SUPERCHARGE YOUR ONLINE VISIBILITY! CONTACT US AND LET’S ACHIEVE EXCELLENCE TOGETHER!
This project demonstrates the application of the BLEU (Bilingual Evaluation Understudy) score to evaluate the quality of machine translation. The evaluation is performed on English-to-German content using standard benchmark data from the WMT14 dataset.
Machine-translated outputs are generated using a state-of-the-art model, and compared against human-provided translations. The BLEU score serves as a robust metric to measure how closely the machine-generated text matches the reference, based on n-gram overlap.
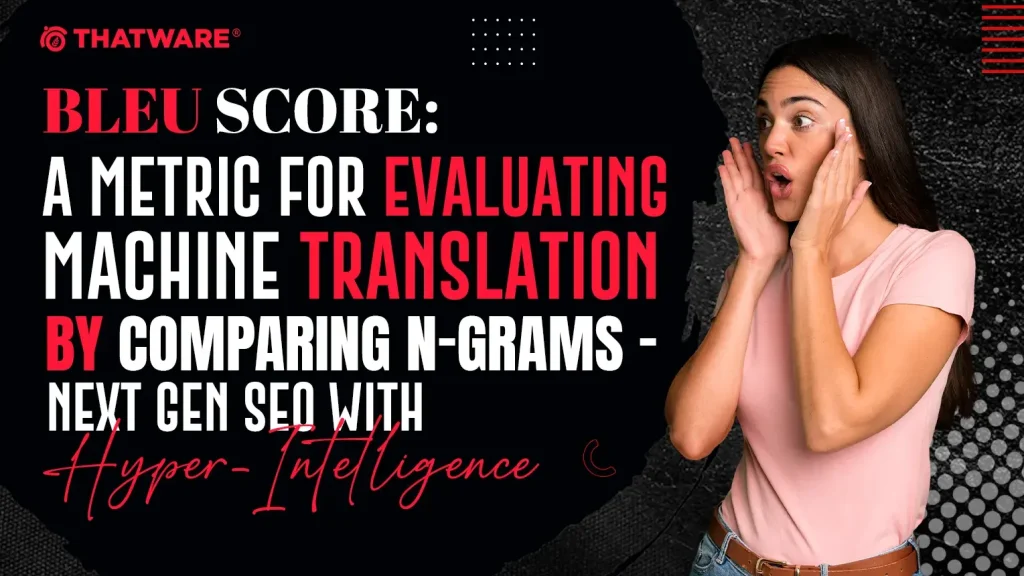
The goal is to provide a reliable, scalable approach to quantify translation quality—especially relevant for multilingual SEO content workflows where consistency and semantic accuracy are critical for global reach.
Purpose of the Project
The purpose of this project is to showcase how the BLEU score can be used to objectively evaluate the quality of machine-translated content. In multilingual SEO and global content strategies, ensuring that translations preserve meaning and intent is essential for both user experience and search performance.
By comparing machine-generated translations to professionally translated references, this project demonstrates how BLEU provides a measurable and repeatable way to assess translation accuracy. The approach helps identify the effectiveness of translation models and supports data-driven decisions for content localization and quality control.
Understanding BLEU Score
What is BLEU Score
BLEU (Bilingual Evaluation Understudy) is a widely used metric that helps evaluate the quality of text generated by machine translation systems. In simple terms, it tells us how close a machine-translated sentence is to a human translation.
Think of it as comparing two versions of the same sentence — one written by a professional translator and the other by a machine. BLEU gives us a score between 0 and 1:
- A score closer to 1 means the machine translation is very similar to the human version.
- A score near 0 means the translations differ significantly.
How BLEU Works
BLEU works by breaking down sentences into small pieces called n-grams (short word groups) and comparing these between the machine and human translations.
For example:
- 1-gram looks at individual words (e.g., “cat”, “runs”).
- 2-gram looks at word pairs (e.g., “the cat”, “cat runs”).
- 3-gram and 4-gram go further, checking for even longer phrases.
The more matching n-grams found between the machine and human translations, the better the BLEU score.
What Does BLEU Measure?
