding and is, therefore, critical in NLP applications for SEO in which contextual understanding is vital in interpreting user intent.
SEO strategies at ThatWare, therefore, adopt contextualized language representations to achieve high relevance and intent-driven content. We can analyze subtle differences in user queries through models that can generate context-sensitive word embeddings and hence react to them. For example, a query such as “Apple store near me” could mean a technological shop or even a fruit vendor in certain regions, based on the location of the user and their previous engagement. With contextual understanding, our approach would be able to differentiate such intents and respond or send content accordingly to exactly what the user is looking for. This means that there is optimized, highly relevant content that will boost rank and be per the user’s intent during the search.
Let’s say you wanted a webpage to rank on “organic apple juice” and “Apple store deals.” Although both phrases happen to contain the word “apple,” their meanings are pretty dissimilar. ThatWare works with contextualized language models to analyze content relevance based on each keyword. It will optimize for content related to health advantages, organic certifications, and nutrients for “organic apple juice.” For “Apple store deals,” it will go for technology products, special sales events, and close-by Apple retail stores. Contextual differentiation in that sense makes the SEO approach of ThatWare more appropriate and user-friendly; the visitor gets the most relevant information following his intent. This, therefore means better engagement rates, higher conversion rates, and good ranking in search engines that depict the effects of contextualized language representations in our SEO practices.
⭐️Real-time Query Rewriting Algorithms
Real-time query rewriting algorithms modify complex or vague queries in search time to much more lucid or relevant phrases for searching. These algorithms critically enhance how well the search engine finds a match for a person’s intent regarding what has been searched based on how likely a person is thinking and possibly wanting to prefer and is currently searching with their trends. Thus it predicts what exact things people want to discover, which is why, therefore, the search engines bring exactly those results into the market that are worthwhile for businesses to connect people with the right crowds.
At ThatWare, real-time query rewriting is part of the SEO strategy. Our approach makes use of advanced AI tools and NLP techniques to interpret user intent correctly. For instance, if a user’s search query is too broad or unclear, our algorithms identify synonymous terms, relevant keywords, and context so that they can rewrite it into a clearer query targeting a more specific intent. This process allows us to perfect keywords and content strategies for clients’ sites so they align more closely with what the user might look for in real-time.
For example, a user may be searching for “best restaurant experience.” That is a very broad term. That may mean the quality of dining, the ambience, or a specific kind of cuisine. Our query rewriting algorithm determines intent by searching similar previous queries, and the location and preferences of users. Maybe it will write the same query as “top-rated Italian restaurants with live music” if this better caters to most users for the “restaurant experience” search request. This kind of better-targeted query rewrite can likely generate more targeted results; therefore, these will comply with the intended meaning that the user looks for but also with the ranking metrics of the search engine.
Using real-time query rewriting, ThatWare enables its clients to tap into a much more focused audience, increasing user engagement and driving conversions. This means that clients will benefit from increased visibility, better click-through rates, and an SEO strategy finely tuned to changing search behaviours. Ultimately, this fine-tunes ThatWare’s SEO effectiveness to each user’s unique intent in real time.
⭐️Salience-Based Relevance Modeling
Salience-Based Relevance Modeling uses a strategic approach of the most relevant and important items in the content by dint of the relevance of topic or query. Instead of placing equal weight on all these items of the content, Salience is used to prioritize importance or prominence on such parts of the content concerning which alignment towards user queries and search intention is most direct. This method has improved how search engines understand and rank content, making the most relevant information prominent, thereby increasing visibility in search results.
ThatWare utilizes salience-based modelling as part of its SEO campaigns by targeting content elements showing high query relevance. We identify keywords and topics highly associated with a user’s query and optimize the content sections so that the most salient parts of the content stand out. For instance, it uses AI-driven tools to analyze the structure and hierarchy of the content so that the primary keywords and topics are placed most obviously in titles, headers, and summary sections. This means that we further refine the content with semantic analysis, which will allow it to better match the algorithms by Google on topical relevance and user intent. By doing so, ThatWare can get content indexed better by search engines while ensuring maximum value for the reader as it will deal with the primary concerns or interests at the forefront.
For example, in the case of e-commerce, on the product page for the “wireless noise-cancelling headphone,” salience-based relevance modelling will rank the order of importance of product specs, user benefits, and FAQs regarding noise cancellation at the top of the page. ThatWare’s approach will ensure that the search engine indexes the most relevant sections of the page first. Increasing the visibility of pages to queries such as “best wireless noise-cancelling headphones.” This will make the page appeal more to both parties: the search engine and the customer; increasing engagements and conversion rates.
⭐️Relation Extraction for Query Matching
Relation Extraction for Query Matching is the technique in SEO and information retrieval to find and interpret the relationships of entities inside a query. When different entities, like brands, locations, or actions, are related to one another, search engines and AI systems can better understand the user’s intent, which results in more accurate and relevant answers. This method benefits SEO by aligning deep or complex user queries with suitable content to satisfy search intent.
