SUPERCHARGE YOUR Online VISIBILITY! CONTACT US AND LET’S ACHIEVE EXCELLENCE TOGETHER!
⭐️Improving Factual Accuracy in Search and its SEO Applications
The Concept of Topical Authority and the strategy around it that involves microsemantics, and macrosemantics come from the Topical Authority Course of Koray Tugberk GUBUR, owner and founder of Holistic SEO, and we are proud of giving credit to the original source and thought leader.
Improving the Factual accuracy of answers to different search queries is one of the top priorities of any search engines. The internet is full of information. Search engines like Google train large language models like BERT, RoBERTa, GPT-3, T5 and REALM to create large natural language corpuses (datasets) that are derived from the web. Finetuning these natural language model, search engines are able perform a number of natural language tasks.
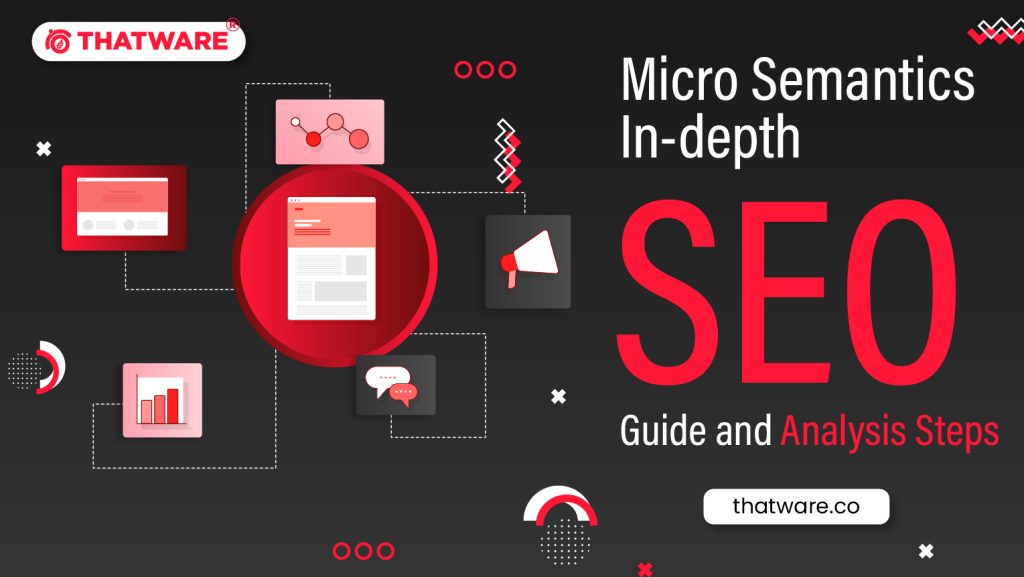
⭐️The Problem of Bias in Search
In earlier days the challenge of Google was to accurately understand the user intent behind queries. There was the days when Google couldnot really help you in planning a trip to mount fiji or give you detailed suggestions for an itinerary.
However nowadays when you type to create a itinerary for travel to mount fiji, Google can accurately understand your search intent and suggest you webpages and answers related questions that can help you plan your trip.
It can also help you book your hotels or flights directly:
With the advent of Hummingbird, Rankbrain and large language models like BERT and LAMBDA, Google over the years have evolved enough to accurately understand and deliver results as per the user intent.
However as the Internet gets crowded with content , one of the most recent challenges is to not only to deliver the most relevant information but also factually correct information.
Here’s an example of Factual Inaccuracy:
In the Azerbaijan Grand Prix, you’ll notice a problem with the rich results. The McLaren Renault cars, in positions seven and nine, aren’t called McLaren Honda as Google shows.
As per the official website of McLaren and Wikipedia, Mclaren uses Renault Engines and not Honda engines!
Also a search for “prime minister of Armenia leaves out the fact that Serzh Sargsyan was prime minister from 2007 to 2008 in the carousel:
Factual Innaccuracies are unacceptable as they cause Bias and for a search engine it is of primary importance to serve factually correct information from the Internet without user created biases.
⭐️Source of Factual Information | Knowledge Graphs
In order to give more leverage to factually accurate content , Google introduced the concept of KGs or Knowledge Graph.
The Knowledge Graph is an intelligent model that taps into Google’s vast repository of entity and fact-based information and seeks to understand the real-world connections between them.
Instead of interpreting every keyword and query literally, Google infers what people are looking for.
The goal of the Knowledge Graph – as Google explains nicely in their (still relevant) introductory video – is to transition “from being an information engine [to] a knowledge engine.”
Google displays what it deems to be the most relevant information in a panel (called a Knowledge panel) to the right of the search results, based on the Knowledge Graph’s understanding of semantic search and the relationship between items.
In its early days, these results were static, but today you can book movie tickets, watch YouTube videos, and listen to songs on Spotify through these panels.
⭐️Are Knowledge Graphs and Rich Results the Same?
With knowledge graphs one may ask a question as to are knowledge graph the same as to other search features like featured snippet.
Although knowledge graph and featured snippets seems use the same styling and images patterns, the main difference are as follows:
Featured Snippet The Featured snippet is just one aspect of a search feature which has a singular purpose of delivering the most relevant answer to a query. | Knowledge Graphs The knowledge graph is an underlying algorithm that helps Google keep the most relevant and factual information in the form of entities. These information is used to deliver various kinds of search engines results including featured snippets. |
Featured Snippet cannot be requested to change or update. | 2. You can suggest changes to the Knowledge Graph, especially where it concerns your brand identity and information. |
⭐️How to Reduce Bias in Search Results | Introduction of KELM Algorithm
KELM is an acronym for Knowledge-Enhanced Language Model Pre-training. KELM is an acronym for Knowledge-Enhanced Language Model Pre-training. Natural language processing models like BERT are typically trained on web and other documents. KELM proposes adding trustworthy factual content (knowledge-enhanced) to the language model pre-training in order to improve the factual accuracy and reduce bias.
⭐️Background to KELM
Natural Language Text, are often include biases and factually inaccurate information. However alternative data sources like Knowledge Graphs contain structured data. KGs are factual in nature because the information is usually extracted from more trusted sources, and post-processing filters and human editors ensure inappropriate and incorrect content are removed.
Therefore any natural language model that can incorporate these have the advantage of factual accuracy and reduced biases. However the structured nature of these data make them difficult to be incorporated in natural language models.
In KELM Pre Training of a Language Model, Google tried a conversion method of KG data to natural language in order to create a synthetic corpus.
Then they utilize REALM which is a retrieval based language model on the synthetic corpus as method of integration both natural language corpus and KGs in pre-training.
⭐️Converting KGs to Natural Language Text
Let us understand this with a simple example.
KGs consist of factual information represented explicitly in a structured format, generally in the form of [subject entity, relation, object entity] triples, e.g., [10×10 photobooks, inception, 2012]. A group of related triples is called an entity subgraph. An example of an entity subgraph that builds on the previous example of a triple is { [10×10 photobooks, instance of, Nonprofit Organization], [10×10 photobooks, inception, 2012] }, which is illustrated in the figure below. A KG can be viewed as interconnected entity subgraphs.
Source: Google AI Blog
Converting an entity subgraph into natural language is a standard data to text processing task. However converting an entire KG into a meaningful text has additional challenges.
