A wise man once said, “Knowledge of Languages is the Doorway to Wisdom”.
It’s actually a 13th-century quote. However, that does not mean that it is any less true as of now. In fact, it is perhaps one of the most important subjects of study in the 21st century since the applications of this concept have grown tremendously. Today we know it as Natural Language Processing, and we know for sure Google takes it very seriously.
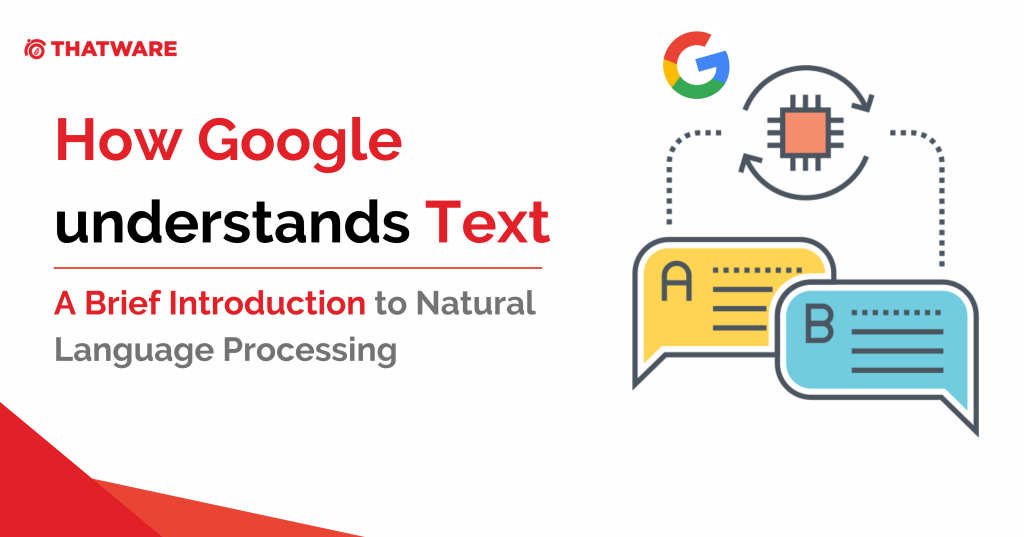
In this article, I will be explaining the basic concepts of NLP namely, POS Tagging and Dependency Parsing.
Parts of Speech Tagging
Since our school days when we learned English for the first time, we have come across different forms of words, namely nouns, adjectives, prepositions, verbs, determiners and other kinds of subjects and modifiers.
Such identifiers were created in order to understand the relation of each word in a sentence with another. The concept is so basic, that defining the purpose of each word in a sentence became the foundation for Natural Language Processing.
That is where Part of Speech Tagging comes from. POS Tags are labels assigned to different words in a sentence to understand the context of the “parts of speech”.
There are mainly two types of Tags:
- Universal POS Tags
These tags include NOUN(Common Noun), ADJ(Adjective), ADV(Adverb).
You can learn more about them in this document.
- Detailed POS Tags
These include secondary tags NNS for common plural nouns and NN for the singular common noun compared to NOUN for common nouns in English.
Now that we know about POS Tags, we can run a program that returns such relations between each word in a sentence.
Let us take an example: “The quick brown fox jumping over the lazy dog”
Run the Following Code in Python:
Terminal Commands:
(base) C:\Users\USER>pip install spacy
(base) C:\Users\USER>conda install -c conda-forge spacy-model-en_core_web_sm
(base) C:\Users\USER>python -m spacy download en_core_web_sm
(base) C:\Users\USER>pip install networkx
Python Code:
>>> import spacy
>>> nlp=spacy.load(‘en_core_web_sm’)
>>> import en_core_web_sm
>>> nlp = en_core_web_sm.load()
>>> from spacy import displacy
>>> displacy.render(nlp(text),jupyter=True)
<IPython.core.display.HTML object>
>>> sentence = “The quick brown fox jumping over the lazy dog”
>>> doc = nlp(sentence)
>>> print(f”{‘Node (from)–>’:<15} {‘Relation’:^10} {‘–>Node (to)’:>15}\n”)
>>> for token in doc:
… print(“{:<15} {:^10} {:>15}”.format(str(token.head.text), str(token.dep_),
str(token.text)))
…
Output
Node (from)–> Relation –>Node (to)
fox det The
fox amod quick
fox amod brown
fox ROOT fox
fox acl jumping
jumping prep over
dog det the
dog amod lazy
over pobj dog
Dependency Parsing
It involves making sense of the grammatical structure of the sentence based on the
dependencies between the words of a sentence.