This includes the extraction of relations in SEO strategies. Here, the search engine refines interpreting the query by finding whether the type of relation is between two entities in a query, for example, “best Italian restaurants near Central Park,” which primarily will be “interest” or “Italian restaurants,” while “Central Park” will be “location”. This can bring back very accurate results even for the faintest and multi-entity questions also as the search engine identifies not just entities but relations between entities as well.
The more advanced SEO techniques of ThatWare apply relation extraction to achieve better content targeting. We apply AI-powered NLP algorithms that recognize the relationship between entities in a user’s search query to ensure businesses feature in the appropriate results for that query. For instance, we apply relation extraction in the analysis of user search behaviour and how to structure website content so that it follows the intent of the user as well as Google’s interpretation of complex queries.
A simple relation extraction example could be as follows: a search term “best affordable coworking spaces near San Francisco for startups.” Here, relation extraction picks up relationships among the phrases “affordable coworking spaces,” “San Francisco,” and “startups.” ThatWare will apply these findings to help a coworking space brand work its content for optimal exposure over the search terms regarding price, location, and enterprise type (startups).
⭐️Commonsense Reasoning in IR
It is essential to develop information retrieval (IR) systems that understand the context and intent behind user queries. ThatWare utilizes advanced machine learning techniques to integrate commonsense knowledge into our IR models, enabling the creation of systems that interpret queries more effectively.
This capability allows us to deliver results that not only meet keyword criteria but also align with user intent and hidden needs. For instance, when a user types “best places to eat,” our system can analyze contextual clues to determine whether the user is looking for casual eateries or fine dining experiences. As a result, users receive more relevant options for their preferences to increase customer satisfaction.
Furthermore, leveraging commonsense reasoning reduces the reliance on exact phrase matches. This approach helps us capture the nuances of natural language more successfully. It also aids in understanding ambiguous terms and various expressions of the same concept, thereby expanding the range of relevant results provided.
Continuous refinement of our commonsense reasoning models through user feedback and ongoing training ensures that ThatWare’s clients’ content ranks higher in search engine results while effectively addressing the actual needs of their audience.
⭐️Named Entity Recognition (NER) Enhanced Ranking
Named Entity Recognition (NER) is a crucial component of modern SEO strategies, as it allows for the identification and categorization of key entities within a text, including people, organizations, locations, and events. At ThatWare, we employ advanced NER techniques to enhance content ranking, ensuring it aligns with the queries users are searching for.
We utilize sophisticated algorithms that extract and classify entities from large volumes of text. This capability allows us to gain a better understanding of context and relevance, enabling us to optimize content more effectively for search engines. For example, when a user searches for “restaurants in New York,” our NER system identifies and highlights relevant entities in our clients’ content, such as specific restaurant names, types of cuisine, and locations. This prioritization helps these elements stand out in search results.
To further enhance user experience, we apply contextual NER, which analyzes the relationships among entities within the content. This means the information provided is not only accurate but also meaningful, as it reflects the connections between different entities. By promoting well-defined entities, we assist our clients in achieving better rankings in search results and driving more relevant traffic to their websites.
At ThatWare, we are committed to continuously improving our NER capabilities to ensure our strategies remain effective and adapt to the evolving SEO landscape. By focusing on NER-enhanced ranking algorithms, we provide our clients with optimal visibility and engagement.
⭐️Contextual Thesaurus Expansion
Contextual thesaurus expansion is an innovative approach to keyword optimization that enhances the relevance and variety of search terms associated with clients’ content. This method goes beyond traditional thesaurus techniques, which often provide synonyms in isolation. Instead, our approach considers the specific context in which words are used, allowing us to deliver a tailored vocabulary that aligns closely with user intent.
At ThatWare, we employ advanced natural language processing techniques to analyze the semantic relationships between words and phrases. This enables us to create a dynamic list of keywords and phrases that resonate with our target audience and reflect their search behaviors. For instance, if a client’s website focuses on “digital marketing,” our contextual thesaurus expansion can suggest related terms such as “SEO strategies,” “social media advertising,” and “content marketing techniques.” This broadens the content’s reach and relevance.
Additionally, we optimize content to incorporate long-tail keywords, which are particularly effective for capturing specific search queries. By integrating these expanded terms naturally into the content, we improve the likelihood of ranking for a wider range of relevant searches.
Through ongoing analysis and adjustments, ThatWare ensures that our clients’ content remains relevant in a constantly evolving digital landscape. By adopting this advanced keyword optimization strategy, we help clients attract more organic traffic and improve their overall visibility on search engines.
⭐️User-Centric Entity Resolution
User-centric entity resolution is a methodology designed to enhance information retrieval accuracy based on the specific needs and preferences of users. By understanding user behavior and contextualizing data, ThatWare ensures that content resonates with our client’s target audiences.
This approach involves identifying and disambiguating entities in user queries to connect users with the most relevant information. For example, when a user searches for “Apple,” our entity resolution system can discern whether they are referring to the technology company or the fruit, as it leverages contextual cues from the user’s search history and the phrasing of their query. This precision is crucial for enhancing user satisfaction and engagement.