Also real world KGs are more granular and vast than benchmark KGs. Also with benchmark datasets, they have subgraphs predefined that can form meaningful sentences. With an entire KG, such a segmentation into entity subgraphs needs to be created as well.
In order to convert the Wikidata KG into synthetic natural sentences, we developed a verbalization pipeline named “Text from KG Generator” (TEKGEN), which is made up of the following components: a large training corpus of heuristically aligned Wikipedia text and Wikidata KG triples, a text-to-text generator (T5) to convert the KG triples to text, an entity subgraph creator for generating groups of triples to be verbalized together, and finally, a post-processing filter to remove low quality outputs.
The result is a corpus containing the entire Wikidata KG as natural text, which Google call the Knowledge-Enhanced Language Model (KELM) corpus. It consists of ~18M sentences spanning ~45M triples and ~1500 relations.
⭐️How KELM Works to Reduce BIAS and Improve Factual Accuracy
KG Verbalization is an efficient method of integrating KG with natural language models.
In order to assess the impact of search result accuracy, Google researchers tried to augment the REALM corpus which contains Wikipedia text with the KELM corpus (verbalized triplets).
They measure the accuracy with each data augmentation technique on two popular open-domain question answering datasets: Natural Questions and Web Questions.
Augmenting REALM with concatenated triples alone accounts for improved accuracy. However the use of verbalized triplets accounts for a smooth integration of KG data as well which is confirmed by the improvement in accuracy.
⭐️Impact of KELM in Reducing Bias and Improve Search Accuracy
Google conducts extensive research, some of which appears to be exploratory but otherwise appears to be fruitless. The conclusion of research that is most likely not going to be incorporated into Google’s algorithm typically states that additional research is necessary because the technology in question doesn’t meet expectations in any particular way.
With the KELM and TEKGEN studies, however, such is not the case. In actuality, the essay is upbeat about the discovery’ potential for practical implementation. This seems to increase the likelihood that KELM will eventually appear in search in some capacity.
Extract from Google AI Blog on KELM
⭐️What does it Mean for SEOs?
Wether Google Introduces into Search or develops on a more advanced corpus , one thing is quite clear, that Knowledge Graphs are the most important and vital source of factual information, and hence all brands and SEO must target to achieve it.
⭐️How to Achieve a Knowlegde Panel?
There are no direct ways of obtaining a knowledge panel. However, several resources on Google’s docs and our understanding of the Knowledge Graph generation process helps us to identify certain steps vital for achieving a Knowledge Panel.
- Leverage Schema on Home Page
Visitors cannot see schema markup, but it is essential for the Knowledge Graph to understand your company’s information.
Include any and all pertinent information, including company, individual, and nearby business. Utilise markup as much as you can because the Knowledge Graph may gather up any data using Schema.org elements.
- Define Entities in Schema Markup
Your website brand is itself an Entity. Similarly different service pages and products on your website may describe different entities some of which may be unique to your brand. Indexing these entities into Google is crucial for strengthening your knowledge Graph.
It is possible to define the Entity of a Page using Schema. Read more about Main Entity of Page Schema.
- Get Listed at WikiData.org and Wikipedia
For official website addresses, Google frequently uses Wikipedia (unless you provide them yourself).
Therefore, it should go without saying that if your company doesn’t already have a Wikipedia page, you should either make one yourself or pay a reputable Wikipedia editor to do it for you.
Make sure to add an entry about your company to Wikidata and link to it from your Wikipedia article because Google also uses Wikidata for some of its information.
Other Suggestions
- Local Business Listings like Google My Business and Bing Places.
- Get Listed in Popular Business Directories
- Verify Social Media Accounts.
⭐️Unlocking Topical Authority: Building A Topical Map for Semantic SEO for Unbeatable Organic Growth
Topical Authority and Semantic SEO are no doubt some of the most groundbreaking advancements in search that have revolutionized how SEO works. Now outranking your competitor is not that tough when you actually know how search engines’s work and can master the art of building topical authority.
We have been applying the methodologies of Topical Authority and Semantic SEO for our client’s and our own website and we have seen significant improvements in the traffic and project stats.
In this article we will covering the basics and provide actionable steps on how an average SEO can understand the concepts of topical authority and take advantage of it by building a topical Map.
But before that let’s show some results.
5 months GSC Data of GetWordly.com
Last 6 Months GSC Data for a9-play.com
Last 12 Months Growth Data for upperkey.com
⭐️Brief Introduction to Semantic Web, Semantic Search And Topical Authority
The way information is currently organised on the web is known as the semantic web. Taxonomy and ontology are two fundamental components of the semantic web that derive from the universe and the nature of the human brain, respectively.
The words “taxonomy” and “nomia,” which together mean “arrangement of things,” are derived from the Greek words taxis and nomo, respectively. Ontology, which means “essence of things,” is derived from the words “ont” and “logy.” Both are methods for defining entities by grouping and categorising them. The semantic web is comprised of taxonomy and ontology.
Google has developed several projects that are geared towards a semantic web over the last ten years.
Google introduced the “Structured Search Engine” in 2011 to organise the information on the internet.
Additionally, they introduced Knowledge Graph in May 2012 to aid in the understanding of data pertaining to actual entities.
In order to understand the relationships between words, concepts, and entities in human language and perception better, they introduced BERT in 2019.
The semantic web, semantic search, Google as a semantic search engine, and consequently semantic SEO were all produced by these processes.
⭐️What is Topical Coverage? How It is Correlated to Topical Authority?
Every source of information has a different level of coverage for various topics in a semantic and organised web. Through their shared characteristics, things or entities are related to one another. The “Ontology” that these attributes represent. Within a classification hierarchy, things are also connected to one another. The “Taxonomy” is represented by this hierarchy. A source needs to cover a topic’s various attributes in a variety of contexts in order to be considered an authority for that topic by a semantic search engine. Additionally, it must make use of analogous items as well as parent and child category references.
The key to these SEO case studies is building a content network for every “sub-topic,” or hypothetical question, within contextual relevance and hierarchy with logical internal links and anchor texts.
The most comprehensive content network that is entity-oriented, semantically organised, and can acquire Topical Authority and Topical Coverage. Every piece of content that is successful increases the likelihood that other content will also be successful for the connected entities and related queries.
⭐️Steps To Build Topical Authority and Leverage Semantic SEO
Understanding why a search engine needs the web to be semantic is necessary to fully grasp the semantic SEO concept. This need has grown even more, particularly with the prevalence of machine learning-based search engine ranking systems rather than rule-based search engine ranking systems and the use of natural language processing & understanding technologies. To comprehend the suggestions below, approach these ideas from the perspective of a search engine.
- Create a Topical Map before Starting to Write an Article
You should check Google’s Knowledge Graph because there may be different connections between things for Google than there are according to dictionaries or encyclopaedias. Google’s entity recognition and contextual vector calculations use the web and data supplied by engineers.
In order to determine which entity has been related to which and how for which queries, you should also check SERP.
You can check a niche and query group quickly in preparation for creating a topical map.
- Examine the sitemaps of your competitors to learn about their topical maps.
- Obtain relevant topics and queries from Google Trends.
- Gather information from search suggestions and autocomplete.
- Take note of how the content hubs of your rivals are connected.
- Google Knowledge Graph can be used to retrieve permanent entities.