BLEU evaluates translation quality using n-gram overlap, which refers to the occurrence of contiguous sequences of words — such as unigrams (1-word), bigrams (2-word), trigrams (3-word), and so on — between the machine translation (candidate) and the reference translation(s). The more n-grams that match, the better the score.
However, BLEU is not just about counting words — it also considers:
- Word order and phrase structure
- Sentence completeness
- Conciseness and redundancy
To discourage overly short translations that artificially inflate match counts, BLEU introduces a brevity penalty. This ensures that the machine translation captures the full meaning of the source without being overly concise.
Key Concepts in BLEU:
- N-gram Precision: Measures how many n-grams in the machine-generated output appear in the reference translation. This reflects surface-level fluency and accuracy.
- Modified Precision: BLEU avoids inflating scores by clipping the number of times a matching n-gram can be counted — it only considers the maximum count of that n-gram in any reference.
- Brevity Penalty (BP): Penalizes translations that are too short compared to the reference, addressing issues where the output might be fluent but incomplete.
- Smoothing Techniques: When evaluating longer n-grams (e.g., 4-grams), it’s common to get zero matches. BLEU applies smoothing methods to prevent a single zero from collapsing the entire score.
Why BLEU Matters for SEO
In multilingual SEO, ensuring accurate and high-quality translations is critical. Poor translations can misrepresent the message, hurt brand perception, or even affect rankings due to reduced user engagement.
BLEU offers a standardized, automatic way to:
- Measure the quality of translated web content
- Compare different machine translation models
- Ensure consistency across large-scale translated content
This helps teams maintain quality without manually reviewing every translated sentence — saving time, effort, and improving global SEO performance.
How does BLEU help with search engines and SEO?
BLEU helps ensure that automated translations maintain high linguistic quality and semantic accuracy. Search engines evaluate not just keywords but also fluency, context, and overall content quality. Poorly translated pages may be flagged as low-quality or duplicate content, harming rankings. By applying BLEU, we can systematically identify and improve low-quality translations before they impact SEO performance.
Why is BLEU important for evaluating translation models?
BLEU offers a standardized, objective way to measure how closely machine-generated content matches human-quality translations. Rather than relying on subjective opinions, we use BLEU scores to benchmark and compare models. This helps in selecting the best-performing model and ensuring the consistency of translated SEO content across multiple languages.
What are the practical benefits of this project for a website owner?
- Improved Global Visibility: Ensures translated content ranks well on international search engines.
- Content Accuracy at Scale: Allows automated checks on thousands of translated pages without human review.
- Model Validation: Verifies which machine translation model is best for a specific domain or language pair.
- Reduced Costs: Minimizes the need for full manual translation by identifying which areas need intervention.
- Quality Assurance: Builds confidence that automated translations meet a measurable quality standard.
Libraries and Tools Used
PyTorch (torch)
Purpose:
PyTorch is an open-source machine learning library developed by Facebook AI. It serves as the backend computation engine for running deep learning models, particularly those used in natural language processing.
How it’s used:
In this project, PyTorch is the framework that powers the inference of the pre-trained neural machine translation models. When a sentence is translated using a model, PyTorch handles the internal computations across multiple layers of the model and supports GPU acceleration to speed up the process.
Why it matters:
Without PyTorch, we would need to implement and train complex neural models from scratch. Its modularity and performance make it ideal for running state-of-the-art models efficiently at scale.
Hugging Face Datasets (datasets.load_dataset)
Purpose:
This module provides seamless access to thousands of standard NLP datasets from within Python. It supports automatic downloading, preprocessing, and version management.
How it’s used:
We use it to load the WMT19 English-German dataset, one of the most established benchmarks for evaluating machine translation systems. The dataset is accessed in a structured format, where each entry contains both the source English sentence and its human-translated German equivalent.
Why it matters:
This saves significant time in manual data collection, and ensures the dataset is consistent, trustworthy, and widely recognized in the industry for fair evaluation.
Hugging Face Transformers (transformers)
Purpose:
This is the primary library for working with large-scale pre-trained transformer-based models such as BERT, T5, and in our case, machine translation models like facebook/wmt19-en-de.