Additionally, we continually refine our entity resolution processes through feedback mechanisms. By analyzing user interactions with search results, we can evaluate the effectiveness of our entity resolution methods in practice. This process involves making real-time adaptations and improvements to our strategies, ensuring they align with user expectations.
The concept of user-centric entity resolution focuses on understanding the specific entities that users seek while navigating our clients’ content. ThatWare enhances SEO processes to maintain a strong focus on delivering relevant information to its users, ultimately increasing conversion rates.
⭐️Multi-Passage Ranking Models
Multi-passage ranking models represent one of the latest trends in information retrieval, significantly improving search engine efficiency. At ThatWare, we use these models to analyze and rank multiple text passages simultaneously, providing users with the most accurate and comprehensive information from diverse sources.
This technique recognizes that a well-rounded answer often requires synthesizing information from multiple passages rather than relying on a single source. By leveraging multi-passage ranking, we can gather and score relevant snippets from various content pieces, offering users a complete understanding of the subject matter. For instance, when a user searches for “benefits of meditation,” our model aggregates insights from numerous articles, research studies, and expert opinions to deliver a holistic answer.
Our multi-passage ranking models are sophisticated, as they weigh both the relevance and quality of each passage to ensure that the most informative, authoritative content appears at the top of search results. This approach enhances the user experience and fosters greater engagement by providing comprehensive and nuanced answers tailored to users’ queries.
At ThatWare, we continuously improve our multi-passage ranking capabilities to adapt to the evolving landscape of search engine algorithms. By embracing this advanced approach, we empower our clients to achieve superior visibility and user engagement, positioning them as leaders in their respective industries.
⭐️Dense Phrase Embeddings
ThatWare uses dense phrase embeddings to achieve superior search results by closely aligning with user intent, surpassing traditional keyword-centric approaches. Dense embeddings work by mapping phrases into a multi-dimensional vector space, which enables the identification of semantic similarities even when phrases are worded differently. By encoding nuanced meanings, this approach allows for more efficient analysis and interpretation of content relevance to user queries.
With this technique, ThatWare can optimize content not only for specific keywords but also for broader, related concepts, making SEO strategies more resilient to variations in search language. Dense phrase embeddings also support a more advanced approach to keyword grouping and clustering. In this vector space, keywords are represented as points, allowing ThatWare to identify clusters of related terms and organize content to comprehensively cover entire themes or topics.
Instead of designing a page focused on a single, narrow keyword, ThatWare can create content that ranks for a wide range of related searches to enhance overall search visibility. This strategy bridges the gap between what users might be looking for and the content presented, ensuring optimal results at both a high level of visibility and detailed search precision.
⭐️Contextual Knowledge Graph Expansion
ThatWare’s Contextual Knowledge Graph Expansion method builds authoritative connections between related topics and entities to establish a robust framework for content relevance and search engine authority. A knowledge graph is a structured information network that visually displays relationships between various entities, showcasing how different pieces of information are interconnected.
Through the continuous, contextually relevant expansion of this knowledge graph, ThatWare creates a rich web of content recognized by search engines as authoritative. This approach optimizes content for primary keywords while also reinforcing relevance for long-tail keywords and peripheral topics, which often guide users throughout their search journey.
By expanding the knowledge graph in this way, ThatWare significantly enhances topical authority, positioning their clients as experts within specific niches. This process allows the organic integration of interconnected subtopics, improving user experience by delivering deeper, more cohesive information.
This method empowers ThatWare to create content that anticipates user queries and organizes topics into well-defined, logically ordered clusters. The result benefits users, who receive balanced and comprehensive information, and search engines, which recognize the page’s rich value, potentially improving its rankings.
⭐️Dynamic Page Segmentation for Relevance
Dynamic page segmentation enables ThatWare to identify specific sections of a web page that can be optimized, particularly beneficial for long-form content. Most web pages are composed of various content blocks, yet not all these blocks carry the same SEO value. With dynamic page segmentation, ThatWare can focus on optimizing the most relevant sections with targeted keywords or queries, rather than treating the entire page as a single entity. This enhances the relevance of a page, allowing us to boost specific areas while preserving overall content quality and user experience.
Additionally, advanced segmentation allows ThatWare to improve user engagement and content flow by guiding users to the sections that best match their search intent. This approach helps reduce bounce rates and increases the time users spend on the site. For content-rich pages, dynamic segmentation is especially effective, as different sections may address unique queries.
By integrating dynamic page segmentation into our SEO strategy, ThatWare maximizes relevance at both the macro and micro levels on each page, ensuring optimal performance and user satisfaction.
⭐️Inter-Document Relevance Modeling
IDRM, or Inter-Document Relevance Modeling, is a crucial technique that enables ThatWare to implement a cohesive SEO strategy by viewing individual documents on a site as interconnected elements rather than as standalone pages. This approach allows ThatWare to establish relevance between different pages, thereby broadening and deepening the topical scope of the site.
With this enhanced relevance among documents, we can guide users through an intentional and logical flow of content, strategically structured to answer more abstract queries, maintain user engagement, and rank effectively for complex search intents.
By utilizing IDRM, we also optimize the internal linking structure, ensuring that link equity flows toward strategically important pages. This approach enhances the visibility of critical content for search engines and presents a clear content hierarchy.