- To view entity properties, hierarchies, and connections, use non-web resources.
The final point is crucial if you want to develop into a source that contributes reliable, original information to a search engine’s knowledge base.
Example of a Topical Map from Inlinks.net
- Determining Link Count Per Page
All of these SEO case studies and accomplishments had a maximum of 15 links on each webpage.
The majority of these links had natural anchor texts that were pertinent to the main content. I skipped the header and footer menus. This runs counter to conventional technical SEO advice. I had to come to terms with that, and I’m not advocating using no more than 15 links per web page. I’m advising you to keep the pertinent and contextual links within the text’s main body and work to draw search engines’ attention to them.
Use the following checklist to estimate the number of links to be used on the Website:
- To understand the minimum and maximum values, consider the industry standards for internal link count.
- The quantity of named entities in the text
- The number of named entity contexts
- The content’s degree of “granularity”
- There can only be one link per heading section.
- if the entities are in “list format,” linking them to the relevant pages for entities of the same type.
- Implement Anchor Texts in a Natural And Relevant Way. Determine Count, Position and Words
It is already well known that anchor text are very useful in determining link relevancy and also determine the Page Rank Passage. However one should not use the same anchor text more than three times in a document. The fourth time, it should have a different wording. Some other rules are:
- Never use the first paragraph of a page’s text as the anchor text for links to that page.
- Never link to a page using the first word of any paragraph on the page.
- Always use one of the last heading’s paragraphs when linking one article to another from a different context or tangential subject (Google refers to this kind of connection as “Supplementary Content”).
- Always look at the internal and external anchor texts of competitors for a specific article.
- When writing anchor texts, make an effort to always use synonyms for the topic.
- Always verify whether the “anchor text” is present in the content of the targeted web page and any associated heading text from the link source.
- Determine Your Contextual Vectors
Again, the terminology might be a little “scratchy” for your ears. For me, this is a term from Google Patents. Contextual domains, contextual phrases, and contextual vectors… Google Patents offer a wealth of information to explore (thanks again to our educator, Bill Slawski).
Contextual vectors are the signals used to determine the angle of content, to put it simply. A context can be “comparing earthquakes,” “guessing earthquakes,” or “chronology of earthquake,” with “earthquake” as the topic.
For instance, Healthline has more than 265 articles devoted solely to the topic of “apple” (a type of fruit). The advantages of apples, their nutritional value, varieties, and apple trees (basically a different thing entirely, but it is close enough.)
All of these websites were, therefore, related to the field of teaching second languages. The primary subject is “English Learning”; examples of different contexts include learning English through games, videos, movies, songs, and friends.
Contextual Vectors Diagram. A schema from Google’s User-context-based Search Engine Patent. “A vocabulary list is created with a macro-context (context vector) for each, dependent upon the number of occurrences of unique terms from a domain”
We always try to use a variety of pillar cluster contents to bridge the gaps between various topics and the entities contained within them in order to establish more contextual connections. You should also read Google’s patents to learn more about their contextual vectors and knowledge domains.
- Does Content Length Matter For Ranking?
Content Length is not a ranking factor. Actually, for many factors like crawl budget, PageRank distribution, backlink dilution, or cannibalization issues, telling more things with less content in more thorough and authoritative articles is preferable.
But in order to plan the process, content count is crucial. You must determine how many writers you will need and how many articles you will publish each day or each week. In this executive summary, I left out a lot of SEO terminology like content publication and content update frequency. You still do not know how much content you will need, even after choosing the topics, contents, contexts, and entities. Google occasionally favours websites that display multiple contexts for a topic on the same page, but in other cases Google prefers to see different contexts on different pages.
Average Heading Count per heading level on the web pages
To know the exact count for the content/article, examining the Google SERP types, competitors’ content network’s shape is important. This is also important for the budget of the project. If you tell your customer that you just need 120 pieces of content but later, you realize that you actually need 180 pieces of content, it is a serious problem for trust.
- Determine Topical Hiearchy and URL Categories
In none of the SEO case studies presented here, URL categories were used. This does not imply, however, that URL categories and associated breadcrumbs are not advantageous for semantic SEO. It is simpler for a search engine to understand a website when similar content is kept in the same folder in the URL path. Additionally, it offers user advice and makes site navigation easier.
Oncrawl’s Inrank Flow Distribution for different URL Categories. One can easily see the most important part of OneCrawl Website is the Blog. This is true for most SAAS Websites.
- Creating a Topical Hiearchy and Adjusting with URL Categories
The use of subtopics by Google in January 2020 has been confirmed, but the term “Neural Nets” or “Neural Networks” has actually been used by Google before. There was also a nice summary of how topics are connected to one another within a hierarchy and logic on the Google Developers YouTube channel. Taxonomy and ontology are essential for semantic SEO because of this once more.
The phrase “creating a Topical Hierarchy with Contextual Vectors” however, what does that mean? It implies that each topic should have been processed in all relevant contexts and groups with logical URL structures.
A more granular and detailed information architecture will result in the search engine giving a source greater topical authority and expertise.
- Adjust your Heading Tags (Heading Vectors)
As a signal for identifying the primary angle and topic of the content, heading vectors are actually just the order of the headings. The “Main Content,” “Ads,” and “Supplementary Content” sections of content are seen as having different functions in accordance with the Google Quality Rater Guidelines.
We all know that Google gives more weight to the content in the “upper section” or area of the article that is visible above the fold. The queries in the upper section of the content always have a higher rank than the queries in the lower section for this reason. In reality, Google considers the bottom section to be “supplementary content.”
A representation of Google’s methodology for Contextual Answer Passage Score Calculating via Heading Vectors.
Use of contextual relevance and logic within the heading hierarchy is crucial for this reason. Simply put, from the standpoint of semantic SEO, the following are some fundamental guidelines for heading vectors:
- Use semantic HTML tags, including heading tags, regardless of what the search engine says.
- The title tag serves as the starting point for heading vectors, so they should be consistent.
- Any paragraph that follows those headings shouldn’t reiterate the information that was previously provided because each heading should concentrate on a different piece of information.
- Group headings that concentrate on related concepts together.
- Any heading that calls for the inclusion of another object should have a link to that object.
- The content of each heading should be properly formatted with lists, tables, and descriptive definitions.
As you can see, this section as a whole follows some simple logic. nothing brand-new. However, allow me to present one of Google’s patents below, titled “Context scoring adjustments for answer passages.”
Source: Context scoring adjustments for answer passages
Google tries to determine which passage has the best contextual vector for a given query by using the heading vector. Therefore, I advise you to establish a distinct logical structure between these headings.
- Connecting Related Entities for a Topic Within a Context
Entity associations and connecting entities are similar concepts. Search engines can associate entities based on the attributes of the entities and also based on how queries are written for a potential search intent.
An ontology’s practical application is the linking and grouping of entities within a context. For instance, in the context of these SEO projects’ industry, “English Learning,” you can also use “Irregular Verbs,” “Most-used Verbs,” “Useful Verbs for Lawyers,” “Etymology of Verbs of Latin Origin,” and “Less Known Verbs” that can be connected to one another for the topic of “Phrasal Verbs.”
All those contexts actually focus on “verbs in English”. They are all related to “Grammar Rules”, “Sentence Examples”, “Pronunciation” and “Different Tenses”. You can detail, structure, categorize and connect all these contexts and entities to each other.
