Get a Customized Website SEO Audit and Online Marketing Strategy and Action Plan
The purpose of this project is to use artificial intelligence (AI) and deep learning techniques to analyze and optimize images on a website for SEO (Search Engine Optimization). By enhancing images in this way, the project aims to make them more discoverable by search engines like Google, improve accessibility for users (especially those who rely on screen readers), and ultimately help drive more traffic and visibility to the website.
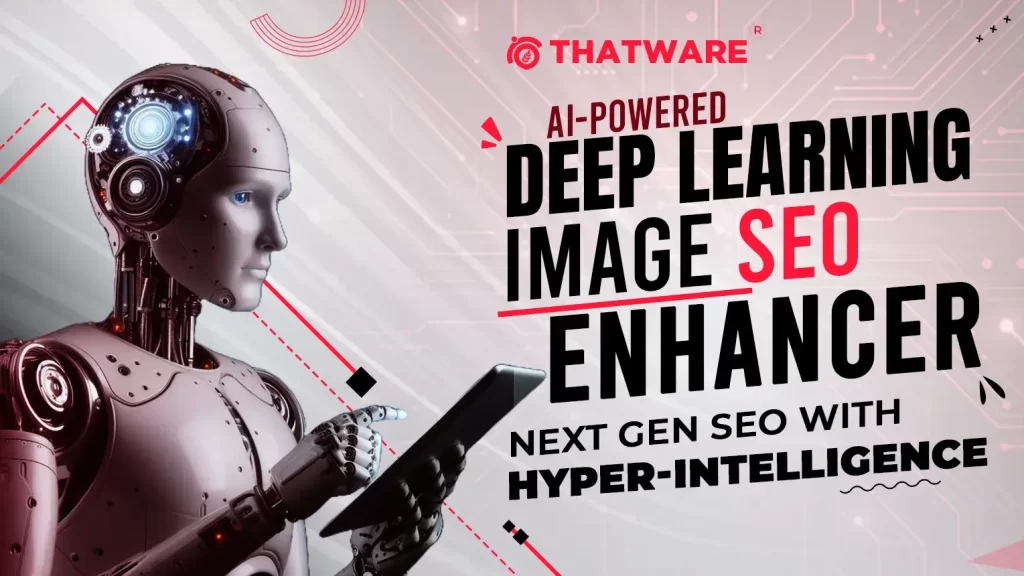
To understand why this is important, let’s break down some key terms:
- Search Engine Optimization (SEO): This is the process of making a website more visible to people who are searching for relevant content using search engines like Google. For images, this involves using keywords, descriptions, and other elements that help search engines understand what the image is about.
- Deep Learning: This is a form of AI that mimics the way the human brain works. It can learn from large amounts of data to recognize patterns and make decisions. In this project, it helps analyze and understand the content of images.
Detailed Breakdown of the Purpose:
1. Automatically Generate Tags for Images:
- What this means: The deep learning model analyzes each image and generates descriptive tags (keywords) that represent what the image contains. For example, if the image is of a “book jacket,” the model might generate tags like “book,” “cover,” and “jacket.”
- Why it matters: These tags help search engines better understand what the image is about, making it easier for people to find the image when they search online. This can increase website traffic.
2. Improve Alt Text for Images:
- What this means: Alt text (alternative text) is a description of an image that is displayed when the image cannot be shown. It is also used by screen readers to describe images to visually impaired users.
- Why it matters: Many images on websites either have no alt text or have alt text that is not very helpful. This project uses AI to automatically create meaningful and descriptive alt text for each image, improving accessibility and helping search engines understand the image’s content.
3. Categorize Images Based on Content:
- What this means: The model groups images into categories based on what it “sees” in the image. For example, if the model detects that an image shows a book cover, it might categorize it as “Books.”
- Why it matters: Categorizing images makes it easier to organize and display them on a website. It also helps users find related images more easily and can improve the overall user experience.
4. Enhance Metadata for SEO:
- What this means: Metadata is additional information about an image, like its tags, alt text, and category. This project enriches the metadata with detailed descriptions that are optimized for search engines.
- Why it matters: Enhanced metadata improves the chances of an image appearing in search engine results, driving more traffic to the website. It also helps search engines better understand the context of each image, making them more relevant for users.