Components used:
- AutoModelForSeq2SeqLM: Automatically selects and loads a suitable sequence-to-sequence model based on the name provided. These models are specialized for tasks like translation and summarization.
- AutoTokenizer: Prepares raw input text by converting it into a format (tokens) that the model can understand, and vice versa.
How it’s used:
Once the dataset is prepared, we use these tools to send sentences into the model and retrieve translated outputs. This library abstracts away the complexity of deep learning architectures, making it possible to use high-performing models without having to build or train them.
Why it matters:
The Hugging Face ecosystem drastically reduces development time and enables rapid experimentation with different models, which is critical when trying to optimize translation quality for real-world applications like multilingual SEO.
NLTK BLEU (nltk.translate.bleu_score)
Purpose:
The BLEU (Bilingual Evaluation Understudy) score is the most widely used metric to evaluate the quality of machine-translated text. The NLTK library provides a robust and customizable implementation of BLEU.
How it’s used:
- corpus_bleu: Measures how closely the machine-generated translations match the human reference translations.
- SmoothingFunction: Helps stabilize BLEU score calculations when dealing with shorter texts or less common word overlaps, which are common in real-world web content.
Why it matters:
BLEU provides a numeric value that helps quantify how “accurate” or “natural” a machine translation is. This allows us to benchmark models, track performance improvements, and make informed decisions about which models are suitable for production use.
Regular Expressions (re)
Purpose:
This standard Python module is used for text normalization and cleanup, which is a critical preprocessing step before running any machine learning or evaluation operations.
How it’s used:
We use regular expressions to:
- Replace inconsistent quotation marks and apostrophes
- Remove non-breaking spaces or redundant whitespace
- Convert text to lowercase for case-insensitive evaluation
Why it matters:
Unclean or inconsistently formatted text can skew BLEU score calculations by introducing mismatched tokens. Proper normalization ensures that both reference translations and generated outputs are compared fairly, leading to more accurate and meaningful scores.
Function clean_text(text)
This function is responsible for standardizing and cleaning text before it’s passed into a model or evaluation metric like BLEU.
This function helps eliminate noisy inconsistencies in the data like spacing, casing, or punctuation that do not affect translation meaning but can significantly affect BLEU scores. It ensures that comparisons between machine-translated output and reference translations are fair, consistent, and aligned with real-world SEO text processing needs.
Here’s what each line of code is doing:
text = text.replace(“\u202f”, ” “).replace(“\xa0″, ” “) This line replaces two special types of invisible or non-breaking spaces with regular space characters.
text = re.sub(r”\s+”, ” “, text.strip()) First, it removes any extra spaces at the beginning and end of the sentence (strip()). Then it condenses multiple spaces (like double or triple spaces) into a single space using a regular expression.
text = re.sub(r”[“”‘’\”‘]”, “\””, text) Replaces all types of curly/smart quotes (like “ or ” or ‘) and even regular single or double quotes (” and ‘) with a standard double quote character. Quotes are handled differently in various datasets and languages. This ensures uniformity across source, reference, and predicted text — avoiding false mismatches during comparison.
return text.lower() Converts the entire string to lowercase.
Dataset Overview: WMT19 (de-en)
In this project, the WMT19 dataset from Hugging Face is used as the benchmark dataset for evaluating machine translation quality between English (en) and German (de).
Source
Hugging Face Dataset Link: https://huggingface.co/datasets/wmt/wmt19
What is WMT?
WMT (Workshop on Machine Translation) is one of the most prominent annual events in the field of machine translation. It brings together researchers and practitioners to evaluate and benchmark translation systems across multiple language pairs.
Why WMT Matters
- Trusted Benchmark: WMT datasets are curated and released as part of international shared tasks for translation quality assessment.
- Human References: The translations in WMT datasets are created or verified by professional translators, ensuring high linguistic quality.
- Widespread Usage: Used by companies like Google, Facebook, Microsoft, and many academic institutions to train and test machine translation models.
Explanation
What it Contains:
Each entry in the dataset is a dictionary under the translation key, where:
- “de” refers to the German source sentence.
- “en” refers to the English reference translation.
This project uses the “validation” split, which includes approximately 3,000 examples. It is commonly used for evaluating model performance without training on the same data.