After you basically cover every possible context for a topic and all related entities, a semantic search engine doesn’t have any other chance besides choosing you as a reliable source for possible search intents for these.
- Cover All Possible Search Intents using Question and Answers
In essence, a search engine creates questions from web content and uses query rewriting to match these questions with queries. And it employs these queries to fill in any potential content gaps for conceivable web search intentions.
That is why I advise you to consider each entity in each context while linking them together. You should be aware of information extraction, though. Information extraction involves sifting through a document for the key details and unmistakable connections between ideas. A search engine can determine which questions can be answered from a document or which facts can be understood thanks to information extraction. Information extraction can even be used to create a knowledge graph between entities and their attributes, and used for generating related questions.
Generating Related Questions for Search Queries
Don’t just concentrate on the SEARCH VOLUME! It’s possible that this question has never been posed before. Even the search engine may not have the solution to this problem. Create and respond to these inquiries, however, and become a distinctive source of information for the web and search engines in your niche if this particular information is useful for defining the characteristics of entities within the topic.
- Focusing on Finding Information Gaps Rather than Keyword Gaps
Source: Patent “Contextual Estimation Of Link Information Gain”
We are all aware that even as recently as 2020, “Google uses RankBrain to match these queries with possible search intents and new documents” since “15% of everyday queries are new.” Additionally, Google is constantly looking for original data and solutions to conceivable new questions from its users. Try to include less well-known “terms, related information, questions, studies, persons, places, events, and suggestions” as well as original information.
For these SEO case studies, “longer content” or “keywords” are therefore not the key. The keys are “more information,” “unique questions,” and “unique connections.” Each piece of content for these projects has a distinctive heading that may not even be related to the volume of searches and that even users are not necessarily aware of.
Below, you will see another Google Patent to show the contextual relevance for augmented queries and possible related search activities.
“Including every related entity with their contextual connections while explaining their core” is of Utmost Importance in Semantic SEO.
- Stop Giving Weightage on Keyword Volume or Difficulty
- We weren’t intimidated by reputable competitors with a tonne of backlinks when the project first started.
- Third-party metrics like keyword difficulty didn’t interest us.
- We were not alarmed by the competitors’ brand power or historical data.
- The phrase “We just used Google Search Console to show my client the latest situation of projects” was avoided at all costs. We only entered GSC to review Google’s responses.
If a subtopic is necessary for an article’s semantic structure, it should be written. Even if there is a “0” search volume, it should still be written. Even if the keyword difficulty is 100, it needs to be written.
Here, another crucial point needs to be made.
All phrases and every detail in all related topics in a topical map must be included if you want to be ranked first in the SERP for a “phrase.” In other words, without thoroughly processing each related topic, it is not possible to use semantic SEO to see an improvement in rankings in searches related to that topic.
Word Count Evaluation by page depth. The older the content gets, the page click depth increases on this example since we don’t use a standard internal navigation. But even in the 10th depth, we have stronger content than our competitors. This encourages Google to look further and deeper.
- Topical Coverage And Authority With Historical Data
A topical graph displays which topics are interconnected within which connections. How well you cover this graph is referred to as topical coverage. Historical data is the length of time you have been studying this particular topical graph at a particular level.
Topical Coverage * Historical Data = Topical Authority
Because of this, every graph I show you shows “rapid growth” after a predetermined amount of time. Additionally, because I use natural language processing and understanding, featured snippets are the main source of this initial wave-shaped rapid growth in organic traffic.
If you can take featured snippets for a topic, it means that you have started to become an authoritative source with an easy-to-understand content structure for the search engine.
Final Thoughts
We have done my best to keep the writing of this guide for this SEO case study with four different SEO projects as simple as possible. And I’ve been completely honest in everything we have said.
Thanks to deep learning and machine learning, semantic SEO will soon become a more popular strategy. And I believe that technical SEO and branding will give more power to the SEOs who give value to the theoretical side of SEO and who try to protect their holistic approach.
⭐️Lexical Semantics, Micro Semantics and Semantic Similarity in SEO and its Impact
What is Lexical Semantics?
Lexical Semantics is branch of linguistics that studies the different relationships between words. The different types of words relationships include:
- meronyms(parts of a whole)
- holonyms (wholes that contain parts)
- antonyms (opposites)
- synonyms (similar meanings)
- hypernyms (general categories)
- and hyponyms (specific examples)
⭐️What is Micro Semantics?
Micro Semantics is a subfield of Lexical Semantics that studies the meaning of words in a specific context. For example,
the word “dog” can have different meanings depending on the context in which it is used. In the sentence “The dog is barking,” the word “dog” refers to a specific animal. However, in the sentence “I’m a dog person,” the word “dog” refers to a type of person who loves dogs.
Here are some of the key concepts in micro semantics:
Sense: A sense is a specific meaning of a word or phrase. For example, the word “bank” has multiple senses, such as “a financial institution” and “the sloping ground alongside a river or lake.”
Reference: Reference is the relationship between a word or phrase and the object or concept that it refers to. For example, the word “dog” refers to a four-legged mammal that is often kept as a pet.
Denotation: Denotation is the literal meaning of a word or phrase. For example, the denotation of the word “dog” is “a four-legged mammal that is often kept as a pet.”
Connotation: Connotation is the emotional or cultural associations that are associated with a word or phrase. For example, the word “dog” has positive connotations of loyalty and companionship, while the word “cat” has negative connotations of independence and aloofness.
⭐️Semantic Similarity
Semantic Similarity is used to determine the macro and micro contexts of a document or webpage. It refers to how close or relevant two words are to each other. Semantic search engines, which use natural language processing and understanding, rely on these relationships and the distance between word meanings to work effectively.
The Methodology or SEO Applications of these are as below:
- Understanding the Distance between Words as Vectors.
- Creating the sentence structures for the questions and the answers.
- Matching the answers and the questions to sharpen the context.
- Using accurate information with different forms and connections.
⭐️What are the Different Lexical Relations Between Words
Lexical relations between words involve various types of connections, such as superiority, inferiority, part-whole, opposition, and sameness in meaning. The relationship between words can determine their context within a sentence and impact the Information Retrieval (IR) Score, which measures the relevance of content to a query. Having a clear and well-structured lexical relation helps increase the IR Score, indicating better relevance and potential user satisfaction.
IR Score Dilution and How To Avoid It?
IR Score Dilution occurs when a document covers multiple topics, leading to diluted relevance and lower rankings compared to more focused documents.
To avoid it authors must lexical relations and word proximity should be properly utilized within the document, with closely related words appearing in close proximity to each other within paragraphs or sections.
Search engines can check if a document contains the hyponym (a word with a narrower meaning) of the words in a query and generate query predictions from the hypernyms (words with broader meanings). They can also examine anchor texts to determine the hyponym distance between different words.
⭐️How is it Significant for Search Engines?
Lexical and Microsemantic relations work as semantic annotations for a document. These outline the main entity and accurately define the context of the document. These semantic annotations ultimately aid in matching a document to a query and contribute to a higher IR Score.
- Search engines can generate phrase patterns based on the lexical relationships between words in queries or documents.
- These patterns define concepts with qualifiers, such as placing a hyponym just after an adjective or combining a hypernym with the antonym of the same adjective.