Practical Benefits for a Website Owner:
- Increased Visibility: Optimized images are more likely to appear in search engine results, which can attract more visitors to the website.
- Better User Experience: Improved alt text and image categorization make the website more accessible and user-friendly, especially for visually impaired users.
- Higher Engagement: When users can easily find and understand images, they are more likely to engage with the website, leading to higher retention rates and potentially more sales or conversions.
- Streamlined Image Management: Automated tagging and categorization make it easier to manage large image libraries, saving time and effort.
Deep Learning for Image SEO:
What is Deep Learning for Image SEO?
Deep Learning for Image SEO (Search Engine Optimization) focuses on using advanced AI models to automatically recognize, tag, and enhance the discoverability of images on websites. This is achieved using a form of deep learning called Convolutional Neural Networks (CNNs), which are especially effective in processing and understanding visual content like images. CNNs can learn to detect patterns, features, and objects within images, allowing for improved labeling, categorization, and overall SEO optimization, meaning your website images become more searchable and rank higher on search engines like Google.
Use Cases of Deep Learning for Image SEO
- Automatic Image Tagging: Using CNNs, images on a website can be automatically tagged with relevant keywords based on what the AI identifies. This tagging can make images more accessible in search results, boosting the website’s traffic.
- Enhanced Image Descriptions (Alt Text): CNN models can generate or improve descriptive alternative text for images, enhancing accessibility and SEO ranking.
- Content-Based Image Retrieval: For websites with large image databases (e.g., e-commerce sites), deep learning can enable better user search results based on the image’s content, leading to more relevant suggestions.
- Image Categorization: Deep learning can group and categorize images based on recognized features (e.g., clothing type in a fashion store) to optimize user navigation and content discovery.
- Image Quality Enhancement: Techniques like image super-resolution or noise reduction can enhance image quality for better user experience and SEO.
Real-Life Implementations
- E-Commerce Websites: Sites like Amazon or eBay use image recognition to automatically tag product images, making them searchable by features such as color, shape, or product type.
- Social Media Platforms: Platforms such as Instagram use deep learning to recommend relevant hashtags or group similar content.
- Search Engines: Googleās reverse image search relies heavily on deep learning models like CNNs to understand and categorize images based on visual content.
Website Context Use Case Explanation
For website owners, Deep Learning for Image SEO can improve how images are indexed and discovered by search engines. Suppose you own an online store. By using a deep learning model, your websiteās images could be tagged automatically with terms like “red leather jacket” or “modern lamp,” based on their content. This makes the images more searchable and likely to appear in Googleās image results, driving more organic traffic to your site.
Data Requirements for Deep Learning for Image SEO
To train and use a Deep Learning for Image SEO model, the following data inputs are typically required:
- Image Files: The model needs access to the images you want to optimize (e.g., product photos).
- Labels/Tags: In some cases, labeled training data might be needed, especially when customizing the model for specific image categories.
- Webpage URLs (Optional): If you want the model to associate images with specific web pages or text content, URLs can be provided.
- CSV Files (Optional): CSV files can store metadata like image names, current tags, or related descriptions, which the model may use to enhance context during processing.
For a non-tech approach, data can be supplied as images directly or in CSV files with metadata. The model processes these inputs to produce outputs such as automatic tags, alt texts, and optimized metadata for images on your website.
Expected Output from the Model
The output from a Deep Learning for Image SEO model includes:
- Auto-Generated Tags: Relevant tags for each image, aiding in SEO.
- Improved Alt Text: Descriptive alt text that aligns with image content and enhances accessibility.
- Categorization Data: Grouping of images into categories, useful for better display and user navigation.
- Enhanced Metadata: SEO-rich metadata that improves image search rankings.
Practical Example
Imagine a website selling artwork. When you upload images of paintings, a deep learning model scans the image and generates tags like “abstract art,” “blue tones,” and “oil on canvas.” It can also create descriptive alt text such as “Blue abstract oil painting with textured strokes.” This makes it easier for search engines to understand and display your images in relevant search results.
Explanation of Labels/Tags
When I mentioned labels/tags, I was referring to descriptive keywords or categories that define the content of images. For example, an image of a red car could have labels like “car,” “vehicle,” “red color,” and “sedan.” These tags help the Deep Learning model understand what the image represents. The more accurately labeled data you provide during training, the better the model can learn and make predictions.