Additionally, IDRM enables us to design content clusters centered around a primary “hub” page, which directs users to related subpages, creating a comprehensive knowledge architecture. In doing so, ThatWare unifies various topics under a structured framework, building continuity and reinforcing the perception of the site as a powerful, interactive authority on multiple related subjects.
⭐️Polysemous Word Disambiguation
Polysemous word disambiguation is essential for clarifying search intent, especially when handling keywords with multiple meanings. Polysemous words are those that share the same spelling and pronunciation but have different meanings based on context. In SEO, these words present a challenge, as a single keyword can carry multiple intents, making it difficult to target content effectively.
ThatWare addresses this challenge by using advanced machine learning techniques and natural language processing to distinguish between different meanings of polysemous words. This enables us to align content with the precise intent behind user queries, improving relevance and accuracy.
Proper disambiguation of polysemous words enhances keyword targeting and increases content relevance. It results in highly focused content that addresses user needs while ensuring engagement metrics remain strong by avoiding irrelevant traffic.
By preventing common pitfalls in keyword targeting, ThatWare improves the clarity of client content, making it easier for search engines to interpret and rank pages appropriately. This approach not only drives more relevant traffic but also strengthens the SEO impact of our clients’ content.
⭐️Pattern Recognition for Query Matching
Pattern recognition in query matching is a powerful tool used by SEO agencies to interpret and respond to search engine queries effectively. At ThatWare, pattern recognition enables us to identify and understand the nuances in search queries, even when phrased differently. This process involves analyzing query data to identify recurring patterns or structures that can provide deeper insights into user intent. With advanced algorithms, our team can match these patterns to specific content, ensuring that the results are relevant and contextually appropriate.
In query matching, the focus is on detecting semantic patterns beyond simple keyword matches. For example, when a user searches for “best SEO agency for small businesses,” pattern recognition helps identify intent related to agency type, target audience (small businesses), and service quality (“best”). By identifying these patterns, ThatWare ensures that its clients’ content aligns precisely with the search intent, improving click-through rates and engagement.
Furthermore, our approach to pattern recognition is bolstered by machine learning models that continuously refine and adapt based on user behavior data. This dynamic learning process means that the more queries the system processes, the better it becomes at identifying subtle variations in language and intent. By prioritizing pattern recognition in query matching, ThatWare helps clients achieve higher rankings, providing users with content that closely matches their search needs.
⭐️Probabilistic Topic Modeling (e.g., PLSA)
Probabilistic topic modeling, particularly Probabilistic Latent Semantic Analysis (PLSA), is a technique ThatWare uses to uncover latent topics within a corpus of text. By analyzing co-occurrence patterns in words and phrases across various documents, PLSA identifies hidden semantic structures that define different “topics.” This technique is instrumental in understanding the thematic essence of content, ensuring that it is highly relevant to targeted keywords and search intents.
At its core, PLSA operates by modeling the probability distribution of topics within documents. Each document in a dataset is viewed as a mixture of topics, with each topic represented as a probability distribution over words. By decomposing content into these topic distributions, ThatWare can understand and optimize content for the full scope of user search queries. For instance, for an SEO-focused piece, PLSA can help identify subtopics like “on-page SEO,” “backlink strategies,” or “technical optimization,” making the content more comprehensive and appealing to a broader audience.
Additionally, PLSA assists in keyword expansion by identifying contextually relevant terms that align with target topics. Rather than solely focusing on primary keywords, the content developed through this process resonates with various related search intents, enhancing relevance and visibility. The result is more organic traffic and higher content engagement as users find more complete answers to their queries.
⭐️Disentangled Representations in LLMs
Large Language Models (LLMs) are at the forefront of natural language processing, and ThatWare utilizes disentangled representations within these models to enhance SEO strategy. Disentangled representations involve breaking down complex data into distinct, interpretable components that capture specific aspects of information. By separating these factors—such as sentiment, topic, or intent—ThatWare can develop content that precisely matches user search behavior, leading to more targeted and effective SEO.
In an LLM, representations typically consist of numerous factors or “dimensions” that encode information about words and their relationships. Disentangling these dimensions allows ThatWare to pinpoint relevant features, such as distinguishing between informational and transactional intent. This distinction enables more accurate query responses, improving search engine rankings by delivering content that aligns with specific user needs.
For example, disentangled representations allow us to optimize for nuanced search phrases, like distinguishing between “learn SEO basics” and “buy SEO tools.” Each query carries unique intent, and by using disentangled representations, ThatWare ensures that content is tailored to match this intent. This technique reduces ambiguity and improves the accuracy of query matching, fostering higher engagement and conversion rates. In addition, disentangled representations improve the interpretability of LLMs, making it easier to analyze and refine SEO strategies based on model insights.
⭐️Relevance-Based Fusion Techniques
Relevance-based fusion techniques are an advanced approach ThatWare employs to combine multiple signals or sources of information, ensuring search results are as pertinent as possible. Unlike traditional methods that may prioritize certain ranking factors over others, relevance-based fusion dynamically integrates various signals—such as click-through rates, user engagement, and content quality—to determine the most relevant content for each query.