- Recurrent Neural Networks (RNNs) often employ these connections and patterns for next-word predictions.
- This enhances a search engine’s confidence in relating a document to a specific query or understanding its meaning.
In other words, search engines can use the relationships between words to generate patterns that can be used to predict the next word in a sequence. This can be used to improve the accuracy of search results, as the search engine can be more confident that a document is relevant to a query if it contains words that follow a similar pattern.
To understand Lexical Relations, the types of lexical semantics between words should be seen.
Hypernym: The general word of another word. For example, the word color is the hypernym of red, blue, and yellow.
Hyponym: The specific word of another general word. For example, crimson, violet, and lavender are hyponyms for purple. And, purple is the hyponym for the color.
Antonym: The opposite of another word. For example, the big is the antonym of the small, and the early is the antonym of the late.
Synonym: The replacement of another word without changing the meaning. For example, huge is the synonym for big, and initial is the synonym for early.
Holonym: The whole of a part. For example, the table is the holonym of the table leg.
Meronym: The part of an entire. For example, a feather is the meronym of a bird.
Polysemy: The word with different meanings such as love, as a verb, and as a noun.
Homonymy: The word with different meanings accidentally, such as bear as an animal and verb, or bank as a river or financial organization.
⭐️Use of Micro Semantics and Lexical Semantics in Semantic Role Labelling
Both Micro Semantics and Lexical Semantics help in understanding the accurate meaning and Context behind words.
Semantic Role Labeling is the process of assigning roles to words in a sentence based on their meaning. These two tasks are interconnected, as Lexical Semantics can be used to help with Semantic Role Labeling.
For example, the words “door” and “close” can be used in different ways. In the sentence “The door is closed,” the word “door” is the patient, or object, of the verb “close.” In the sentence “George closed the door,” the word “George” is the agent, or subject, of the verb “close.”
Lexical Semantics can help with Semantic Role Labeling by providing information about the meaning of words. For example, the word “door” is typically associated with the concept of a doorway, which is a physical opening in a wall. This information can be used to help determine the role of the word “door” in a sentence.
In addition, Lexical Semantics can be used to identify relationships between words. For example, the words “door” and “close” are semantically related, as they are both related to the concept of a doorway. This information can be used to help determine the role of the word “door” in a sentence.
The same verb “close” can also be connected to another noun, such as “eyes.” In this case, a search engine can analyze the co-occurrence of “close” with “door” and “eye” using a co-occurring matrix. “Closing eyes” and “Closing doors” represent different contexts, even though the word “close” is relevant to both. Generating word vectors and context vectors is valuable for tasks like next-word prediction, query prediction, and refining search queries.
A search engine can adjust its confidence score for relevance based on the semantic role labels assigned to words and the lexical-semantic relationships between them in a text.
Here is a simple explanation of how Micro Level Semantics can help with Semantic Role Labeling:
- Micro Semantics and Lexical Semantics can help to identify the meaning of words.
- The meaning of words can be used to determine the role of a word in a sentence.
- For example, the word “door” can be used as a patient or an agent, depending on the context.
- Semantics can also help to identify relationships between words.
- These relationships can be used to determine the role of a word in a sentence.
- For example, the words “door” and “close” are semantically related, as they are both related to the concept of a doorway.
⭐️Steps to Use MicroSemantics and Using a Large Language Model to Improve Contextual Coverage To Rank High?
Before diving into the methodologies and basic concepts let us show you some examples of results that have been driven due to the procedures of semantic SEO.
Last 6 Months GSC Data for a9-play.com
Last 12 Months Growth Data for upperkey.com
Here we are going to create a fresh content draft and we are going to break down the exact implementations of micro semantics in the creation of the draft.
In this case we are trying to rank a website whose Source Context: Handmade Lifestyle Products.
The Central Topic in this Case is Aromatherapy.
First we set out to create a Topical Map that covers the Topic Entirely:
Here is an example of a Topical Map for a Particular Entity. Although in this article we won’t be going over the specific steps for building a topical map, but basically a topical map consists of a hierarchical list of topics and subtopics and is used to establish a topical authority on a particular subject.
Each Subject under the Topical Map defines the Macro Context of the specific subject.
In this article we would try to define the content brief for the Macro Context : Aromatherapy Benefits
Each contextual Brief contains 4 sections, The Contextual Vector(SubTopics), Headings Levels, Article Methodology and Query Terms.
For each content brief we find out the top two ranking competitors and find out the ranking terms for the exact webpage:
⭐️Step 1: Defining the Query Terms (Query Network)
In order to make the process easier use a Large Language Model like ChatGPT to input all of the ranking terms of the top ranking competitor Websites.
Then we try to verbalize them by Asking ChatGPT to extract all the relevant entities and questions that contextually cover the above query list.
The Desired Response is Obtained Below:
As you can see the Relevant Entities and the Verbalized Questions have been outputed by ChatGPT.
The same process is repeated for the other competitor. At the end you get a complete list of Questions and Entities that can completely cover or are related to the Topic : Aromatherapy Benefits.
All these queries can be incorporated in the Query Column for further Usage.
⭐️Step 2: Turning Queries into Heading
Now that we have a complete set of Unique Queries that completely define all the relevant entities for the topic: Aromatherapy Benefits, its now time to write the Article Outline.
In order to write the Headings we must understand the following terms:
Entity: The central concept or topic of the heading.
Attribute: A Property that defines the entity in a specific context. Eg: Height, Size, Width, Cost etc.
Value: Value represents the meaning of the text.
While Turning the Queries into Headings two things can be kep in mind:
- Group Similar Questions and find out the Representative Query and the Variations
Eg: Word 1 + Aromatherapy + Word 2 + Benefits + Word 3
Word 1 + Benefits + Word 2 + Aromatherapy + Word 3
This is a query template where Aromatherapy and Benefits are the main context words. Any query that only consist of these two terms is a representative query.
Eg: What are the benefits of Aromatherapy.
Word 1, Word 2 and Word 3 are variation terms that changes original query and defines in different micro contexts (MicroSemantics).
For Eg:
Here frankincense, lemongrass, vanilla, are different essential oils extracts and is more closely related to the word benefits and hence changes the query meaning in the context of the specific essential oil.
Identifying all of the Word variation and hence the contextual variations of a queries is important to effectively write unique headings that will cover the entire query network.
- Write Down the Headings in a Logical Hiearchy that completely covers the Query Network
Here two things should be kept in mind:
- The Headings must be relevant to the source context , here Aromatherapy Benefits.
- The Headings must cover the query network.
- The Headings must be written in a logical order to maintain the contextual flow.
Accordingly the headings are written. Notice How each of the Entities are Defined in the list format.
⭐️Step 3: Defining the Article Methodology
Defining the Headings of an Article along with the appropriate Heading level is just half way in the journey to creating a content brief. The real contextual coverage is achieved by defining the contextual structure or in this case the article methodology (for writers).
In the article methodology portion we try to define the main headings of the article in different relevant micro context using the lexical semantics.
Let us understand this in a few examples:
While writing the article methodology for the 13 Health Benefits of Aromatherapy heading we went on to define Aromatherapy and then cover the various physical and psychological benefits. However in the later section we mentioned the authors to also the common diseases of Aromatherapy. Here diseases is used in a different micro context to benefit but still relevant to the overall topic, as knowing the overall benefits also requires the knowledge of the potential diseases that it can cure.