For non-technical users, hereās how you might provide labels/tags:
- Manual Labeling: You can manually assign keywords or categories to your images based on what they depict. This can be done in a spreadsheet (CSV file) where you list image names and their respective tags.
- Automatic Labeling with Pretrained Models: You can also use existing image recognition models to automatically generate tags for images, which can then be fine-tuned.
Collecting Data from Webpages with URLs via Web Scraping
If the Deep Learning for Image SEO model can collect image details from web pages by using URLs and web scraping. Yes, this is absolutely possible. Hereās how it can be done:
1. Web Scraping Process:
- You can use web scraping tools like BeautifulSoup (Python) or Scrapy to fetch the content of web pages, including images.
- The scraper extracts the image URLs, alt texts, and any other metadata associated with the images on your web pages.
- Example: Suppose you have a webpage URL. The web scraping code can extract all image elements (<img> tags) and gather the src (image URL), alt attributes (text descriptions), and other related data.
2. Feeding Data to the Deep Learning Model:
- Once the data is gathered, you can save it in a structured format (e.g., CSV file) with fields like image URLs, alt text, etc.
- The Deep Learning for Image SEO model can then access this data. You can either download the images locally using their URLs or use direct links if your model supports online processing.
- If necessary, additional preprocessing can be done, such as resizing images, converting formats, or extracting features.
How This Works Together
- Step 1: Use a web scraper to extract images and their metadata (alt text, descriptions) from your webpages using their URLs.
- Step 2: Save this extracted data in a structured way (CSV file or directly in your code).
- Step 3: Provide this data to the Deep Learning model. The model can access the images either by downloading them (if needed) or by using URLs to process and analyze them.
- Step 4: The Deep Learning model will then generate output like enhanced tags, optimized alt text, and other metadata improvements for each image, all of which help improve image SEO for your website.
Possible Challenges and Solutions
1. Web Scraping Challenges:
- Rate Limiting: Some websites may restrict scraping activity, so you need to ensure you respect their policies and use techniques like throttling requests.
- Dynamic Content: If your website uses dynamically loaded content (e.g., JavaScript), you may need more advanced scraping tools like Selenium.
2. Image Processing:
- Storage: Downloaded images can take up space, so consider whether you want local or online processing.
- Model Customization: Depending on your goals, you may want to customize your Deep Learning model to recognize specific features relevant to your websiteās niche (e.g., fashion, automobiles).
Applicability of Deep Learning for Image SEO on Non-Product Websites
Yes, a Deep Learning for Image SEO Model can still be useful for non-product-based websites like thatware.co, but its application and benefits will be slightly different from product-selling sites (e.g., Amazon, Flipkart). Here’s how and why it matters:
Why Deep Learning for Image SEO is Useful for Content Websites
- Improving Search Engine Visibility: Even though the images on a site like thatware.co are used to support content rather than sell products, they can still play a crucial role in boosting SEO. Search engines like Google index images and use them as part of how they understand and rank web pages. Therefore, optimizing these images with relevant tags, descriptions, and metadata can increase the chances of the website appearing in image searches, which can bring more traffic to the site.
- Accessibility: Descriptive alt text (alternative text) is essential for accessibility. Users with visual impairments who use screen readers rely on this text to understand the content of images. Improved alt text makes your website more inclusive and can positively impact its SEO rankings since search engines prioritize accessible websites.
- Contextual Relevance: Since the images on the website are meant to explain or enhance textual content, having accurate and contextually relevant tags and descriptions helps search engines better understand the content on pages. This can improve the relevance of web pages in search results.
Specific Outputs for thatware.co and Their Relevance
1. Auto-Generated Tags:
- Purpose: Automatically generating tags for each image ensures that each image has meaningful descriptors related to the content it supports. For example, an image on a page about “SEO strategies” might be tagged with terms like “SEO,” “digital marketing,” and “content strategy.”
- Relevance: This is useful for enhancing discoverability of images on your site in image search results.
2. Improved Alt Text:
- Purpose: Creating accurate and descriptive alt text for images makes the content accessible and helps search engines understand the relevance of the image within the webpageās context.
- Relevance: This is especially important for content-driven sites to ensure inclusivity and better SEO rankings.