This method involves aggregating diverse data sources, analyzing their relevance, and weighting them accordingly to produce highly accurate rankings. For example, if two pages rank well due to high-quality backlinks but differ in user engagement metrics, relevance-based fusion assesses which metric better reflects the user’s search intent. This way, ThatWare ensures that search results are fine-tuned to match both algorithmic and user-defined relevance, delivering optimized, valuable content to the audience.
In addition to enhancing search rankings, relevance-based fusion provides flexibility in SEO strategy. Since user behavior and search algorithms constantly evolve, a fusion-based approach allows ThatWare to adapt without overhauling the entire strategy. By weighting each factor dynamically, relevance-based fusion remains effective regardless of changes in search engine algorithms or user trends. This adaptability is key in the competitive SEO landscape, enabling ThatWare clients to consistently rank well.
⭐️Synthetic Data Generation for Training
In the world of machine learning and SEO, access to high-quality, diverse datasets is essential for effective model training. ThatWare leverages synthetic data generation to augment training data, ensuring that models are robust, well-rounded, and capable of handling a broad spectrum of queries. Synthetic data involves creating artificial yet realistic data points that mirror real-world search queries, user behaviors, and content structures.
Synthetic data generation is especially beneficial for rare or unique search scenarios where limited data is available. For instance, if a client operates within a highly niche industry, ThatWare can generate synthetic data to simulate user search patterns and behaviors in this niche. This approach ensures the SEO strategy is well-informed, even if organic search data is sparse. The generated data allows ThatWare’s models to improve query understanding and content relevancy, directly contributing to better search rankings.
Additionally, synthetic data is pivotal for training models to handle long-tail keywords and unconventional search phrases, expanding the scope of keyword targeting and improving visibility. By creating diverse data samples, ThatWare ensures that its clients’ content remains relevant across various search contexts, capturing organic traffic from both common and rare search terms. The result is a more resilient and versatile SEO approach, driving consistent engagement and traffic growth.
⭐️Aspect-Based Sentiment Analysis
Aspect-based sentiment analysis is what lets ThatWare break down the reviews into individual aspects within those same comments. It goes deeper and wider than general sentiment into positives, negatives, and more: it pinpoints exact sentiments that customers hold upon the aspects of the particular product or service such as quality, price, or design functionality. Therefore, focusing more on these unique features will help us to establish the content in line with user opinions and exactly what these users are concerned about increasing relevancy to search results.
👉Implementation at ThatWare
- ThatWare takes advanced algorithms of NLP for this understanding of feedback in other platforms that determine which point the targeted audiences find most important. For example, if it just mentions satisfaction in features of a product but talks about concern for its price; our strategy would discuss items such as durability or long-term value for the product in question. We take it up to be more sensitive or user-oriented because we always hit the points the people targeted are concerned with.
- Where our content experts and our SEO experts approach, as one team, such insight born out of the analysis of the sentiment that helps users align with their intent by word placement of those positive-attribute keywords so our user’s expectations can be matched exactly.
- This also helps rank well but enhances user satisfaction, fostering trust in the brand as well as repeat engagement. Continuous engagement with user sentiments helps us build a reputation of understanding and catering to customer needs, thereby lending to sustainable SEO benefits.
⭐️Graph Attention Mechanisms
Graph Attention Mechanisms allow us to explore and understand intricate relationships between entities-a real heart of how we conceptualize context and retrieve related information to a user’s intent.
In other words, these entities are not independent, isolated pieces of information: they connect within the whole broad knowledge graph and give greater importance to relevance with some particular search query. All of this enables us to serve a much more contextualized answer that can be more precious for complex, multilevel queries.
👉How ThatWare Uses Graph Attention Mechanisms?
ThatWare uses Graph Attention Mechanisms to give our content a contextual understanding structure. We can find and weigh nodes, which might encapsulate people, places, events, and so on into the knowledge graph with which attention is applied over such nodes, more contextually relevant to any user’s query.
For instance, if one types in “luxury watch brands with strong water resistance,” our method highlights entities more connected to luxury and strong water resistance, such as Rolex and Omega, yielding a more accurate and complete search experience for the end user.
Our team collaborates with GAM tools that are driven by AI, implementing successful content and interlinks related to entities. This enables search engines to perceive our content as topically relevant for niche queries.
In application, Graph Attention Mechanisms do not only improve the capability of ThatWare in mapping user intent but also help in strengthening the position of our content by filling in gaps in complex searches. In the end, it improves both visibility and engagement, which strengthens ThatWare’s competitive advantage in the digital world.
⭐️Bilinear Transformation Models
Bilinear transformation models can be highly effective for representing user intent and content attributes and assisting ThatWare in developing a ranking model that is perfectly in sync with what the users want.
Therefore, by interlinking these two facets of the business in an organized manner, we ensure that our content meets the very expectations and needs of the intended audience, making it relevant and competitive in this ruthless world.
👉How We Use Bilinear Transformation Models?