Also we have used another context i.e “Common side effects of Aromatherapy”, side effect being a antonym variation of the term “benefits” but still contextually relevant to the overall subject.
Hence one can understand the application of both micro contexts (microsematics) and lexical variations (lexical semantics) in ensuring a contextual coverage for a given topic.
Let us take another example.
Under the Benefits of Aromatherapy we have the H2 Heading, called Enhances Immunity. Again we use lexical semantics to define this heading in different context.
One of them being defining the antimicrobial properties of certain essential oils, antimicrobe being a hyponym of immunity. Similarly the context of aromatherapy affecting white blood cell generation is another example for the use of using a hyponym variation in the same context.
In the last heading, we try to cover a body immunity in a different context by using the term vulnerabilities, being an antonym variations.
These small changes in the context of the writing while staying relevant to the overall macro context of the heading helps in improving the relevancy of the article passages to the overall macro context.
⭐️Using Micro Context in Supplementary Context
Each Content Brief in a Topical Map contains a Main Content and a Supplementary Content along with a border question.
In the Supplementary section of the Content we used a Micro Context: Side Effects of Aromatherapy which is a seperate micro context although still remaining contextually relevant to the main topic.
One can link this section to a relevant article about “Side Effects of Aromatherapy” and Vice Versa. Thus the use of micro semantics in the Supplementary content helps in effective interlinking thus deepening the link coverage of the overall topical map.
In conclusion, exploring the realm of micro and lexical semantics and harnessing the power of large language models has proven to be a game-changer when it comes to enhancing document relevancy for search engine optimization (SEO). The synergy between these two areas opens up new possibilities for understanding and leveraging the intricacies of language in order to optimize content for better visibility and user engagement.
⭐️Final Thoughts
Micro semantics delves into the fine-grained analysis of individual word meanings, encompassing aspects such as word senses, synonymy, antonymy, and semantic relationships. By understanding the subtle nuances and context-dependent nature of words, we can craft content that aligns more precisely with user intent. This enables us to optimize on-page elements, such as headings, meta tags, and content structure, with a focus on relevant keywords and their semantic variations.
Furthermore, lexical semantics explores the broader network of word meanings and their interconnections within a language. Building upon the foundation of micro semantics, it allows us to dive deeper into the semantic relationships between words, such as hyponymy, meronymy, and troponymy. By incorporating this knowledge, we can develop content strategies that not only incorporate primary keywords but also encompass related terms and concepts that enhance the overall topical relevance and authority of the document.
⭐️Understanding Entities and Entity Oriented Search and Its Significance in Ecommerce SEO
Entity-oriented Search Understanding is an important part of Search Engine Understanding or Search Engine Communication. These terms might be new to the traditional understanding of SEO, but the process of understanding a search engine is a daily routine for any SEO to analyze the search engines’ decision trees that create their result pages.
Entity-oriented Search Understanding is the understanding of a SERP Instance based on entities, their types, attributes, and connections to each other. A search engine might choose only certain types of pages that include a type of entity along with particular attributes and phrase variations for these attributes with the most related facts. Or, a search engine might filter the results according to the sources’ N-Grams for certain entities. If they don’t have enough numbers, or if they don’t have the relevant facts and external references for these entities, they might be outranked.
⭐️Topical Authority and Entity Search
Topical Authority overall is calculated by the relevance of a information source to a topic by proposing a link value to a search engine by satisfying users via the queries that seek answers for certain entities with certain context.
⭐️Steps to Improve Topical Authority By Entity Oriented Search Understanding
To improve the topical authority, a web page must cover all the related details to a topic , with a certain context, query and intent template. In this case its the information gap that matters and not the keyword gap.
The following methodology can be followed:
- Compare the entities within different web pages.
- Compare the context and content angle for these entities.
- Compare the facts, prepositions, and semantic role labels for these entities.
- Compare the questions on the competing web pages.
- Compare the Site-wide and Page-level N-Grams of the web pages.
- Compare the web page layout of the web pages (web page design can affect the meaning and context of the entities within the web page)
- Compare the anchor texts from outgoing, and incoming links for these web pages.
- Take all the attributes of the specific entity, and give them an order based on the relatedness of the attribute for the source, and the popularity of the attribute to generate better questions.
- Use a clear sentence structure for all the prepositions.
- Do not dilute the context of the web page with irrelevant opinions, or analogies, and other types of entities.
- Process the same entity or same entities from the same type with the same context from start to end.
⭐️How to Practically Improve Topical Authority for Ecommerce Sites Using Entity Oriented Search Understanding?
To use topical authority for e-commerce sites with entity-oriented search understanding, a website should create content that is rich in factual information about the products and services that they sell. This content should also be structured in a way that makes it easy for search engines to understand the entities that are mentioned.
For example, an e-commerce site that sells electronic bikes could create content about different types of electronic bikes, their features, their benefits, and their uses. This content could also include information about the history of electronic bikes, the different brands of electronic bikes, and the different ways to maintain electronic bikes.
It’s essential to consider different types of queries, including possible search intents, correlated queries, sequential queries, and entity-seeking queries. By incorporating these query themes into the content, the e-commerce site can cover various knowledge domains and provide a comprehensive and relevant user experience.
In summary, to leverage topical authority for e-commerce sites, it’s crucial to offer detailed information about products and related aspects, while ensuring the content satisfies different search intents and addresses a wide range of user queries.
In Summary, the following methodology can be followed
- Understand the dimensions of the product that you sell.
- Find all the relevant entities for the product, including its brand, material, inventor, alternatives, and similar.
- Generate the best proper questions for these dimensions of the product, brands, related entities, and their attributes.
- Give the questions a proper order based on the web page layout, and web page purpose.
- Match the query and answer format with NLP convenient sentence structures.
- Use information redundancy, and unique value opportunities for the products.
- Connect all the entities based on their ontology for commercial purposes.
- Understand the popularity of entity attributes and the relatedness of entity attributes.
- Try to use entity relations, relation types, semantic role labeling, and entity resolution from the eyes of the search engines.
- Use phrase templates, and phrase pattern taxonomies, and create a prominence hierarchy without diluting the context.
- Search Engines’ perspectives on a topic and the central context of the topical map should align with each other to make search engines understand the website easier, and faster.
⭐️How to Understand Which Entity Attributes are More Prominent over the other?
To determine which attributes of an entity are more important for a given context, we consider the prominence, relatedness, and popularity of those attributes.
- Prominence refers to how often an attribute is mentioned in a particular context.
- Relatedness refers to how closely an attribute is related to a particular topic.
- Popularity refers to how often an attribute is searched for.
To understand which entity attributes are more important for a context, an entity-oriented search analyst should consider the following factors:
- The source’s context: What is the source about? What are the most common attributes of entities of the same type in this context?
- The source’s purpose: What is the source trying to achieve? What information does the source need to provide to achieve its purpose?
- The user’s intent: What is the user trying to find out? What information does the user need to answer their question?
Once the analyst has considered these factors, they can identify the most important entity attributes for a particular context. These attributes can then be used to generate questions, answer questions, and create content that is relevant to the user’s needs.
For example, in a source about Formula One, important attributes of a car would include the driver, constructor, engine, top speed, and weight. However, in a historical context, attributes like the inception of cars or the inventors would be more significant. The most common attributes among entities of the same type in a given source are typically the most essential.