3. Categorization Data:
- Purpose: Grouping images into categories (e.g., “infographics,” “graphs,” “illustrations”) can help organize visual content and make it easier for users to navigate content-rich pages.
- Relevance: While not as critical as for e-commerce sites, it still helps improve user experience, which can positively impact SEO indirectly.
4. Enhanced Metadata:
- Purpose: Adding SEO-rich metadata to images ensures that search engines can understand what each image is about and how it relates to the overall webpage content.
- Relevance: For sites like thatware.co, having relevant metadata increases the chances of pages being indexed accurately by search engines, boosting overall visibility.
1. Part 1: Image Data Collection and Scraping
Ā· Name: “Image Data Scraper”
Ā· What It Does: This part of the code collects image data from specified web pages.
Ā· Purpose: This script goes through a list of URLs, fetches the HTML content of each page, extracts image information (like image URLs, alt text, and other metadata), and saves this data to a CSV file.
Ā· Why It’s Important: This part is the first step in building a dataset of images from a website. It helps you gather information on all the images present on the provided web pages, which will be used later for SEO enhancement.
Ā· Key Steps:
- Sending HTTP Requests: Fetches the HTML content of each URL.
- Parsing HTML: Extracts <img> tags to find images on each page.
- Extracting Image Data: Retrieves image URLs, alt text (if available), and other metadata like width, height, etc.
- Saving to CSV: Saves this collected data in a structured format (CSV).
Explanation of the Code
Importing Libraries
- requests: This library allows us to send HTTP requests to websites and retrieve their content (e.g., HTML pages). Imagine this like visiting a webpage and getting its data for further processing.
- BeautifulSoup: This library is used to parse HTML and XML documents. It helps in finding and extracting specific parts of a webpage, such as images, text, etc., from the HTML code.
- pandas: This library is used for data manipulation and analysis, making it easy to work with tables (data frames) like those in Excel.
- urljoin: This function helps combine base URLs with relative URLs to create complete (absolute) URLs for images.
List of URLs to Scrape
- Purpose: This is a list of website pages that you want to scrape images from. Each URL represents a webpage from which images will be collected.
- Example: If you want to get images from a homepage and a services page, you add their URLs here.
Data Storage List
- Purpose: This empty list will be used to store the data (like image URLs, alt text, and metadata) that we extract from each webpage.
Function to Scrape Images
- Explanation: This function takes a URL as input and tries to fetch the webpage content using the requests library. If it fails (e.g., if the page doesnāt exist), an error will be raised.
- Explanation: Here, the HTML content of the webpage is parsed using BeautifulSoup. This makes it easier to navigate and extract specific parts of the webpage.
- Explanation: This line searches for all <img> tags in the HTML. These tags represent images on the webpage. If a webpage has five images, it will find all five.
Loop to Extract Image Information
- Explanation: This loop goes through each image found on the webpage. It extracts the src attribute, which holds the imageās URL.
- urljoin: If the image URL is not a complete URL (e.g., it starts with /images/example.jpg), this function converts it into a full URL (e.g., https://thatware.co/images/example.jpg).
- Explanation: The alt attribute provides a text description of the image, useful for accessibility and SEO. If no alt text is present, we set it to ‘No alt text available’.
- Explanation: This line captures all the attributes of the <img> tag (e.g., width, height) and stores them in a dictionary called metadata.
Storing the Extracted Data
- Explanation: This line creates a dictionary with the collected data (page URL, image URL, alt text, and metadata) and adds it to the data list.
Handling Request Errors
- Explanation: If there is an issue fetching a webpage (e.g., it doesnāt exist or thereās a network error), this except block prints an error message.
Loop to Scrape All URLs
- Explanation: This loop goes through each URL in the urls list and calls the scrape_images function to extract image data from each webpage.
Creating a DataFrame and Saving the Data
- Explanation:
- The data collected is converted into a DataFrame using pandas for easy viewing and manipulation.
- print(image_data_df.head()): This displays the first 5 rows of the data to check what was collected.
- image_data_df.to_csv(…): This saves the collected data to a CSV file named ‘image_data.csv’, which can be opened in Excel or used for further processing.
2. Part 2: Data Cleaning and Filtering
Ā· Name: “Image Data Cleaner”
Ā· What It Does: This part of the code cleans and filters the image data collected in the first part.