At ThatWare, we improve the relevance of our content by using bilinear transformation models. From these models, we infer how user intent, such as wanting environmentally-friendly products, interconnects with content attributes-for example, sustainable materials or eco-friendly packaging.
So, if a user searches for “recycled clothing brands,” our system would highlight content featuring recycled materials and environmentally friendly practices.
Hence, such a customized approach makes our content highly relevant and valuable for specific searches, thus having a higher chance of click-throughs and user satisfaction.
It enables ThatWare to create material that is currently ranking well for targeted queries based on relevant attributes and keywords. It also ensures that our approach is dynamic and adaptable to changes in user needs and market trends.
This will enable us to hone in on the exact intersection of user intent and content attributes so that ThatWare stands as a responsive and user-oriented brand, which further strengthens both short-term ranking and long-term engagement.
⭐️Contextual Inference on Queries
Contextual inference will help ThatWare to clear out unclear queries as, with the help of contextual clues, we get hold of the intent behind any query. This would really be important for answering correct queries that have many different meanings, such as one term having multiple meanings, or is just a bit ambiguous. Knowing more about the context, we’ll know what the users need, and our content will give maximum relevance.
At ThatWare, we tap the potential of the machine learning algorithms that infer the intent behind the ambiguous search. Our systems would know, for example, that searching for “java programming” versus “java” requires the correct context: that one is a reference to coding and technology, while the latter is possibly the coffee or island in Indonesia. Such refinement allows content to change dynamically, based on intent, in order to make results more relevant.
Our team utilizes information on the behavior of users and the history of trends to refine those inferences so that every piece of content answers the actual needs of a searcher. It delivers very personalized and highly relevant content. ThatWare improves user satisfaction, which consequently increases rankings in search engines. The approach of ThatWare, therefore, strengthens its brand reputation. Users are more likely to return to the same source that consistently meets their expectations.
⭐️Spatio-Temporal Information Ranking
This combines location-based data and time-based data to ensure the delivery of relevant results to the current situation of a user. This is very essential to searches whose key criteria involve location or timing. Local events, restaurant recommendations, or seasonal offers require the inclusion of these two dimensions to make ThatWare produce updated, geographically relevant content in line with users’ needs at the same moment.
ThatWare utilizes spatio-temporal ranking where our content is relevant on a time-sensitive as well as geographically specific basis.
For example, whenever a user searches for something like “outdoor concerts in New York this weekend,” our system will rank our content based on what’s happening in New York and at that said period. Through such means, when the ability to consume information applies the knowledge right away, chances for conversion increase with the time-sensitive offers.
Our team strategically creates and organizes content around high-demand seasonal and local searches. This way, we can enjoy the benefit of traffic spikes as long as the content is well-aligned with current events and local relevance.
Moreover, ThatWare captures more traffic and offers an experience that users will find timely and valuable in the process. In the long run, this helps increase ThatWare’s authority in localized and event-driven searches, hence leading to sustainable ranking improvements.
⭐️Masked Language Modeling for Inference
Masked Language Modeling (MLM) improves ThatWare’s ability to answer user queries partially or vaguely. By predicting the missing words or phrases, MLM fills in content gaps that help us better understand the intent behind vague searches. For example, if a user makes partial queries or omits certain information, this predictive power helps generate contextual completeness in our responses.
👉How ThatWare Utilizes Masked Language Modeling?
This will integrate MLM into the process of SEO, whereby user queries are analyzed for the possible missing elements and predicts what those missing elements could be.
For example, when a user is searching for “best laptops for,” MLM algorithms expect keywords like “programming,” “gaming,” or “students” in the query, thus making the query more relevant. Therefore, this process helps ThatWare to offer content that accurately fits into the expectations of the user, even when the original query is incomplete.
Our SEO specialists and data scientists are fine-tuning MLM algorithms in tandem with improving the accuracy of predicting the missing words. It’s beneficial for search result quality but gives ThatWare a competitive edge in being able to better understand its users’ needs, more likely to click on the content that directly answers the users’ needs.
Ultimately, it ensures the content is doing just that to drive traffic and engagement over time.
⭐️Personalized Knowledge Graphs
Personalized knowledge graphs allow ThatWare to create unique content experiences for users based on previous engagements, preferences, and browser history. In short, we outline personal content structures that provide very relevant recommendations that connect to the particular user, resulting in greater engagement and loyalty towards the brand.
👉Implementation at ThatWare
We apply personalized knowledge graphs for content recommendation at ThatWare. If a user often looks up information on electric cars, the system will emphasize such related topics as charging stations, tax incentives, and how to drive ecologically friendly in future sessions. Thus, the user’s interest is matched, which in turn will increase the chance of revisiting and having positive interactions.
Our team takes such information and creates dynamic knowledge graphs while users are interacting with our content. This way, each experience will be unique to them. Such fine-tuned content is understood by search engines as extremely relevant.
Thus, ThatWare increases its ranking potential. In the end, such increased user satisfaction will boost the reputation of ThatWare as a user-centric and reliable source of information.