To identify attributes that matter and generate relevant questions, search analysts consider the relatedness and prominence of the attributes. For instance, in a Formula One-focused source, attributes like the car’s driver and race circuits would be more prominent than attributes such as lap count or circuit viewer capacity. Certain attributes may also have higher popularity, and understanding search-demand trends and changes can help improve the ranking of documents, particularly in news-focused contexts.
⭐️How to Strengthen Contextual Signals and Relevance by Connecting Entities
To strengthen contextual signals and relevance, we can connect entities to each other using ontology and knowledge graphs. By forming triples of related entities, we create connections that help build a knowledge graph. This graph includes factual information and improves the relevance of content for specific queries.
Semantic annotations, which are labels assigned to documents based on named entity recognition, play a role in connecting entities within a context. These annotations indicate the weighted attributes of an entity as a subject or an object. The switches between entities and attributes change the semantic annotations, creating internal links with definitive relevance.
For example, consider the entities
- Germany,
- France,
- England,
- Turkey, and the
- United States,
all of which are countries. These entities share common attributes related to their status as countries.
By understanding the attribute hierarchy or semantic dependency tree, we can determine the priority of attributes in defining an entity. If a web page discusses attributes like currency, banks, and finance, a search engine will recognize the topic as international finance and retrieve relevant information and questions to satisfy user needs. Conversely, if the page includes terms like education, schools, and classrooms, the context will shift to education in these countries.
Creating entity connections involves using mutual attributes to establish relevance within a specific context, enabling ranking signal consolidation. For example, Germany can be connected to Turkey based on currency exchange rates or shared population features. Multiple connections and variations exist between these entities, such as Turkey’s connection to England for external debts or Germany’s connection to the United States for the dollar index. These connections and their permutations define the relevance and factuality of a web page in relation to user queries.
⭐️How to Specify Context and Define Entities Accurately
An Entity is a real word object. However its semantic definition can change based on the context. For example, a tree can be a plant in the context of city planning, but it can also be a mythological creature or a material for bridges.
To improve the precision and factual information redundancy of a source, entities should be defined with their functions, importance, usage, benefits, and effects for the specific knowledge domain.
If an entity’s differences, unique and similar sides, alternatives, and advantages are absent within the web page, or if they are not being able to select easily, the web page might dilute its context, relevance, and informational value for the search engine’s re-ranking, and initial ranking algorithms.
Question answering is an important part of the entity-oriented search. Google can choose multiple possible answers for a specific question. A contextual domain can be determined by a single qualifier, such as a year, place, or demographic group. In this context, the search engine can choose the best web page with the best answer coverage.
⭐️An Example of Entity Oriented Search To Boost Ecommerce Website SEO?
To explain the benefits of Entity Oriented Search can be understood using the following example of an Ecommerce Website and how they implemented it successfully to boost their organic reach.
In this case we are reviewing the following website: https://www.bkmkitap.com/
This is a Books Aggregator in Turkey. Although they had good branded search traffic their organic traffic was failing.
The site had a tremendous amount of technical SEO issues:
Overall here are the list of Technical and Pagespeed related problems:
- Half of the website URLs don’t exist in the sitemaps
- There are millions of cannibalized URLs.
- There are thousands of duplicate product URLs.
- More than 30000 internal 404 pages. (From full data)
- Blocked URLs within the Sitemap.
- Hundreds of 5XX errors daily
- Submitted URLs with Noindex
- Redirection Errors
- Submitted but 404 URLs
- Indexed but blocked URLs (Tens of thousands)
- Indexed content without actual content
- More than half a million robots.txt excluded pages.
- Nearly 100,000 URLs are crawled and not indexed.
- Nearly 53.224 pages are currently discovered and not crawled.
- Over 600,000 duplicate with canonical and submitted URL is not selected as canonical.
- The site has millions of URLs, but even a single URL doesn’t pass the Core Web Vitals.
- Most of the website has poor scores on the PSI.
- Thousands of AMP Related issues such as referenced AMP URL is not an AMP, or custom javascript, etc.
- Thousands of structured data errors, and missing information for the related products.
- Hundreds of thousands of products without stock information, or stock existence. These last two subjects also affect the search engine’s confidence to rank the specified e-commerce page, since the stock information, brand, reviews, and prices are not clear enough for the evaluation algorithms.
With Technical SEO, Page Performance fixing out of option, the website had to focus on entity SEO and Semantics. To implement Entity Oriented SEO, the SEO analyst have to understand the context of the source and then using the context to improve the Site’s Ranking.
⭐️Step By Step Methodololgy To Improve the Ecommerce Category and Product Relevancy
- For BKMKitap, the overall context of the website was “Book Ecommerce”.
- To cover both informational and e-commerce-related contextual domains, the webmasters identified the most relevant attributes for both angles.
- The webmaster used ontology and taxonomy to connect the e-commerce and informational domains.
- When it comes to books, as a product, it has “size, material, author, ISBN Number, price, page count, an image or visual for cover, editor.”
- When it comes to books as a literature value, it has “an effect on the subject that it processes, a topic, unique sides, differences, authors, characters, genre, era, style, school and more.”
- When an SEO understands these two sides of the entity as a product and artwork, the next step is search intent understanding.
- Search intent understanding is the process of understanding what a user is looking for when they perform a search.
- In the context of search intent understanding, an SEO should know that a web page should have a dominant context.
- In other words, a web page can’t be an e-commerce web page and an informational web page at the same time at the same level.
- One of these options dominates the other one for the specific web page, and the anchor texts or the web page layout should align with this option.
- In the BKMKitap.com SEO Case Study, the author created two different web page types, one for the books’ e-commerce side, and the other one for the books’ literature value along with their authors.
- An e-commerce web page can have an informational content piece, but if this content is about “buying the book,” and “using the product” along with “refund and delivery policies and conditions,” it would improve the search intent coverage for the related web page.
How Did They Improve the Contextual Relevance and Topical Authority with Informational Content and Commercial Intent?
Improving the Contextual Relevance and Topical Authority of a Website, an SEO should cover the informational, definitional, and factual hinterland of the topics for the specified products.
For a book Ecommerce, this would mean creating separate webpages based on the following topics:
- Books Genres
- Books from Different Geographies
- Authors from Eras
- Authors from Geographies
- Authors from Cultures
- Authors from Ideologies
- Individual Author Biographies
- Author and Book Connections
- Author’s Similarities, Differences, Thoughts, Childhood, and more.
⭐️Here’s a Screenshot of a Semantically Engineered Page Content
Here are some additional details about each of the strategies mentioned above:
Creating different web page groups for different entities: This allows the SEO to focus on specific topics and provide more in-depth information about each one. This can help to improve the ranking of the website in search results for those specific topics.
Generating questions: This can help to improve the user experience by making it easier for users to find the information they are looking for. It can also help to improve the ranking of the website in search results by making it more likely that users will click on the website’s links.
Creating context-sharpening entity-oriented search documents: This involves creating documents that provide information about different entities and how they are related to each other. This can help users to understand the relationships between different entities and to find the information they are looking for more easily.
Connecting all the relevant facts to each other: This involves creating links between different pieces of information on the website. This can help users to find the information they are looking for more easily and can also improve the ranking of the website in search results.