Ā· Purpose: It removes irrelevant or unnecessary entries from the dataset to ensure only useful data remains for further processing.
Ā· Why It’s Important: Cleaning the data helps remove noise and ensures that only relevant images are analyzed. It focuses on images from specific domains and removes entries like SVG images or invalid URLs.
Ā· Key Steps:
- Loading the Dataset: Reads the CSV file created by the first part.
- Removing Unwanted Entries: Excludes SVG images, data URIs, or invalid URLs.
- Domain Filtering: Keeps only images that belong to a specific domain (e.g., ‘thatware.co’).
- Saving the Cleaned Data: Writes the cleaned data to a new CSV file for further use.
Explanation of the Code:
Importing Required Library
- Explanation: The pandas library is used to work with data in table-like structures, similar to Excel sheets. It makes it easy to manipulate and analyze data.
- Example: If you have a table of image data, pandas lets you view, clean, and modify the data.
Step 1: Load the Input Dataset
- Explanation: This block loads the data that was previously scraped and saved into a CSV file named ‘image_data.csv’. If the file doesn’t exist, an error message is printed.
- Use Case: This ensures we have data to work with. If the file is missing, it prevents further processing, which would result in errors.
- Example: Imagine you have data about images saved in an Excel-like file (CSV). This code reads that data into a table (DataFrame) for further processing.
Step 2: Data Cleaning Function
- Explanation:
- Purpose: This function cleans the data by filtering out unwanted entries based on specific criteria.
- Step 2.1: The first two lines remove:
- Entries with SVG images (often vector images used in web design). SVG images may not be relevant for image SEO.
- URLs that don’t start with ‘http’ (e.g., data URIs or invalid URLs).
- Example: If an entry has an image URL like ‘data:image/svg+xml,…’, it will be removed because it’s not relevant for our analysis.
- Step 2.2: The next line filters out images that do not belong to the ‘thatware.co’ domain.
- Use Case: This is useful when you only want to focus on images hosted on a specific website.
- Example: If an image URL points to a third-party site like https://example.com/image.jpg, it will be excluded.
Step 3: Apply the Cleaning Function to the Loaded Data
- Explanation: This line applies the cleaning function to the loaded data. The result is a cleaned DataFrame that contains only relevant image data.
- Use Case: Ensures that the data used in further analysis is accurate and relevant.
Step 4: Save the Cleaned Data to a New CSV File
- Explanation: This saves the cleaned data to a new CSV file named ‘cleaned_image_output.csv’.
- Use Case: Storing cleaned data allows you to use it later without needing to repeat the cleaning process.
- Example: You now have a new file with only the cleaned image data that you can use for further analysis.
Step 5: Display the First 5 Rows of the Cleaned Dataset for Verification
- Explanation: This prints the first 5 rows of the cleaned data, making it easy to quickly verify that the data has been cleaned correctly.
- Example: If your cleaned data contains 100 entries, this will show the first 5 so you can check for issues.
Step 6: Provide a Completion Message
- Explanation: This is a simple print statement to let the user know that the data cleaning process has completed successfully.
- Use Case: It confirms that the cleaned data has been saved.
3. Part 3: Image Data Enhancement with Deep Learning
Ā· Name: “Deep Learning Image Enhancer”
Ā· What It Does: This part of the code enhances the image data by analyzing each image using a pre-trained deep learning model (ResNet50).
Ā· Purpose: The model recognizes and categorizes the content of each image, generates relevant tags, improves the alt text, and adds categorization and metadata enhancements.
Ā· Why It’s Important: This step uses artificial intelligence to analyze the images and generate SEO-friendly data, which can improve the visibility and accessibility of images on search engines.
Ā· Key Steps:
- Loading a Pre-trained Model: Uses ResNet50, a deep learning model trained to recognize objects in images.
- Fetching and Preprocessing Images: Downloads and prepares images for analysis by resizing and converting them to a format the model can understand.
- Generating Tags: Uses the model to generate descriptive tags for each image based on its content.
- Improving Alt Text: Creates new alt text using the generated tags.
- Categorization and Metadata Enhancement: Categorizes each image and creates additional metadata to improve its SEO value.
- Saving the Enhanced Data: Writes the enhanced data to a new CSV file.