Why it is Used:
WMT datasets are widely adopted benchmarks in machine translation research. WMT19 specifically contains high-quality human-translated sentence pairs, making it ideal for evaluating translation systems with metrics like BLEU.
Benefits in This Project
- Ensures real-world language quality with authentic sentences.
- Enables objective evaluation using BLEU scores by comparing model-generated translations to expert human references.
- Standardized across research and industry, providing credibility to the results.
Explanation
source_texts = […]
This line collects all the German sentences (“de”) from the dataset as source inputs.
Each sentence is passed through the clean_text() function to ensure normalization (lowercasing, consistent spacing, and quote formatting).
The result is a list of clean German strings, ready for input to the translation model.
reference_translations = […]
This line prepares the English reference translations (“en”) for BLEU evaluation.
Each English sentence is:
- Cleaned using the same clean_text() function.
- Tokenized using .split() to produce a list of words.
Wrapped in an additional outer list ([ … ]) to match the expected BLEU input format, which allows multiple reference translations per sentence.
Explanation:
device = torch.device(“cuda” if torch.cuda.is_available() else “cpu”)
- This line checks whether a CUDA-compatible GPU is available.
- If a GPU is available, it sets device to “cuda”, which means the model and data will be moved to the GPU for faster computation.
- If no GPU is available, it defaults to using the CPU (“cpu”).
- This is important for making sure the model can utilize hardware acceleration if possible, speeding up inference.
model_name = “facebook/wmt19-en-de”
- The variable model_name specifies the pre-trained model that will be used for the translation task.
- Here, it points to the model “facebook/wmt19-en-de”, which is a large translation model for German to English translations, trained on the WMT19 dataset.
model = AutoModelForSeq2SeqLM.from_pretrained(model_name).to(device)
- This line loads the pre-trained sequence-to-sequence model (AutoModelForSeq2SeqLM) using the specified model_name.
- The .to(device) part ensures the model is moved to the appropriate device (GPU or CPU), as determined in the first step.
- This model will be used to generate translations for the input sentences.
tokenizer = AutoTokenizer.from_pretrained(model_name)
- This line loads the corresponding tokenizer for the model.
- The tokenizer is responsible for converting the input text into tokens that the model can process and then converting the model’s output tokens back into human-readable text.
Model Explanation
The model used in this project is facebook/wmt19-en-de, a high-performance transformer-based translation model developed by Facebook AI Research (FAIR). It was trained as part of the WMT19 shared task, focusing on the English <-> German language pair.
Key Features of the Model
· Pre-trained on Massive Data
- This model is trained on extensive parallel corpora sourced from the WMT19 dataset, including millions of sentence pairs in English and German. This allows it to capture a wide range of vocabulary, grammar patterns, and linguistic nuances.
· Transformer Architecture
- The model is based on the Transformer architecture, the industry standard for neural machine translation. It uses self-attention mechanisms to understand the context of every word in a sentence and generate fluent translations.
· Sequence-to-Sequence (Seq2Seq) Translation
- It implements the seq2seq framework, where an encoder processes the input (English sentence) and a decoder generates the corresponding translation (German sentence). This allows the model to handle varying sentence lengths and structures effectively.
· Deep Model with Large Capacity
- The model features deep encoder and decoder stacks with many layers, enabling it to learn complex language representations. This contributes to its ability to translate idiomatic expressions, domain-specific vocabulary, and intricate grammar patterns.
· WMT19 Winner
- The facebook/wmt19-en-de model was part of Facebook’s winning system in the WMT19 news translation task. It was ranked among the top by human evaluators, often outperforming other open-source systems.
Why This Model Was Chosen
- Top-tier BLEU Scores: It consistently produces high BLEU scores, making it ideal for measuring translation quality in this project.
- Battle-tested in Competitions: Its performance in WMT19 evaluation benchmarks ensures reliability and robustness across multiple domains.
- Pre-trained and Ready-to-Use: Available through HuggingFace Transformers, this model can be easily integrated into workflows without expensive training.
- Well-optimized for German <-> English: It is fine-tuned on domain-rich and well-curated data, making it more accurate than general-purpose multilingual models.