Here’s an example of a Semantically Engineered page Content for BKMKitap.com
The Topic is about e-YDS an Academic Personnel and Graduate Education Examination. The following headings were covered:
What Is ALES?
What Does ALES Do?
How to Apply for ALES?
Who Can Enter ALES?
How Many Minutes Is ALES Exam?
Which Courses Are Available in ALES?
What are the Course Topics in ALES?
How is ALES Score Calculated?
In ALES, Does Wrong Lead to the Right?
How Many Nets Should Be Made to Calculate ALES Score?
What are ALES Score Types?
How Many Times Will ALES Be Performed in 2023?
When is the 2023 ALES Exam?
When will the 2023 ALES Exam Results Be Announced?
How Long Is the Validity Period of the ALES Score?
How to Study for ALES?
One can notice the Question Answer Format used to write the content. Also the different types of context and content angles used to write the article.
Here is the result:
Here is How They USed Semantic Engineering for a Product Category Page
This is a Category Page for Harry Potter Books
Notice how they added more content at the buttom of the page product page to improve the contextual relevance.
Now here is the result
Overall Website Organic Performance Over Time
⭐️So What did the Entity Oriented Search Optimization Really Do?
- Focusing on more educational topics, and contexts.
- Covering educational books, lectures, research, and researchers.
- Focusing on university materials, professor, and their work.
- Focusing on exams from different educational layers and levels along with their books.
- Connecting the educational need materials to the stationary e-commerce pages.
- Extending the contextual coverage to the scientific research and study topics.
- Extending the contextual coverage to the school lectures, their topics, and necessary books.
- Focusing on the school ages, and school-related children’s books.
- Extending the coverage to the student requires, and student lifestyle.
- Increasing the coverage for the internal links, and anchor text for a better-directed graph over all of these context domains.
Leveraging Entity Recognition to Enhance Micro Semantics in SEO
Entity recognition is a powerful technique in the realm of SEO that plays a crucial role in enhancing micro semantics. It involves identifying and classifying key entities such as people, places, organizations, events, and concepts within a given text. By incorporating entity recognition into SEO strategies, websites can achieve better understanding and contextual relevance in search engine algorithms, ultimately improving their search visibility and rankings.
What is Entity Recognition?
Entity recognition, also known as Named Entity Recognition (NER), refers to the process of detecting and categorizing specific pieces of information (entities) within a text. For instance, in a sentence like “Albert Einstein was born in Ulm, Germany,” entity recognition identifies “Albert Einstein” as a person and “Ulm, Germany” as a location. It plays a vital role in natural language processing (NLP) systems, enabling search engines to understand the relationships between different entities mentioned in web pages.
The Role of Entity Recognition in Micro Semantics
Micro semantics in SEO focuses on the deep, granular understanding of words and their relationships within a piece of content. Traditional keyword-based SEO tactics are often not enough to fully capture the meaning behind content, especially when it comes to long-tail queries or more complex search intents. This is where micro semantics comes into play, focusing on the contextual relationships between words.
Entity recognition can significantly enhance micro semantics by providing search engines with a structured understanding of the content. By identifying the key entities within a webpage, search engines can better comprehend the topic, relevance, and relationships within the text. For instance, if a page is about “SEO techniques,” entity recognition can identify terms like “Google,” “algorithm,” “ranking factors,” and “content marketing” as relevant entities. This allows search engines to rank the page higher for queries that relate to these entities.
How Entity Recognition Enhances Search Engine Understanding
Search engines like Google have evolved significantly over the years, moving from simple keyword matching to more advanced semantic understanding. Google’s Knowledge Graph, for example, is built on entity recognition and helps the search engine connect the dots between various pieces of information. By understanding the relationships between entities, search engines can provide more accurate and relevant search results.
When a search engine detects entities within a webpage, it can place that content within a broader context. This contextual understanding enables the search engine to deliver rich results, such as knowledge panels, rich snippets, and other enhanced search features. For example, if a user searches for “Barack Obama,” the search engine can pull up a knowledge panel that includes information about his career, achievements, and personal life, based on entities it has recognized in the content.
Improving Content Relevance and Visibility
Incorporating entity recognition into SEO strategies helps create content that is more relevant and comprehensive, ultimately improving visibility in search results. Here’s how it works:
- Content Clarity and Structure: By identifying key entities within the content, you can ensure that your content is structured around relevant and authoritative terms. This helps search engines clearly understand the subject matter of the page and improves its chances of ranking for relevant queries.
- Targeting Specific User Intent: Entity recognition enables SEO experts to align content with user intent. By understanding the entities associated with a query, you can tailor your content to address specific needs. For example, if a user is searching for “best practices for SEO in 2024,” entities such as “SEO tools,” “content optimization,” and “on-page SEO” can help guide the content to provide specific, valuable insights.
- Boosting Topical Authority: When search engines recognize the relevant entities in your content, they associate it with a specific topic or industry. This contributes to the overall topical authority of your site. As search engines connect your content to well-established and authoritative entities, it can improve your ranking for queries related to those entities.
Entity Recognition and Structured Data
One way to leverage entity recognition is through structured data markup. Structured data, such as Schema.org, helps search engines understand the context of the entities within a webpage. By using structured data to label entities like products, reviews, authors, and events, you provide search engines with more explicit context, which can improve content visibility in search results.
For example, if you’re writing a blog about a book, you can use structured data to mark up the book’s title, author, publisher, and release date as entities. This allows search engines to display rich snippets that highlight this specific information, making your content stand out in the search results.
Supercharging SEO with Entity Recognition: The Key to Mastering Micro Semantics
Leveraging entity recognition in SEO is a smart strategy for improving micro semantics and enhancing search engine understanding. By identifying and classifying entities within content, websites can improve the accuracy, relevance, and visibility of their content. This approach aligns with search engines’ evolving focus on semantic search, helping websites deliver better user experiences and gain a competitive edge in search rankings. As search engines continue to refine their algorithms, integrating entity recognition into SEO practices will only become more essential for achieving long-term organic growth.
⭐️Final Thoughts on Entity Oriented Ecommerce SEO
- Entity-oriented search understanding (EOSU) is a technique that uses entities to understand the meaning of search queries and to create content that is relevant to those queries.
- EOSU is not as popular as AI text generation, but it is becoming increasingly important as search engines become more sophisticated in their ability to understand natural language.
- EOSU can be used to improve the ranking of websites in search results by creating content that is more relevant to search queries.
- To implement EOSU, SEOs should:
- Create hyper-structured data, which is data that is organized in a way that makes it easy for search engines to understand.
- Perform experiments with different sentence structures to extract different information from text.
- Use A/B testing to compare the performance of different EOSU strategies.
EOSU is a powerful technique that can be used to improve the ranking of websites in search results. By understanding entities and their relationships, SEOs can create content that is more relevant to search queries. This can lead to more traffic and more conversions.
Thatware | Founder & CEO
Tuhin is recognized across the globe for his vision to revolutionize digital transformation industry with the help of cutting-edge technology. He won bronze for India at the Stevie Awards USA as well as winning the India Business Awards, India Technology Award, Top 100 influential tech leaders from Analytics Insights, Clutch Global Front runner in digital marketing, founder of the fastest growing company in Asia by The CEO Magazine and is a TEDx speaker.