Explanation of the Code:
Importing Required Libraries
- Purpose: These libraries are used to:
- requests: Fetch images from the internet using their URLs.
- PIL (Python Imaging Library): Process and transform images.
- BytesIO: Handle byte streams for image conversion.
- NumPy: Handle numerical operations and create arrays (data structures) for image processing.
- ResNet50: A pre-trained model that recognizes and classifies objects in images.
- pandas: Work with data in table format, making it easy to process and analyze.
Loading the Cleaned Dataset
- Purpose: Load the cleaned data from the previous step. This data contains image URLs and metadata from the initial data-cleaning step.
- Example: If the CSV file contains image data like URLs, alt text, etc., this line reads the file into a data table (DataFrame) for further processing.
Loading a Pre-trained Deep Learning Model
- Purpose: Load a deep learning model called ResNet50. This model has been trained on a huge dataset called ImageNet and can recognize thousands of different objects in images.
- Example: If you provide an image of a cat, the model can tell you it’s a cat and may even provide additional categories like “tabby cat” or “Siamese cat”.
Fetching and Preprocessing Images
- Purpose: This function fetches an image from a URL and prepares it for analysis by the deep learning model.
- Example: If the URL points to an image of a dog, this function fetches the image, resizes it, and prepares it for the model to analyze.
- Why: Resizing and converting images to a specific format is necessary for the model to work correctly.
Generating Tags for Images
- Purpose: This function uses the model to identify the contents of an image and generate relevant tags (descriptive words) for it.
- Example: If the image shows a “car”, the generated tags might include [“car”, “vehicle”, “sedan”].
Enhancing Image Data with New Information
- Purpose: This function adds additional useful information to each image using the deep learning model. It creates:
- Auto-generated tags for each image.
- Improved alt text using these tags.
- Category labels based on image content.
- Example: If the image is a “laptop”, it might generate tags like [“laptop”, “computer”], improve the alt text, and categorize it as “laptop”.
Saving the Enhanced Data
- Purpose: This part saves the enhanced data with new tags, improved alt text, and other metadata to a new CSV file.
- Example: If you previously had a simple “image URL” and “alt text”, now you also have tags, better descriptions, and categories.
Analysis of the Provided Output
1. Page URL and Image URL:
- These columns provide the webpage URL where each image is found and the direct URL to the image itself. This information is important for understanding where each image is located on the website and how it can be accessed or linked.
2. Original Alt Text:
- This column shows the alt text that was originally associated with each image. For images that had alt text, it is preserved. If there was no original alt text, subsequent steps would have created improved alt text.
3. Generated Tags:
- Provided: This column contains a list of tags generated by the Deep Learning model. For example, [‘book_jacket’, ‘comic_book’, ‘barbershop’] for the first image.
- Expected: The output should contain relevant tags generated by the model to aid in SEO.
- Analysis: This expectation is met. The generated tags are present and appear to describe the content of the images. While the relevance and accuracy of tags can be further fine-tuned, this is a solid start that improves SEO by associating images with descriptive keywords.
4. Improved Alt Text:
- Provided: This column contains improved alt text generated by the model. The improved text is descriptive and based on the generated tags (e.g., “Image showing book_jacket, comic_book, barbershop”).
- Expected: The output should include descriptive alt text that aligns with the image content and enhances accessibility.
- Analysis: This expectation is met. The alt text has been improved to provide a more descriptive and informative description of each image’s content. While the structure is templated (“Image showing …”), it still adds significant value compared to missing or generic alt text.
5. Category:
- Provided: This column categorizes each image based on the top generated tag (e.g., book_jacket).
- Expected: Images should be grouped into categories based on their content to facilitate better display and user navigation.
- Analysis: This requirement is met. Categorization based on the top tag provides a simple way to group images. More sophisticated categorization can be explored later, but this approach works for an initial implementation.
6. Enhanced Metadata:
- Provided: This field contains a dictionary with enriched metadata, including auto_tags, improved_alt_text, and category.
- Expected: The metadata should be SEO-rich, containing information that improves image search rankings.
- Analysis: This requirement is met. The enhanced metadata combines tags, improved alt text, and category data to provide comprehensive information that can improve image searchability and SEO performance.
Conclusion of the Output:
- Comprehensive Data: The output includes all expected fields, such as auto-generated tags, improved alt text, categorization, and enhanced metadata.