Function: translate(texts, model, tokenizer, batch_size=16)
This function handles the translation of a list of English sentences into German (or any target language), using a pre-trained model and tokenizer:
translations = [] for i in range(0, len(texts), batch_size): batch = texts[i:i+batch_size]
Divides the input text into smaller chunks (batches). Allows efficient processing, especially for large datasets and when running on GPU.
inputs = tokenizer(batch, return_tensors=”pt”, padding=True, truncation=True).to(device)
Tokenizes and prepares the batch for the model. Converts raw text into numerical input the model can understand. It also ensures proper padding and truncation to handle different sentence lengths.
with torch.no_grad(): outputs = model.generate(**inputs, max_length=512)
Generates translations using the model in inference mode. torch.no_grad() disables gradient calculation to save memory and computation, which is ideal for evaluation.
decoded = [tokenizer.decode(t, skip_special_tokens=True).lower().split() for t in outputs]
Decodes generated token IDs back to readable text. Converts model output into human-readable translations. The .lower().split() ensures consistency and prepares the data for BLEU evaluation.
translations.extend(decoded)
Collects all translated batches into a single list. Allows the function to return complete results for the full dataset.
It is optimized for evaluation purposes and ensures compatibility with BLEU scoring methods.
This code invokes the translate function to generate translations. The source_texts (which are the German sentences from the dataset) are passed to the function, and the model generates English translations.
Smoothing Function:
The SmoothingFunction() is used to handle cases where the n-grams in the candidate translations don’t appear in the reference translations. This helps to avoid a BLEU score of 0 when rare or unseen n-grams are involved. We use method1, which applies a basic smoothing technique.
Calculate BLEU Score:
The corpus_bleu function calculates the BLEU score by comparing the candidate translations (candidates) with the reference translations (reference_translations).
The smoothing function is passed to handle edge cases, ensuring a more stable score.
The final BLEU score, which indicates the quality of the generated translations, is stored in bleu_score.
Result Analysis and Discussion
BLEU (Bilingual Evaluation Understudy) is a standardized metric used across the translation industry to assess the quality of machine-generated text. It provides a numerical value that reflects how closely a translated sentence aligns with one or more high-quality human references. While it’s widely used in research and development, it also has strong relevance in real-world business and product environments.
In practical terms, the BLEU score helps identify whether a machine translation is fluent, accurate, and contextually appropriate—without requiring manual evaluation for every sentence.
What Is Considered a Good BLEU Score?
BLEU scores range from 0 to 1, though they are typically represented as decimals (e.g., 0.34). A score of 1 would indicate a perfect overlap with the reference, though this is rare in practice, especially when there’s only one reference sentence. Here’s how BLEU is commonly interpreted in real-world applications:
- Scores below 0.20 usually reflect poor translation quality—phrases may be broken, missing, or grammatically incorrect.
- Scores in the 0.20–0.30 range indicate basic fluency. The translations are often understandable but may still lack naturalness or miss finer nuances.
- Scores between 0.30 and 0.50 are generally considered strong and practical for production use. Translations in this range tend to be accurate, fluent, and easy to post-edit.
- Scores above 0.50 reflect very high-quality output, nearing human translation. This level is ideal but often requires more advanced models or multiple reference translations.
- Scores beyond 0.70 are rarely seen unless the test cases closely resemble training data or when multiple references are available.
Interpreting BLEU in Production Contexts
It’s important to note that BLEU scores do not fully capture meaning or semantic correctness. A translation can be perfectly valid with different wording and still receive a moderate BLEU score. Therefore, in client-facing systems or content pipelines, BLEU should be used as a diagnostic signal—one that indicates whether the model is performing consistently across batches or languages.
In many real-world scenarios (such as website localization, knowledge base translation, or automated customer support content), a BLEU score in the mid-to-high 30s is already a sign of a capable translation model—especially when dealing with domain-specific or informal language.
How does this project benefit business and SEO?
This project helps improve the quality of translations on your website, which can significantly benefit your global SEO strategy. Here’s how:
· Improved User Engagement: Accurate and fluent translations increase user satisfaction, which keeps visitors on your site longer. Search engines favor websites with lower bounce rates, meaning better translation can directly boost your rankings.