For example in the above sentence “The quick brown fox jumping over the lazy dog”,
“brown” acts as an adjective that modifies the noun “fox”. Hence there is a dependency on the word “fox” to the word “brown”. This dependency is defined by the “amod” tag known as the adjective modifier.
A thing to note is that dependency always occurs between two words in a sentence. Let’s now write a program that can return such dependencies between different words in the following sentence.
“It took me more than two hours to translate a few pages of English.”
Terminal Commands:
(base) C:\Users\USER>pip install spacy
(base) C:\Users\USER>conda install -c conda-forge spacy-model-en_core_web_sm
(base) C:\Users\USER>python -m spacy download en_core_web_sm
(base) C:\Users\USER>pip install networkx
Python Code:
>>> import spacy
>>> nlp=spacy.load(‘en_core_web_sm’)
>>> import en_core_web_sm
>>> nlp = en_core_web_sm.load()
>>> text=’It took me more than two hours to translate a few pages of English.’
>>> for token in nlp(text):
print(token.text,’=>’,token.dep_,’=>’,token.head.text)
…
//First column is the text
//Second column is the Tag
//Third column is the head term
Output
It => nsubj => took
took => ROOT => took
me => dobj => took
more => amod => two
than => quantmod => two
two => nummod => hours
hours => dobj => took
to => aux => translate
translate => xcomp => took
a => quantmod => few
few => amod => pages
pages => dobj => translate
of => prep => pages
English => pobj => of
. => punct => took
Finding Shortest Dependency Path With Spacy
Semantic dependency parsing has been often used as a way to obtain information between words(entities) that are related but are far in sentence distance.
The Shortest Dependency Path or SDP contains all the information that is just enough to define the relationship between two words in a sentence.
Now let’s find the SDP with the help of a Python Program.
Terminal Commands:
(base) C:\Users\USER>pip install spacy
(base) C:\Users\USER>conda install -c conda-forge spacy-model-en_core_web_sm
(base) C:\Users\USER>python -m spacy download en_core_web_sm
(base) C:\Users\USER>pip install networkx
Python Code:
>>> import spacy
>>> import networkx as nx
>>> nlp = spacy.load(“en_core_web_sm”)
>>> doc = nlp(u’Convulsions that occur after DTaP are caused by a fever.’)
>>> print(‘sentence:’.format(doc))
sentence:
>>> edges = []
>>> for token in doc:
… for child in token.children:
… edges.append((‘{0}’.format(token.lower_),
… ‘{0}’.format(child.lower_)))
…
>>> graph = nx.Graph(edges)
>>> entity1 = ‘Convulsions’.lower()
>>> entity2 = ‘fever’
>>> print(nx.shortest_path_length(graph, source=entity1, target=entity2))
3
>>> print(nx.shortest_path(graph, source=entity1, target=entity2))
[‘convulsions’, ’caused’, ‘by’, ‘fever’]
As you can see, the shortest path length function returns 3 as the shortest number of jumps of hops made to establish the relationship.
The shortest path function returns the exact words which are part of the SDP.
Conclusion
These are some elements that help gives an elementary idea of how an AI makes relationships between different words and hence is an introduction to the vast subject of NLP. Obviously, Google AI is much more complex than two or three Python scripts, however, our purpose was to share a brief idea of how a machine can create a semantic relationship between words in a text.