- Alignment with Expected Results: The output aligns well with your expectations, as it improves the SEO potential of images by providing descriptive information, meaningful tags, and categorization.
- Improved SEO Potential: The generated tags, improved alt text, and enhanced metadata collectively provide significant potential for improving the SEO performance of the images.
Explanation of Each Part of the Output
1. Page URL:
- What it is: This column contains the URL of the webpage where the image is used.
- Use case: This helps identify which specific webpage contains the image, providing context for where the image appears. For example, if a business owner wants to understand where images are displayed on their website, this column will give that information.
- How it can be used: Knowing which page an image belongs to can help in analyzing how well the image content aligns with the page’s purpose and SEO goals. If a webpage is underperforming, you can optimize the images on that page to increase engagement.
2. Image URL:
- What it is: This column shows the direct URL of the image itself.
- Use case: This provides a way to directly access or view the image. It can also be used for tasks like verifying that the image loads correctly or optimizing image size and format for faster loading.
- How it can be used: You can inspect each image to ensure it is relevant to the page content, properly formatted, and displayed without issues. This can improve user experience and, ultimately, website rankings.
3. Generated Tags:
- What it is: These are tags generated by the Deep Learning model that describe the content of the image. For example, [‘book_jacket’, ‘comic_book’, ‘barbershop’] might describe the objects or concepts that the model recognized in the image.
- Use case: These tags can be used to better understand the image content and ensure it aligns with the page’s topic or the website’s overall theme.
- How it can be used: The tags can be added as keywords or metadata to make the image more discoverable in search engines, improving SEO and driving more traffic to the website.
4. Improved Alt Text:
- What it is: This is alt text generated based on the generated tags. For example, “Image showing book_jacket, comic_book, barbershop.”
- Use case: The improved alt text provides a more descriptive and relevant description of the image content, enhancing accessibility and helping search engines better index the image.
- How it can be used: If the original alt text was missing or inadequate, the improved alt text can be used to replace or supplement it. This improves the website’s accessibility and search visibility.
5. Enhanced Metadata:
- What it is: This field contains a dictionary of metadata, including auto_tags, improved_alt_text, and category. This additional data provides detailed information about the image that can be used to enhance its discoverability and ranking on search engines.
- Use case: Enhanced metadata provides search engines with rich information about each image, increasing the chances of the image appearing in image search results.
- How it can be used: Website owners can use this enhanced metadata to update image descriptions, add relevant tags, and create detailed image alt text. This can improve image ranking and drive more organic traffic.
What Steps Should a Website Owner Take Next?
1. Review and Update Image Descriptions:
- If the original alt text is missing or not descriptive, replace it with the improved alt text.
- Ensure that the alt text is accurate, descriptive, and relevant to the content of the image.
2. Incorporate Auto-Generated Tags:
- Use the tags generated by the model to add relevant keywords to your image metadata. This can make your images more discoverable through image searches and improve the overall SEO of your website.
3. Optimize Page Content:
- Ensure that the images are relevant to the content of the pages they are used on. If necessary, update the page content or swap out images to improve the overall user experience.
4. Use Categories to Group Similar Images:
- Leverage the generated categories to create thematic sections on your website. For example, if you have images categorized under book_jacket, you can create a section that showcases content related to books or publishing.
5. Monitor Performance:
- Track the impact of these changes on your website’s traffic, engagement, and image ranking in search engines. This will help you gauge the effectiveness of the changes and make further adjustments as needed.
Summary for Non-Technical Users
- This output helps you better describe, categorize, and optimize the images on your website.
- By improving image descriptions and metadata, your website becomes more accessible and easier for search engines to understand. This increases the likelihood of your images appearing in search results, which can drive more traffic to your site.
- The steps you take based on this output can enhance user experience, improve SEO, and ultimately contribute to growing your business online.
Thatware | Founder & CEO
Tuhin is recognized across the globe for his vision to revolutionize digital transformation industry with the help of cutting-edge technology. He won bronze for India at the Stevie Awards USA as well as winning the India Business Awards, India Technology Award, Top 100 influential tech leaders from Analytics Insights, Clutch Global Front runner in digital marketing, founder of the fastest growing company in Asia by The CEO Magazine and is a TEDx speaker.