⭐️Contrastive Loss Fine-Tuning
- Fine-tuning with contrastive loss enables ThatWare to bring to view subtle, yet crucial, distinctions of content and therefore enhance competitive positioning. The process involves a special type of loss function that decreases similarities between pieces of content and therefore increases the generality of our material to be clear and relevant. This then, with a difference from other sources, obtains better ranking visibility and search relevance.
- It will be fine-tuned content on ThatWare using contrastive loss for it to stand out. Its unique insights and perspectives, for instance, will keep it standing out from crowded fields like fitness advice through distinct aspects such as exercises that are suited to age or equipment that is eco-friendly. That which the competition does not seem to focus so much on is keeping the content on ThatWare well-ranked for niche or super-specific searches.
- Our SEO and content teams fine-tune our content together, based on user engagement data, honing into attributes that mean the most to our audience. This will help both improve ranking and increase user trust as readers can rely on ThatWare for insightful, relevant information.
As time progresses, fine-tuning with contrastive loss will prime more engaging content from ThatWare as a source of unique, high-value content.
⭐️Fact-Checking Models for Ranking Accuracy
Fact-checking models have to be present in any content to keep the credibility on track because they verify all the facts before publishing. Since search engines always put forward reliable information, fact-checking increases ThatWare’s rank because it increases our reputation for providing trustworthy information. Accuracy plays a more significant role in content simply because critical information, especially medical or legal topics, is important for ranking as well as user satisfaction.
👉How ThatWare Uses Fact-Checking Models?
- At ThatWare, we used real-time fact-checking models cross-referencing data on reputable databases and sources. For instance, when it comes to health and wellness material, we actually cross-check every content against the authoritative medical databases for credibility. Hence, because of this, credibility will be advanced and most importantly user trust will be maximized where visitors will engage better with well-researched and factually correct material.
- Our commitment to accuracy allows ThatWare to build a robust online reputation, which is very important for SEO success. People stay longer and come back more often, and search engines reward us with better rankings. Fact-checking is an essential component of ThatWare’s sustainable, long-term approach to SEO growth.
⭐️Context Aware Attention Routing
Context-aware attention routing optimizes the ability of ThatWare to serve highly relevant content by focusing selectively across various documents based on the context of each query. Such an approach relies on state-of-the-art attention mechanisms, ensuring that only the most pertinent pieces of information get prioritized focus, and thus allows for a much more efficient and relevant process of information retrieval.
That is precisely how we align the content recommendations at ThatWare by using context-aware attention routing: close to what is on the user’s intent. Whenever there is more than one aspect or maybe answers within a query, then it helps zero in on the most contextually relevant parts of a whole lot of documents.
For example, when a user enters the query “best investment options during inflation,” the system selectively prioritizes all of the most important financial assets and economic contexts in contrast to lesser ones.
Our SEO team incorporates these routing mechanisms to ensure users receive information that best matches the intent of the query. This selective attention leads to faster, more accurate content delivery, which enhances ThatWare’s user engagement rates and reduces bounce rates.
Therefore, by ensuring high-quality relevance, we enhance search engine rankings and increase user trust. It helps in positioning ThatWare as a provider of content that truly understands and meets user needs.
⭐️Meta-Learning for Intent Recognition
Meta-learning would allow ThatWare to generalize intent recognition over a variety of queries though they could be in extremely different wording or even utilize new phrases. This harnesses the power of machine learning to train models comprehensively over a wide array of intent types, creating a foundation that is adaptable enough for the recognition of intent between different contexts with minimal training.
👉Application of Meta-Learning at ThatWare
ThatWare makes use of meta-learning for the recognition of intent so that the content we are providing does not go stale and also responds to changing search trends. For instance, while users are beginning to find emerging technologies or newly popularized trends, our models can infer probable intent on these queries even without too much data. Users begin with searches around “virtual reality wellness,” and our models can now interpret this as intently related to fitness and well-being and respond accordingly with relevant content.
Our SEO specialists and data scientists work together to update content and keyword targeting according to the insights derived from the meta-learning model. This responsiveness to prevailing user needs and evolving patterns of search positions ThatWare is in a commanding position relative to ranking adaptability. The facility to address the intent behind a search in an unending flow of changing contexts enhances That Ware’s reputation as a creative, visionary content publisher.
⭐️Cross-encoder ranking models
ThatWare’s content is optimized through cross-encoder ranking models by encoding both query and document at the same time. In doing so, depth and quality in relevance comparison are improved. Through encoding of both elements, a cross-encoder model supplies an explanation that can be much deeper for relationships between user queries and content, which generally leads to highly accurate outcomes for ranking.
At ThatWare, we use cross-encoder ranking models to create content that dynamically responds to complex user queries. So if a user searches for “sustainable fashion brands that use recycled materials,” the cross-encoder model identifies an exact match and ranks the content on recycling practices in the sustainable fashion industry. Such fine-grained comparison helps us deliver results with a high degree of precision in alignment with the nuanced intent behind each search.
The ThatWare team applies these models across our content ecosystem, ensuring that we consistently provide accurate and engaging content for targeted queries. This approach boosts user satisfaction, as the results they receive are tailored to their specific needs. Cross-encoder ranking models enhance ThatWare’s content visibility by reinforcing relevance, a critical factor in driving higher rankings and stronger SEO performance.