· Localized Content for Different Markets: If you target international audiences, machine translation helps you scale your content across languages. By providing high-quality translations, you can ensure that users from different regions see relevant content tailored to their language and culture.
· Efficiency at Scale: Instead of relying on human translators for every piece of content, machine translation lets you quickly and cost-effectively generate content in multiple languages, saving both time and money while maintaining quality.
What should You do after getting the BLEU score for translations?
Once you receive the BLEU score, it serves as a quality check for your translations. Here’s what you can do next:
· If the BLEU score is high: This means your translations are likely accurate and fluent. You can move forward with publishing or using the translated content for marketing or SEO efforts.
· If the BLEU score is lower: It may indicate that the translations need improvement. This might mean adjusting the translation model, manually reviewing some translations, or re-training the model with more specific data related to your business or target audience.
Essentially, the BLEU score is your tool to ensure that the content you’re offering to global users is both correct and engaging. Depending on the score, you can either move ahead with confidence or make necessary tweaks to improve quality.
What does a high or low BLEU score mean for my content?
A high BLEU score suggests that the translation is accurate and fluent, closely matching human-generated translations. This means your translated content is likely to resonate well with your target audience and maintain high-quality SEO practices.
- A good BLEU score typically falls in the range of 0.30–0.50. It indicates that the translation is solid and can be confidently used for publishing.
- A lower BLEU score, under 0.30, may indicate that the translation could be missing nuances or that certain phrases or words are not being translated correctly. In such cases, some manual adjustments or fine-tuning of the translation model might be required to improve the results.
How does BLEU affect the global reach of your marketing campaigns?
Machine translations with a high BLEU score can significantly impact the effectiveness of your global marketing campaigns. Accurate translations ensure that your content is properly understood across different regions and cultures, boosting engagement and performance in local markets. Here’s how:
· Consistency Across Markets: You’ll ensure that your messaging stays consistent, no matter the language. A high BLEU score means that your translated content will convey the same tone, style, and message across all languages.
· Better Engagement: When customers in different countries see content in their own language, they are more likely to engage with it, increasing conversion rates and reducing bounce rates. This ultimately leads to better SEO performance as search engines favor content that keeps visitors engaged.
Final Thoughts
In summary, the use of machine translation models, particularly those leveraging the latest advancements in AI such as transformer-based architectures, offers significant advantages for businesses looking to expand their digital presence globally. These models provide a reliable, scalable, and cost-effective way to translate content across multiple languages, helping to engage a broader audience and improve SEO outcomes.
The BLEU score serves as a crucial tool in assessing the quality of the translations. While it’s not a perfect measure, it provides a consistent and quantifiable way to evaluate how well the machine translation matches human translations. A higher BLEU score typically correlates with more fluent, accurate translations, but it’s important to remember that even a lower score doesn’t necessarily indicate a bad result. It simply suggests areas for improvement, especially for content that requires more contextual understanding or industry-specific terminology.
As businesses grow and expand into new markets, regularly assessing and fine-tuning your translation models will help ensure that your multilingual content remains high-quality and effective in engaging users. Continuous monitoring, combined with human expertise when necessary, ensures that machine translations align with your brand’s voice and resonate with local audiences.
The machine translation project presented here highlights the potential of AI in revolutionizing content localization and SEO. By adopting this approach, businesses can streamline their content processes, provide valuable localized experiences, and ultimately drive better results in international markets.
Incorporating machine translation into your SEO strategy, paired with the right evaluation metrics like BLEU, will help you reach global customers more effectively while ensuring that your content maintains high standards of quality and relevance.
Thatware | Founder & CEO
Tuhin is recognized across the globe for his vision to revolutionize digital transformation industry with the help of cutting-edge technology. He won bronze for India at the Stevie Awards USA as well as winning the India Business Awards, India Technology Award, Top 100 influential tech leaders from Analytics Insights, Clutch Global Front runner in digital marketing, founder of the fastest growing company in Asia by The CEO Magazine and is a TEDx speaker and BrightonSEO speaker.