⭐️Dual-BERT Fine-Tuning for Query Relevance
Dual-BERT fine-tunes two encoders-one encodes the query, while the other encodes the document-and gives a deeper insight into both content context and user intent. In return, this allows ThatWare to give proper answers to its users according to their queries because it helps BERT learn the interaction between a query and a document through dual encodings.
👉Application of Dual-BERT Fine-Tuning at ThatWare
- It means ThatWare applies Dual-BERT fine-tuning to update the contents using two sources at the same time: query contexts and document contexts. When it reaches the point that a specific user submits a query “benefits of cloud computing for small businesses,” we simultaneously use our dual encoder model to interpret the actual intent behind the query with the help of subject matters related to the content provided. This fine-tuning means our content is accurate, targeted information for users, and boosts their engagement and satisfaction.
- Our content experts and technical SEO team refine content together using Dual-BERT, making ThatWare material full of context and targeted toward nuanced search intent.
- Improving query-document understanding increases ThatWare’s potential for ranking and improves the retention of users through interesting and relevant content.
The dual-encoder approach will help ThatWare establish itself as a correct and trusted source of information over time.
⭐️Meta-Path Based Recommendation
The meta-path-based recommendation is the utilization of path-based relationships between entities and relationships in knowledge graphs to identify related content and recommend it. Such a technique helps ThatWare build an information network to enable users to discover relevant content more effectively and therefore be engaged deeper in our material.
We, in ThatWare, utilize meta-path-based recommendations in an effort to make the journey of a user more attractive by recommending relevant content to him. For example, after a user has read an article regarding “renewable energy sources,” our meta-path recommendation system can send further recommendations to him for reading other pieces of content like “applications of solar energy” or “efficiency in wind power.”It offers the user a better experience and encourages him to read more from ThatWare.
We implement these recommendations by making strategic content linking and mapping entity relationships. After that, the search engines can identify our content as informative with a high degree of connectivity. The more the various content that the users use at ThatWare, the greater the improvement in our search engine optimization. It also increases dwell time, providing a positive signal to the search engine that the contents are highly relevant.
Hence, after creating a chain of highly relevant topics, ThatWare also helps improve user experience through improved authority and ranks for the content.
✨Our Hyper-Intelligence SEO Video
⭐️Query-Driven Fine-Tuning of LLMs
Query-driven fine-tuning is a procedure for adapting these large language models for any domain or query. Query-driven fine-tuning means tailoring huge language models in a very specific domain or particular query where ThatWare needs to offer answers that could meet the specific needs of an end-user better. The result could be a great fine-tuning of the content toward the real questions that users might most likely type, giving extremely pertinent and understandable answers.
👉Query-Driven Fine-Tuning Implementation at ThatWare
ThatWare uses query-driven fine-tuning of our language models to what is frequently and of high value in our target domains. For example, within the digital marketing domain, the fine-tuning of the models may engage with the use of “SEO for eCommerce,” “local search optimization,” or “voice search trends.” Fine-tuning ensures our content is very accurate and valuable and meets the needs exactly of our target audience. We ensure that the ThatWare content is relevant by focusing on popular search trends.
Our technical team is attentive to keyword performance and trends and uses this information to fine-tune our models about user interests. With this, ThatWare will be able to produce the most targeted information that search engines consider valuable, hence helping us improve our ranking potential and supporting our strategy for long-term SEO success.
So, such a method not only makes ThatWare better at offering relevant content but also establishes our authority as an expert provider of up-to-date information.
👉End Note
Using such complex techniques, ThatWare thus produces a user-centric, technologically advanced SEO strategy that not only drives rankings but lays a solid foundation for long-term success and audience loyalty. ThatWare leads the ever-changing SEO landscape with meaningful, personalized, and credible content on every search by blending technical precision and user-focused strategies.
ThatWare combines state-of-the-art technology with user-oriented tactics in combining the perfect, solid groundwork for durable and long-lasting SEO triumphs. From context-aware attention routing to query-driven fine-tuning, it provides an environment that means we stay right at the helm of how SEO might shape itself tomorrow.
Thus, by implementing these sophisticated techniques, ThatWare shares relevant content keeping the search intent in mind. Our Hyper-Intelligence SEO strategies in 2025 would meet the expectation both from the users and from the search engines to rank and develop a brand reputation.
Hiring ThatWare provides businesses with strategic expertise, affordable pricing, extensive experience in digital marketing, and access to a full team capable of implementing successful search marketing campaigns. The company’s adaptability and commitment to experimentation are key advantages for businesses seeking effective SEO solutions.
Thatware | Founder & CEO
Tuhin is recognized across the globe for his vision to revolutionize digital transformation industry with the help of cutting-edge technology. He won bronze for India at the Stevie Awards USA as well as winning the India Business Awards, India Technology Award, Top 100 influential tech leaders from Analytics Insights, Clutch Global Front runner in digital marketing, founder of the fastest growing company in Asia by The CEO Magazine and is a TEDx speaker.